mirror of
https://github.com/qurator-spk/eynollah.git
synced 2025-07-05 17:09:57 +02:00
Compare commits
No commits in common. "main" and "v0.3.1" have entirely different histories.
49 changed files with 5385 additions and 9481 deletions
|
@ -1,6 +0,0 @@
|
|||
tests
|
||||
dist
|
||||
build
|
||||
env*
|
||||
*.egg-info
|
||||
models_eynollah*
|
44
.github/workflows/build-docker.yml
vendored
44
.github/workflows/build-docker.yml
vendored
|
@ -1,44 +0,0 @@
|
|||
name: CD
|
||||
|
||||
on:
|
||||
push:
|
||||
branches: [ "master" ]
|
||||
workflow_dispatch: # run manually
|
||||
|
||||
jobs:
|
||||
|
||||
build:
|
||||
runs-on: ubuntu-latest
|
||||
permissions:
|
||||
packages: write
|
||||
contents: read
|
||||
steps:
|
||||
- name: Checkout
|
||||
uses: actions/checkout@v4
|
||||
with:
|
||||
# we need tags for docker version tagging
|
||||
fetch-tags: true
|
||||
fetch-depth: 0
|
||||
- # Activate cache export feature to reduce build time of images
|
||||
name: Set up Docker Buildx
|
||||
uses: docker/setup-buildx-action@v3
|
||||
- name: Login to GitHub Container Registry
|
||||
uses: docker/login-action@v3
|
||||
with:
|
||||
registry: ghcr.io
|
||||
username: ${{ github.actor }}
|
||||
password: ${{ secrets.GITHUB_TOKEN }}
|
||||
- name: Log in to Docker Hub
|
||||
uses: docker/login-action@v3
|
||||
with:
|
||||
username: ${{ secrets.DOCKERIO_USERNAME }}
|
||||
password: ${{ secrets.DOCKERIO_PASSWORD }}
|
||||
- name: Build the Docker image
|
||||
# build both tags at the same time
|
||||
run: make docker DOCKER_TAG="docker.io/ocrd/eynollah -t ghcr.io/qurator-spk/eynollah"
|
||||
- name: Test the Docker image
|
||||
run: docker run --rm ocrd/eynollah ocrd-eynollah-segment -h
|
||||
- name: Push to Dockerhub
|
||||
run: docker push docker.io/ocrd/eynollah
|
||||
- name: Push to Github Container Registry
|
||||
run: docker push ghcr.io/qurator-spk/eynollah
|
24
.github/workflows/pypi.yml
vendored
24
.github/workflows/pypi.yml
vendored
|
@ -1,24 +0,0 @@
|
|||
name: PyPI CD
|
||||
|
||||
on:
|
||||
release:
|
||||
types: [published]
|
||||
workflow_dispatch:
|
||||
|
||||
jobs:
|
||||
pypi-publish:
|
||||
name: upload release to PyPI
|
||||
runs-on: ubuntu-latest
|
||||
permissions:
|
||||
# IMPORTANT: this permission is mandatory for Trusted Publishing
|
||||
id-token: write
|
||||
steps:
|
||||
- uses: actions/checkout@v4
|
||||
- name: Set up Python
|
||||
uses: actions/setup-python@v5
|
||||
- name: Build package
|
||||
run: make build
|
||||
- name: Publish package distributions to PyPI
|
||||
uses: pypa/gh-action-pypi-publish@release/v1
|
||||
with:
|
||||
verbose: true
|
48
.github/workflows/test-eynollah.yml
vendored
48
.github/workflows/test-eynollah.yml
vendored
|
@ -14,28 +14,14 @@ jobs:
|
|||
python-version: ['3.8', '3.9', '3.10', '3.11']
|
||||
|
||||
steps:
|
||||
- name: clean up
|
||||
run: |
|
||||
df -h
|
||||
sudo rm -rf /usr/share/dotnet
|
||||
sudo rm -rf /usr/local/lib/android
|
||||
sudo rm -rf /opt/ghc
|
||||
sudo rm -rf "/usr/local/share/boost"
|
||||
sudo rm -rf "$AGENT_TOOLSDIRECTORY"
|
||||
df -h
|
||||
- uses: actions/checkout@v4
|
||||
- uses: actions/cache@v4
|
||||
id: seg_model_cache
|
||||
id: model_cache
|
||||
with:
|
||||
path: models_eynollah
|
||||
key: ${{ runner.os }}-models
|
||||
- uses: actions/cache@v4
|
||||
id: bin_model_cache
|
||||
with:
|
||||
path: default-2021-03-09
|
||||
key: ${{ runner.os }}-modelbin
|
||||
- name: Download models
|
||||
if: steps.seg_model_cache.outputs.cache-hit != 'true' || steps.bin_model_cache.outputs.cache-hit != 'true'
|
||||
if: steps.model_cache.outputs.cache-hit != 'true'
|
||||
run: make models
|
||||
- name: Set up Python ${{ matrix.python-version }}
|
||||
uses: actions/setup-python@v5
|
||||
|
@ -44,31 +30,7 @@ jobs:
|
|||
- name: Install dependencies
|
||||
run: |
|
||||
python -m pip install --upgrade pip
|
||||
make install-dev EXTRAS=OCR,plotting
|
||||
make deps-test
|
||||
pip install .
|
||||
pip install -r requirements-test.txt
|
||||
- name: Test with pytest
|
||||
run: make coverage PYTEST_ARGS="-vv --junitxml=pytest.xml"
|
||||
- name: Get coverage results
|
||||
run: |
|
||||
coverage report --format=markdown >> $GITHUB_STEP_SUMMARY
|
||||
coverage html
|
||||
coverage json
|
||||
coverage xml
|
||||
- name: Store coverage results
|
||||
uses: actions/upload-artifact@v4
|
||||
with:
|
||||
name: coverage-report_${{ matrix.python-version }}
|
||||
path: |
|
||||
htmlcov
|
||||
pytest.xml
|
||||
coverage.xml
|
||||
coverage.json
|
||||
- name: Upload coverage results
|
||||
uses: codecov/codecov-action@v4
|
||||
with:
|
||||
files: coverage.xml
|
||||
fail_ci_if_error: false
|
||||
- name: Test standalone CLI
|
||||
run: make smoke-test
|
||||
- name: Test OCR-D CLI
|
||||
run: make ocrd-test
|
||||
run: make test
|
||||
|
|
62
CHANGELOG.md
62
CHANGELOG.md
|
@ -5,68 +5,6 @@ Versioned according to [Semantic Versioning](http://semver.org/).
|
|||
|
||||
## Unreleased
|
||||
|
||||
Fixed:
|
||||
|
||||
* restoring the contour in the original image caused an error due to an empty tuple
|
||||
|
||||
## [0.4.0] - 2025-04-07
|
||||
|
||||
Fixed:
|
||||
|
||||
* allow empty imports for optional dependencies
|
||||
* avoid Numpy warnings (empty slices etc)
|
||||
* remove deprecated Numpy types
|
||||
* binarization CLI: make `dir_in` usable again
|
||||
|
||||
Added:
|
||||
|
||||
* Continuous Deployment via Dockerhub and GHCR
|
||||
* CI: also test CLIs and OCR-D
|
||||
* CI: measure code coverage, annotate+upload reports
|
||||
* smoke-test: also check results
|
||||
* smoke-test: also test sbb-binarize
|
||||
* ocrd-test: analog for OCR-D CLI (segment and binarize)
|
||||
* pytest: add asserts, extend coverage, use subtests for various options
|
||||
* pytest: also add binarization
|
||||
* pytest: add `dir_in` mode (segment and binarize)
|
||||
* make install: control optional dependencies via `EXTRAS` variable
|
||||
* OCR-D: expose and describe recently added parameters:
|
||||
- `ignore_page_extraction`
|
||||
- `allow_enhancement`
|
||||
- `textline_light`
|
||||
- `right_to_left`
|
||||
* OCR-D: :fire: integrate ocrd-sbb-binarize
|
||||
* add detection confidence in `TextRegion/Coords/@conf`
|
||||
(but only in light version and not for marginalia)
|
||||
|
||||
Changed:
|
||||
|
||||
* Docker build: simplify, w/ `OCR`, conform to OCR-D spec
|
||||
* OCR-D: :fire: migrate to core v3
|
||||
- initialize+setup only once
|
||||
- restrict number of parallel page workers to 1
|
||||
(conflicts with existing multiprocessing; TF parts not mp-compatible)
|
||||
- do query maximally annotated page image
|
||||
(but filtering existing binarization/cropping/deskewing),
|
||||
rebase (as new `@imageFilename`) if necessary
|
||||
- add behavioural docstring
|
||||
|
||||
* :fire: refactor `Eynollah` API:
|
||||
- no more data (kw)args at init,
|
||||
but kwargs `dir_in` / `image_filename` for `run()`
|
||||
- no more data attributes, but function kwargs
|
||||
(`pcgts`, `image_filename`, `image_pil`, `dir_in`, `override_dpi`)
|
||||
- remove redundant TF session/model loaders
|
||||
(only load once during init)
|
||||
- factor `run_single()` out of `run()` (loop body),
|
||||
expose for independent calls (like OCR-D)
|
||||
- expose `cache_images()`, add `dpi` kwarg, set `self._imgs`
|
||||
- single-image mode writes PAGE file result
|
||||
(just as directory mode does)
|
||||
|
||||
* CLI: assertions (instead of print+exit) for options checks
|
||||
* light mode: fine-tune ratio to better detect a region as header
|
||||
|
||||
## [0.3.1] - 2024-08-27
|
||||
|
||||
Fixed:
|
||||
|
|
47
Dockerfile
47
Dockerfile
|
@ -1,47 +0,0 @@
|
|||
ARG DOCKER_BASE_IMAGE
|
||||
FROM $DOCKER_BASE_IMAGE
|
||||
|
||||
ARG VCS_REF
|
||||
ARG BUILD_DATE
|
||||
LABEL \
|
||||
maintainer="https://ocr-d.de/en/contact" \
|
||||
org.label-schema.vcs-ref=$VCS_REF \
|
||||
org.label-schema.vcs-url="https://github.com/qurator-spk/eynollah" \
|
||||
org.label-schema.build-date=$BUILD_DATE \
|
||||
org.opencontainers.image.vendor="DFG-Funded Initiative for Optical Character Recognition Development" \
|
||||
org.opencontainers.image.title="Eynollah" \
|
||||
org.opencontainers.image.description="" \
|
||||
org.opencontainers.image.source="https://github.com/qurator-spk/eynollah" \
|
||||
org.opencontainers.image.documentation="https://github.com/qurator-spk/eynollah/blob/${VCS_REF}/README.md" \
|
||||
org.opencontainers.image.revision=$VCS_REF \
|
||||
org.opencontainers.image.created=$BUILD_DATE \
|
||||
org.opencontainers.image.base.name=ocrd/core-cuda-tf2
|
||||
|
||||
ENV DEBIAN_FRONTEND=noninteractive
|
||||
# set proper locales
|
||||
ENV PYTHONIOENCODING=utf8
|
||||
ENV LANG=C.UTF-8
|
||||
ENV LC_ALL=C.UTF-8
|
||||
|
||||
# avoid HOME/.local/share (hard to predict USER here)
|
||||
# so let XDG_DATA_HOME coincide with fixed system location
|
||||
# (can still be overridden by derived stages)
|
||||
ENV XDG_DATA_HOME /usr/local/share
|
||||
# avoid the need for an extra volume for persistent resource user db
|
||||
# (i.e. XDG_CONFIG_HOME/ocrd/resources.yml)
|
||||
ENV XDG_CONFIG_HOME /usr/local/share/ocrd-resources
|
||||
|
||||
WORKDIR /build/eynollah
|
||||
COPY . .
|
||||
COPY ocrd-tool.json .
|
||||
# prepackage ocrd-tool.json as ocrd-all-tool.json
|
||||
RUN ocrd ocrd-tool ocrd-tool.json dump-tools > $(dirname $(ocrd bashlib filename))/ocrd-all-tool.json
|
||||
# prepackage ocrd-all-module-dir.json
|
||||
RUN ocrd ocrd-tool ocrd-tool.json dump-module-dirs > $(dirname $(ocrd bashlib filename))/ocrd-all-module-dir.json
|
||||
# install everything and reduce image size
|
||||
RUN make install EXTRAS=OCR && rm -rf /build/eynollah
|
||||
# smoke test
|
||||
RUN eynollah --help
|
||||
|
||||
WORKDIR /data
|
||||
VOLUME /data
|
115
Makefile
115
Makefile
|
@ -1,21 +1,5 @@
|
|||
PYTHON ?= python3
|
||||
PIP ?= pip3
|
||||
EXTRAS ?=
|
||||
|
||||
# DOCKER_BASE_IMAGE = artefakt.dev.sbb.berlin:5000/sbb/ocrd_core:v2.68.0
|
||||
DOCKER_BASE_IMAGE ?= docker.io/ocrd/core-cuda-tf2:latest
|
||||
DOCKER_TAG ?= ocrd/eynollah
|
||||
DOCKER ?= docker
|
||||
|
||||
#SEG_MODEL := https://qurator-data.de/eynollah/2021-04-25/models_eynollah.tar.gz
|
||||
#SEG_MODEL := https://qurator-data.de/eynollah/2022-04-05/models_eynollah_renamed.tar.gz
|
||||
SEG_MODEL := https://qurator-data.de/eynollah/2022-04-05/models_eynollah.tar.gz
|
||||
#SEG_MODEL := https://github.com/qurator-spk/eynollah/releases/download/v0.3.0/models_eynollah.tar.gz
|
||||
#SEG_MODEL := https://github.com/qurator-spk/eynollah/releases/download/v0.3.1/models_eynollah.tar.gz
|
||||
|
||||
BIN_MODEL := https://github.com/qurator-spk/sbb_binarization/releases/download/v0.0.11/saved_model_2021_03_09.zip
|
||||
|
||||
PYTEST_ARGS ?= -vv
|
||||
EYNOLLAH_MODELS ?= $(PWD)/models_eynollah
|
||||
export EYNOLLAH_MODELS
|
||||
|
||||
# BEGIN-EVAL makefile-parser --make-help Makefile
|
||||
|
||||
|
@ -23,105 +7,44 @@ help:
|
|||
@echo ""
|
||||
@echo " Targets"
|
||||
@echo ""
|
||||
@echo " docker Build Docker image"
|
||||
@echo " build Build Python source and binary distribution"
|
||||
@echo " install Install package with pip"
|
||||
@echo " models Download and extract models to $(PWD)/models_eynollah"
|
||||
@echo " install Install with pip"
|
||||
@echo " install-dev Install editable with pip"
|
||||
@echo " deps-test Install test dependencies with pip"
|
||||
@echo " models Download and extract models to $(CURDIR)/models_eynollah"
|
||||
@echo " smoke-test Run simple CLI check"
|
||||
@echo " ocrd-test Run OCR-D CLI check"
|
||||
@echo " test Run unit tests"
|
||||
@echo ""
|
||||
@echo " Variables"
|
||||
@echo " EXTRAS comma-separated list of features (like 'OCR,plotting') for 'install' [$(EXTRAS)]"
|
||||
@echo " DOCKER_TAG Docker image tag for 'docker' [$(DOCKER_TAG)]"
|
||||
@echo " PYTEST_ARGS pytest args for 'test' (Set to '-s' to see log output during test execution, '-vv' to see individual tests. [$(PYTEST_ARGS)]"
|
||||
@echo " SEG_MODEL URL of 'models' archive to download for segmentation 'test' [$(SEG_MODEL)]"
|
||||
@echo " BIN_MODEL URL of 'models' archive to download for binarization 'test' [$(BIN_MODEL)]"
|
||||
@echo ""
|
||||
|
||||
# END-EVAL
|
||||
|
||||
|
||||
# Download and extract models to $(PWD)/models_eynollah
|
||||
models: models_eynollah default-2021-03-09
|
||||
models: models_eynollah
|
||||
|
||||
models_eynollah: models_eynollah.tar.gz
|
||||
tar zxf models_eynollah.tar.gz
|
||||
# tar xf models_eynollah_renamed.tar.gz --transform 's/models_eynollah_renamed/models_eynollah/'
|
||||
# tar xf models_eynollah_renamed.tar.gz
|
||||
# tar xf models_eynollah_renamed_savedmodel.tar.gz --transform 's/models_eynollah_renamed_savedmodel/models_eynollah/'
|
||||
tar xf models_eynollah.tar.gz
|
||||
|
||||
models_eynollah.tar.gz:
|
||||
wget $(SEG_MODEL)
|
||||
|
||||
default-2021-03-09: $(notdir $(BIN_MODEL))
|
||||
unzip $(notdir $(BIN_MODEL))
|
||||
mkdir $@
|
||||
mv $(basename $(notdir $(BIN_MODEL))) $@
|
||||
|
||||
$(notdir $(BIN_MODEL)):
|
||||
wget $(BIN_MODEL)
|
||||
|
||||
build:
|
||||
$(PIP) install build
|
||||
$(PYTHON) -m build .
|
||||
# wget 'https://qurator-data.de/eynollah/2021-04-25/models_eynollah.tar.gz'
|
||||
# wget 'https://qurator-data.de/eynollah/2022-04-05/models_eynollah_renamed.tar.gz'
|
||||
# wget 'https://ocr-d.kba.cloud/2022-04-05.SavedModel.tar.gz'
|
||||
# wget 'https://qurator-data.de/eynollah/2022-04-05/models_eynollah_renamed_savedmodel.tar.gz'
|
||||
wget https://github.com/qurator-spk/eynollah/releases/download/v0.3.0/models_eynollah.tar.gz
|
||||
|
||||
# Install with pip
|
||||
install:
|
||||
$(PIP) install .$(and $(EXTRAS),[$(EXTRAS)])
|
||||
pip install .
|
||||
|
||||
# Install editable with pip
|
||||
install-dev:
|
||||
$(PIP) install -e .$(and $(EXTRAS),[$(EXTRAS)])
|
||||
pip install -e .
|
||||
|
||||
deps-test: models_eynollah
|
||||
$(PIP) install -r requirements-test.txt
|
||||
|
||||
smoke-test: TMPDIR != mktemp -d
|
||||
smoke-test: tests/resources/kant_aufklaerung_1784_0020.tif
|
||||
# layout analysis:
|
||||
eynollah layout -i $< -o $(TMPDIR) -m $(CURDIR)/models_eynollah
|
||||
fgrep -q http://schema.primaresearch.org/PAGE/gts/pagecontent/2019-07-15 $(TMPDIR)/$(basename $(<F)).xml
|
||||
fgrep -c -e TextRegion -e ImageRegion -e SeparatorRegion $(TMPDIR)/$(basename $(<F)).xml
|
||||
# directory mode (skip one, add one):
|
||||
eynollah layout -di $(<D) -o $(TMPDIR) -m $(CURDIR)/models_eynollah
|
||||
test -s $(TMPDIR)/euler_rechenkunst01_1738_0025.xml
|
||||
# binarize:
|
||||
eynollah binarization -m $(CURDIR)/default-2021-03-09 $< $(TMPDIR)/$(<F)
|
||||
test -s $(TMPDIR)/$(<F)
|
||||
@set -x; test "$$(identify -format '%w %h' $<)" = "$$(identify -format '%w %h' $(TMPDIR)/$(<F))"
|
||||
$(RM) -r $(TMPDIR)
|
||||
|
||||
ocrd-test: export OCRD_MISSING_OUTPUT := ABORT
|
||||
ocrd-test: TMPDIR != mktemp -d
|
||||
ocrd-test: tests/resources/kant_aufklaerung_1784_0020.tif
|
||||
cp $< $(TMPDIR)
|
||||
ocrd workspace -d $(TMPDIR) init
|
||||
ocrd workspace -d $(TMPDIR) add -G OCR-D-IMG -g PHYS_0020 -i OCR-D-IMG_0020 $(<F)
|
||||
ocrd-eynollah-segment -w $(TMPDIR) -I OCR-D-IMG -O OCR-D-SEG -P models $(CURDIR)/models_eynollah
|
||||
result=$$(ocrd workspace -d $(TMPDIR) find -G OCR-D-SEG); \
|
||||
fgrep -q http://schema.primaresearch.org/PAGE/gts/pagecontent/2019-07-15 $(TMPDIR)/$$result && \
|
||||
fgrep -c -e TextRegion -e ImageRegion -e SeparatorRegion $(TMPDIR)/$$result
|
||||
ocrd-sbb-binarize -w $(TMPDIR) -I OCR-D-IMG -O OCR-D-BIN -P model $(CURDIR)/default-2021-03-09
|
||||
ocrd-sbb-binarize -w $(TMPDIR) -I OCR-D-SEG -O OCR-D-SEG-BIN -P model $(CURDIR)/default-2021-03-09 -P operation_level region
|
||||
$(RM) -r $(TMPDIR)
|
||||
smoke-test:
|
||||
eynollah -i tests/resources/kant_aufklaerung_1784_0020.tif -o . -m $(PWD)/models_eynollah
|
||||
|
||||
# Run unit tests
|
||||
test: export EYNOLLAH_MODELS=$(CURDIR)/models_eynollah
|
||||
test: export SBBBIN_MODELS=$(CURDIR)/default-2021-03-09
|
||||
test:
|
||||
$(PYTHON) -m pytest tests --durations=0 --continue-on-collection-errors $(PYTEST_ARGS)
|
||||
|
||||
coverage:
|
||||
coverage erase
|
||||
$(MAKE) test PYTHON="coverage run"
|
||||
coverage report -m
|
||||
|
||||
# Build docker image
|
||||
docker:
|
||||
$(DOCKER) build \
|
||||
--build-arg DOCKER_BASE_IMAGE=$(DOCKER_BASE_IMAGE) \
|
||||
--build-arg VCS_REF=$$(git rev-parse --short HEAD) \
|
||||
--build-arg BUILD_DATE=$$(date -u +"%Y-%m-%dT%H:%M:%SZ") \
|
||||
-t $(DOCKER_TAG) .
|
||||
|
||||
.PHONY: models build install install-dev test smoke-test ocrd-test coverage docker help
|
||||
pytest tests
|
||||
|
|
40
README.md
40
README.md
|
@ -3,7 +3,6 @@
|
|||
|
||||
[](https://pypi.org/project/eynollah/)
|
||||
[](https://github.com/qurator-spk/eynollah/actions/workflows/test-eynollah.yml)
|
||||
[](https://github.com/qurator-spk/eynollah/actions/workflows/build-docker.yml)
|
||||
[](https://opensource.org/license/apache-2-0/)
|
||||
[](https://doi.org/10.1145/3604951.3605513)
|
||||
|
||||
|
@ -22,7 +21,7 @@
|
|||
:warning: Development is currently focused on achieving the best possible quality of results for a wide variety of historical documents and therefore processing can be very slow. We aim to improve this, but contributions are welcome.
|
||||
|
||||
## Installation
|
||||
Python `3.8-3.11` with Tensorflow `<2.13` on Linux are currently supported.
|
||||
Python `3.8-3.11` with Tensorflow `2.12-2.15` on Linux are currently supported.
|
||||
|
||||
For (limited) GPU support the CUDA toolkit needs to be installed.
|
||||
|
||||
|
@ -44,10 +43,10 @@ Alternatively, you can run `make install` or `make install-dev` for editable ins
|
|||
## Models
|
||||
Pre-trained models can be downloaded from [qurator-data.de](https://qurator-data.de/eynollah/) or [huggingface](https://huggingface.co/SBB?search_models=eynollah).
|
||||
|
||||
For documentation on methods and models, have a look at [`models.md`](https://github.com/qurator-spk/eynollah/tree/main/docs/models.md).
|
||||
|
||||
## Train
|
||||
In case you want to train your own model with Eynollah, have a look at [`train.md`](https://github.com/qurator-spk/eynollah/tree/main/docs/train.md).
|
||||
🚧 **Work in progress**
|
||||
|
||||
In case you want to train your own model, have a look at [`sbb_pixelwise_segmentation`](https://github.com/qurator-spk/sbb_pixelwise_segmentation).
|
||||
|
||||
## Usage
|
||||
The command-line interface can be called like this:
|
||||
|
@ -72,7 +71,6 @@ The following options can be used to further configure the processing:
|
|||
| `-cl` | apply contour detection for curved text lines instead of bounding boxes |
|
||||
| `-ib` | apply binarization (the resulting image is saved to the output directory) |
|
||||
| `-ep` | enable plotting (MUST always be used with `-sl`, `-sd`, `-sa`, `-si` or `-ae`) |
|
||||
| `-eoi` | extract only images to output directory (other processing will not be done) |
|
||||
| `-ho` | ignore headers for reading order dectection |
|
||||
| `-si <directory>` | save image regions detected to this directory |
|
||||
| `-sd <directory>` | save deskewed image to this directory |
|
||||
|
@ -84,29 +82,23 @@ If no option is set, the tool performs layout detection of main regions (backgro
|
|||
The best output quality is produced when RGB images are used as input rather than greyscale or binarized images.
|
||||
|
||||
#### Use as OCR-D processor
|
||||
🚧 **Work in progress**
|
||||
|
||||
Eynollah ships with a CLI interface to be used as [OCR-D](https://ocr-d.de) [processor](https://ocr-d.de/en/spec/cli),
|
||||
formally described in [`ocrd-tool.json`](https://github.com/qurator-spk/eynollah/tree/main/src/eynollah/ocrd-tool.json).
|
||||
Eynollah ships with a CLI interface to be used as [OCR-D](https://ocr-d.de) processor.
|
||||
|
||||
In this case, the source image file group with (preferably) RGB images should be used as input like this:
|
||||
|
||||
ocrd-eynollah-segment -I OCR-D-IMG -O OCR-D-SEG -P models 2022-04-05
|
||||
```
|
||||
ocrd-eynollah-segment -I OCR-D-IMG -O SEG-LINE -P models
|
||||
```
|
||||
|
||||
Any image referenced by `@imageFilename` in PAGE-XML is passed on directly to Eynollah as a processor, so that e.g.
|
||||
|
||||
|
||||
If the input file group is PAGE-XML (from a previous OCR-D workflow step), Eynollah behaves as follows:
|
||||
- existing regions are kept and ignored (i.e. in effect they might overlap segments from Eynollah results)
|
||||
- existing annotation (and respective `AlternativeImage`s) are partially _ignored_:
|
||||
- previous page frame detection (`cropped` images)
|
||||
- previous derotation (`deskewed` images)
|
||||
- previous thresholding (`binarized` images)
|
||||
- if the page-level image nevertheless deviates from the original (`@imageFilename`)
|
||||
(because some other preprocessing step was in effect like `denoised`), then
|
||||
the output PAGE-XML will be based on that as new top-level (`@imageFilename`)
|
||||
|
||||
|
||||
ocrd-eynollah-segment -I OCR-D-XYZ -O OCR-D-SEG -P models 2022-04-05
|
||||
|
||||
Still, in general, it makes more sense to add other workflow steps **after** Eynollah.
|
||||
```
|
||||
ocrd-eynollah-segment -I OCR-D-IMG-BIN -O SEG-LINE -P models
|
||||
```
|
||||
|
||||
uses the original (RGB) image despite any binarization that may have occured in previous OCR-D processing steps
|
||||
|
||||
#### Additional documentation
|
||||
Please check the [wiki](https://github.com/qurator-spk/eynollah/wiki).
|
||||
|
|
147
docs/models.md
147
docs/models.md
|
@ -1,147 +0,0 @@
|
|||
# Models documentation
|
||||
This suite of 14 models presents a document layout analysis (DLA) system for historical documents implemented by
|
||||
pixel-wise segmentation using a combination of a ResNet50 encoder with various U-Net decoders. In addition, heuristic
|
||||
methods are applied to detect marginals and to determine the reading order of text regions.
|
||||
|
||||
The detection and classification of multiple classes of layout elements such as headings, images, tables etc. as part of
|
||||
DLA is required in order to extract and process them in subsequent steps. Altogether, the combination of image
|
||||
detection, classification and segmentation on the wide variety that can be found in over 400 years of printed cultural
|
||||
heritage makes this a very challenging task. Deep learning models are complemented with heuristics for the detection of
|
||||
text lines, marginals, and reading order. Furthermore, an optional image enhancement step was added in case of documents
|
||||
that either have insufficient pixel density and/or require scaling. Also, a column classifier for the analysis of
|
||||
multi-column documents was added. With these additions, DLA performance was improved, and a high accuracy in the
|
||||
prediction of the reading order is accomplished.
|
||||
|
||||
Two Arabic/Persian terms form the name of the model suite: عين الله, which can be transcribed as "ain'allah" or
|
||||
"eynollah"; it translates into English as "God's Eye" -- it sees (nearly) everything on the document image.
|
||||
|
||||
See the flowchart below for the different stages and how they interact:
|
||||
|
||||
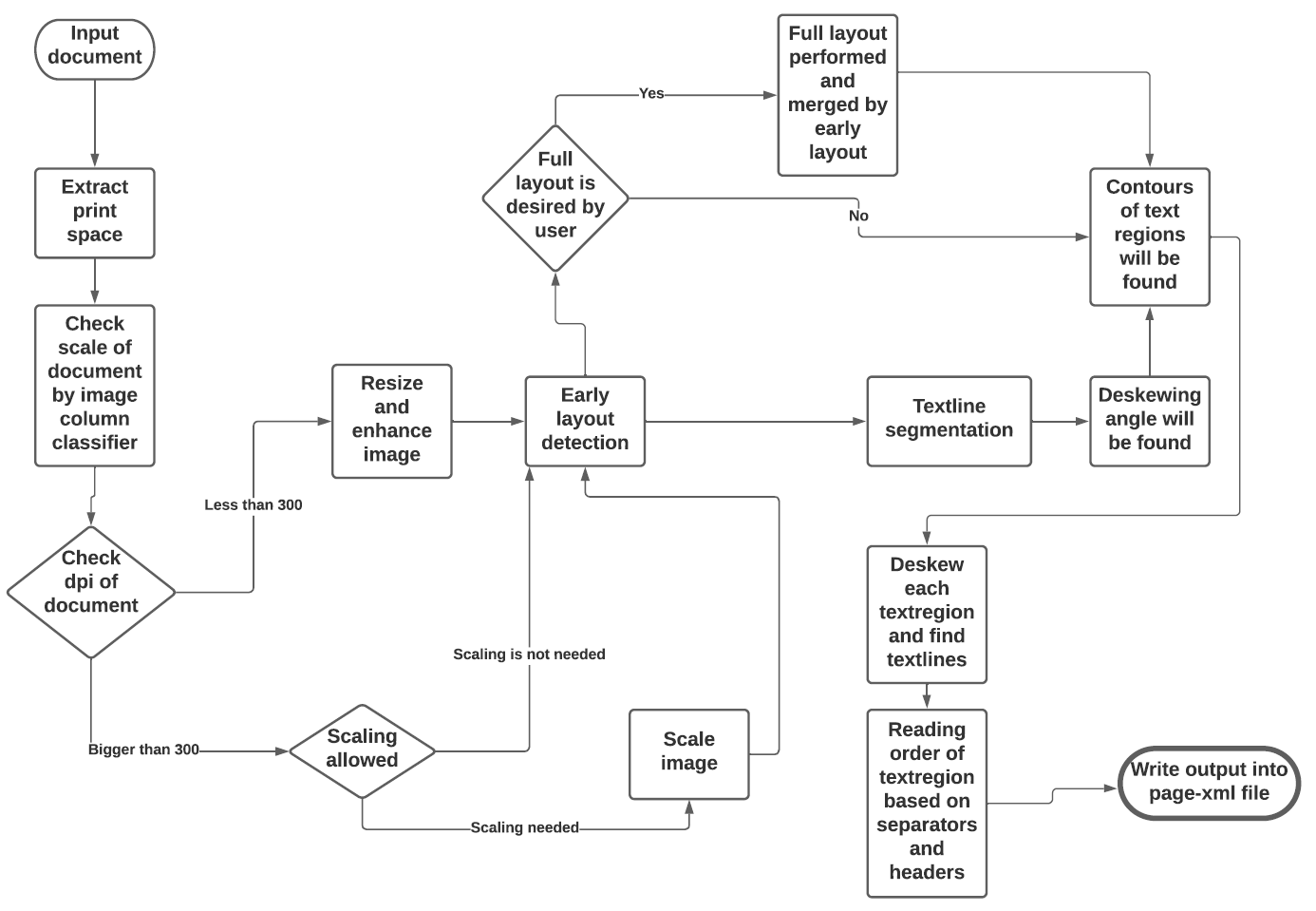
|
||||
|
||||
|
||||
## Models
|
||||
|
||||
### Image enhancement
|
||||
Model card: [Image Enhancement](https://huggingface.co/SBB/eynollah-enhancement)
|
||||
|
||||
This model addresses image resolution, specifically targeting documents with suboptimal resolution. In instances where
|
||||
the detection of document layout exhibits inadequate performance, the proposed enhancement aims to significantly improve
|
||||
the quality and clarity of the images, thus facilitating enhanced visual interpretation and analysis.
|
||||
|
||||
### Page extraction / border detection
|
||||
Model card: [Page Extraction/Border Detection](https://huggingface.co/SBB/eynollah-page-extraction)
|
||||
|
||||
A problem that can negatively affect OCR are black margins around a page caused by document scanning. A deep learning
|
||||
model helps to crop to the page borders by using a pixel-wise segmentation method.
|
||||
|
||||
### Column classification
|
||||
Model card: [Column Classification](https://huggingface.co/SBB/eynollah-column-classifier)
|
||||
|
||||
This model is a trained classifier that recognizes the number of columns in a document by use of a training set with
|
||||
manual classification of all documents into six classes with either one, two, three, four, five, or six and more columns
|
||||
respectively.
|
||||
|
||||
### Binarization
|
||||
Model card: [Binarization](https://huggingface.co/SBB/eynollah-binarization)
|
||||
|
||||
This model is designed to tackle the intricate task of document image binarization, which involves segmentation of the
|
||||
image into white and black pixels. This process significantly contributes to the overall performance of the layout
|
||||
models, particularly in scenarios where the documents are degraded or exhibit subpar quality. The robust binarization
|
||||
capability of the model enables improved accuracy and reliability in subsequent layout analysis, thereby facilitating
|
||||
enhanced document understanding and interpretation.
|
||||
|
||||
### Main region detection
|
||||
Model card: [Main Region Detection](https://huggingface.co/SBB/eynollah-main-regions)
|
||||
|
||||
This model has employed a different set of labels, including an artificial class specifically designed to encompass the
|
||||
text regions. The inclusion of this artificial class facilitates easier isolation of text regions by the model. This
|
||||
approach grants the advantage of training the model using downscaled images, which in turn leads to faster predictions
|
||||
during the inference phase. By incorporating this methodology, improved efficiency is achieved without compromising the
|
||||
model's ability to accurately identify and classify text regions within documents.
|
||||
|
||||
### Main region detection (with scaling augmentation)
|
||||
Model card: [Main Region Detection (with scaling augmentation)](https://huggingface.co/SBB/eynollah-main-regions-aug-scaling)
|
||||
|
||||
Utilizing scaling augmentation, this model leverages the capability to effectively segment elements of extremely high or
|
||||
low scales within documents. By harnessing this technique, the tool gains a significant advantage in accurately
|
||||
categorizing and isolating such elements, thereby enhancing its overall performance and enabling precise analysis of
|
||||
documents with varying scale characteristics.
|
||||
|
||||
### Main region detection (with rotation augmentation)
|
||||
Model card: [Main Region Detection (with rotation augmentation)](https://huggingface.co/SBB/eynollah-main-regions-aug-rotation)
|
||||
|
||||
This model takes advantage of rotation augmentation. This helps the tool to segment the vertical text regions in a
|
||||
robust way.
|
||||
|
||||
### Main region detection (ensembled)
|
||||
Model card: [Main Region Detection (ensembled)](https://huggingface.co/SBB/eynollah-main-regions-ensembled)
|
||||
|
||||
The robustness of this model is attained through an ensembling technique that combines the weights from various epochs.
|
||||
By employing this approach, the model achieves a high level of resilience and stability, effectively leveraging the
|
||||
strengths of multiple epochs to enhance its overall performance and deliver consistent and reliable results.
|
||||
|
||||
### Full region detection (1,2-column documents)
|
||||
Model card: [Full Region Detection (1,2-column documents)](https://huggingface.co/SBB/eynollah-full-regions-1column)
|
||||
|
||||
This model deals with documents comprising of one and two columns.
|
||||
|
||||
### Full region detection (3,n-column documents)
|
||||
Model card: [Full Region Detection (3,n-column documents)](https://huggingface.co/SBB/eynollah-full-regions-3pluscolumn)
|
||||
|
||||
This model is responsible for detecting headers and drop capitals in documents with three or more columns.
|
||||
|
||||
### Textline detection
|
||||
Model card: [Textline Detection](https://huggingface.co/SBB/eynollah-textline)
|
||||
|
||||
The method for textline detection combines deep learning and heuristics. In the deep learning part, an image-to-image
|
||||
model performs binary segmentation of the document into the classes textline vs. background. In the heuristics part,
|
||||
bounding boxes or contours are derived from binary segmentation.
|
||||
|
||||
Skewed documents can heavily affect textline detection accuracy, so robust deskewing is needed. But detecting textlines
|
||||
with rectangle bounding boxes cannot deal with partially curved textlines. To address this, a functionality
|
||||
specifically for documents with curved textlines was included. After finding the contour of a text region and its
|
||||
corresponding textline segmentation, the text region is cut into smaller vertical straps. For each strap, its textline
|
||||
segmentation is first deskewed and then the textlines are separated with the same heuristic method as for finding
|
||||
textline bounding boxes. Later, the strap is rotated back into its original orientation.
|
||||
|
||||
### Textline detection (light)
|
||||
Model card: [Textline Detection Light (simpler but faster method)](https://huggingface.co/SBB/eynollah-textline_light)
|
||||
|
||||
The method for textline detection combines deep learning and heuristics. In the deep learning part, an image-to-image
|
||||
model performs binary segmentation of the document into the classes textline vs. background. In the heuristics part,
|
||||
bounding boxes or contours are derived from binary segmentation.
|
||||
|
||||
In the context of this textline model, a distinct labeling approach has been employed to ensure accurate predictions.
|
||||
Specifically, an artificial bounding class has been incorporated alongside the textline classes. This strategic
|
||||
inclusion effectively prevents any spurious connections between adjacent textlines during the prediction phase, thereby
|
||||
enhancing the model's ability to accurately identify and delineate individual textlines within documents. This model
|
||||
eliminates the need for additional heuristics in extracting textline contours.
|
||||
|
||||
### Table detection
|
||||
Model card: [Table Detection](https://huggingface.co/SBB/eynollah-tables)
|
||||
|
||||
The objective of this model is to perform table segmentation in historical document images. Due to the pixel-wise
|
||||
segmentation approach employed and the presence of traditional tables predominantly composed of text, the detection of
|
||||
tables required the incorporation of heuristics to achieve reasonable performance. These heuristics were necessary to
|
||||
effectively identify and delineate tables within the historical document images, ensuring accurate segmentation and
|
||||
enabling subsequent analysis and interpretation.
|
||||
|
||||
### Image detection
|
||||
Model card: [Image Detection](https://huggingface.co/SBB/eynollah-image-extraction)
|
||||
|
||||
This model is used for the task of illustration detection only.
|
||||
|
||||
### Reading order detection
|
||||
Model card: [Reading Order Detection]()
|
||||
|
||||
TODO
|
||||
|
||||
## Heuristic methods
|
||||
Additionally, some heuristic methods are employed to further improve the model predictions:
|
||||
* After border detection, the largest contour is determined by a bounding box, and the image cropped to these coordinates.
|
||||
* For text region detection, the image is scaled up to make it easier for the model to detect background space between text regions.
|
||||
* A minimum area is defined for text regions in relation to the overall image dimensions, so that very small regions that are noise can be filtered out.
|
||||
* Deskewing is applied on the text region level (due to regions having different degrees of skew) in order to improve the textline segmentation result.
|
||||
* After deskewing, a calculation of the pixel distribution on the X-axis allows the separation of textlines (foreground) and background pixels.
|
||||
* Finally, using the derived coordinates, bounding boxes are determined for each textline.
|
632
docs/train.md
632
docs/train.md
|
@ -1,632 +0,0 @@
|
|||
# Training documentation
|
||||
This aims to assist users in preparing training datasets, training models, and performing inference with trained models.
|
||||
We cover various use cases including pixel-wise segmentation, image classification, image enhancement, and machine-based
|
||||
reading order detection. For each use case, we provide guidance on how to generate the corresponding training dataset.
|
||||
|
||||
The following three tasks can all be accomplished using the code in the
|
||||
[`train`](https://github.com/qurator-spk/sbb_pixelwise_segmentation/tree/unifying-training-models) directory:
|
||||
|
||||
* generate training dataset
|
||||
* train a model
|
||||
* inference with the trained model
|
||||
|
||||
## Generate training dataset
|
||||
The script `generate_gt_for_training.py` is used for generating training datasets. As the results of the following
|
||||
command demonstrates, the dataset generator provides three different commands:
|
||||
|
||||
`python generate_gt_for_training.py --help`
|
||||
|
||||
These three commands are:
|
||||
|
||||
* image-enhancement
|
||||
* machine-based-reading-order
|
||||
* pagexml2label
|
||||
|
||||
### image-enhancement
|
||||
Generating a training dataset for image enhancement is quite straightforward. All that is needed is a set of
|
||||
high-resolution images. The training dataset can then be generated using the following command:
|
||||
|
||||
`python generate_gt_for_training.py image-enhancement -dis "dir of high resolution images" -dois "dir where degraded
|
||||
images will be written" -dols "dir where the corresponding high resolution image will be written as label" -scs
|
||||
"degrading scales json file"`
|
||||
|
||||
The scales JSON file is a dictionary with a key named 'scales' and values representing scales smaller than 1. Images are
|
||||
downscaled based on these scales and then upscaled again to their original size. This process causes the images to lose
|
||||
resolution at different scales. The degraded images are used as input images, and the original high-resolution images
|
||||
serve as labels. The enhancement model can be trained with this generated dataset. The scales JSON file looks like this:
|
||||
|
||||
```yaml
|
||||
{
|
||||
"scales": [0.5, 0.55, 0.6, 0.65, 0.7, 0.75, 0.8, 0.85, 0.9]
|
||||
}
|
||||
```
|
||||
|
||||
### machine-based-reading-order
|
||||
For machine-based reading order, we aim to determine the reading priority between two sets of text regions. The model's
|
||||
input is a three-channel image: the first and last channels contain information about each of the two text regions,
|
||||
while the middle channel encodes prominent layout elements necessary for reading order, such as separators and headers.
|
||||
To generate the training dataset, our script requires a page XML file that specifies the image layout with the correct
|
||||
reading order.
|
||||
|
||||
For output images, it is necessary to specify the width and height. Additionally, a minimum text region size can be set
|
||||
to filter out regions smaller than this minimum size. This minimum size is defined as the ratio of the text region area
|
||||
to the image area, with a default value of zero. To run the dataset generator, use the following command:
|
||||
|
||||
`python generate_gt_for_training.py machine-based-reading-order -dx "dir of GT xml files" -domi "dir where output images
|
||||
will be written" -docl "dir where the labels will be written" -ih "height" -iw "width" -min "min area ratio"`
|
||||
|
||||
### pagexml2label
|
||||
pagexml2label is designed to generate labels from GT page XML files for various pixel-wise segmentation use cases,
|
||||
including 'layout,' 'textline,' 'printspace,' 'glyph,' and 'word' segmentation.
|
||||
To train a pixel-wise segmentation model, we require images along with their corresponding labels. Our training script
|
||||
expects a PNG image where each pixel corresponds to a label, represented by an integer. The background is always labeled
|
||||
as zero, while other elements are assigned different integers. For instance, if we have ground truth data with four
|
||||
elements including the background, the classes would be labeled as 0, 1, 2, and 3 respectively.
|
||||
|
||||
In binary segmentation scenarios such as textline or page extraction, the background is encoded as 0, and the desired
|
||||
element is automatically encoded as 1 in the PNG label.
|
||||
|
||||
To specify the desired use case and the elements to be extracted in the PNG labels, a custom JSON file can be passed.
|
||||
For example, in the case of 'textline' detection, the JSON file would resemble this:
|
||||
|
||||
```yaml
|
||||
{
|
||||
"use_case": "textline"
|
||||
}
|
||||
```
|
||||
|
||||
In the case of layout segmentation a custom config json file can look like this:
|
||||
|
||||
```yaml
|
||||
{
|
||||
"use_case": "layout",
|
||||
"textregions":{"rest_as_paragraph":1 , "drop-capital": 1, "header":2, "heading":2, "marginalia":3},
|
||||
"imageregion":4,
|
||||
"separatorregion":5,
|
||||
"graphicregions" :{"rest_as_decoration":6 ,"stamp":7}
|
||||
}
|
||||
```
|
||||
|
||||
A possible custom config json file for layout segmentation where the "printspace" is a class:
|
||||
|
||||
```yaml
|
||||
{
|
||||
"use_case": "layout",
|
||||
"textregions":{"rest_as_paragraph":1 , "drop-capital": 1, "header":2, "heading":2, "marginalia":3},
|
||||
"imageregion":4,
|
||||
"separatorregion":5,
|
||||
"graphicregions" :{"rest_as_decoration":6 ,"stamp":7}
|
||||
"printspace_as_class_in_layout" : 8
|
||||
}
|
||||
```
|
||||
|
||||
For the layout use case, it is beneficial to first understand the structure of the page XML file and its elements.
|
||||
In a given image, the annotations of elements are recorded in a page XML file, including their contours and classes.
|
||||
For an image document, the known regions are 'textregion', 'separatorregion', 'imageregion', 'graphicregion',
|
||||
'noiseregion', and 'tableregion'.
|
||||
|
||||
Text regions and graphic regions also have their own specific types. The known types for text regions are 'paragraph',
|
||||
'header', 'heading', 'marginalia', 'drop-capital', 'footnote', 'footnote-continued', 'signature-mark', 'page-number',
|
||||
and 'catch-word'. The known types for graphic regions are 'handwritten-annotation', 'decoration', 'stamp', and
|
||||
'signature'.
|
||||
Since we don't know all types of text and graphic regions, unknown cases can arise. To handle these, we have defined
|
||||
two additional types, "rest_as_paragraph" and "rest_as_decoration", to ensure that no unknown types are missed.
|
||||
This way, users can extract all known types from the labels and be confident that no unknown types are overlooked.
|
||||
|
||||
In the custom JSON file shown above, "header" and "heading" are extracted as the same class, while "marginalia" is shown
|
||||
as a different class. All other text region types, including "drop-capital," are grouped into the same class. For the
|
||||
graphic region, "stamp" has its own class, while all other types are classified together. "Image region" and "separator
|
||||
region" are also present in the label. However, other regions like "noise region" and "table region" will not be
|
||||
included in the label PNG file, even if they have information in the page XML files, as we chose not to include them.
|
||||
|
||||
`python generate_gt_for_training.py pagexml2label -dx "dir of GT xml files" -do "dir where output label png files will
|
||||
be written" -cfg "custom config json file" -to "output type which has 2d and 3d. 2d is used for training and 3d is just
|
||||
to visualise the labels" "`
|
||||
|
||||
We have also defined an artificial class that can be added to the boundary of text region types or text lines. This key
|
||||
is called "artificial_class_on_boundary." If users want to apply this to certain text regions in the layout use case,
|
||||
the example JSON config file should look like this:
|
||||
|
||||
```yaml
|
||||
{
|
||||
"use_case": "layout",
|
||||
"textregions": {
|
||||
"paragraph": 1,
|
||||
"drop-capital": 1,
|
||||
"header": 2,
|
||||
"heading": 2,
|
||||
"marginalia": 3
|
||||
},
|
||||
"imageregion": 4,
|
||||
"separatorregion": 5,
|
||||
"graphicregions": {
|
||||
"rest_as_decoration": 6
|
||||
},
|
||||
"artificial_class_on_boundary": ["paragraph", "header", "heading", "marginalia"],
|
||||
"artificial_class_label": 7
|
||||
}
|
||||
```
|
||||
|
||||
This implies that the artificial class label, denoted by 7, will be present on PNG files and will only be added to the
|
||||
elements labeled as "paragraph," "header," "heading," and "marginalia."
|
||||
|
||||
For "textline", "word", and "glyph", the artificial class on the boundaries will be activated only if the
|
||||
"artificial_class_label" key is specified in the config file. Its value should be set as 2 since these elements
|
||||
represent binary cases. For example, if the background and textline are denoted as 0 and 1 respectively, then the
|
||||
artificial class should be assigned the value 2. The example JSON config file should look like this for "textline" use
|
||||
case:
|
||||
|
||||
```yaml
|
||||
{
|
||||
"use_case": "textline",
|
||||
"artificial_class_label": 2
|
||||
}
|
||||
```
|
||||
|
||||
If the coordinates of "PrintSpace" or "Border" are present in the page XML ground truth files, and the user wishes to
|
||||
crop only the print space area, this can be achieved by activating the "-ps" argument. However, it should be noted that
|
||||
in this scenario, since cropping will be applied to the label files, the directory of the original images must be
|
||||
provided to ensure that they are cropped in sync with the labels. This ensures that the correct images and labels
|
||||
required for training are obtained. The command should resemble the following:
|
||||
|
||||
`python generate_gt_for_training.py pagexml2label -dx "dir of GT xml files" -do "dir where output label png files will
|
||||
be written" -cfg "custom config json file" -to "output type which has 2d and 3d. 2d is used for training and 3d is just
|
||||
to visualise the labels" -ps -di "dir where the org images are located" -doi "dir where the cropped output images will
|
||||
be written" `
|
||||
|
||||
## Train a model
|
||||
### classification
|
||||
|
||||
For the classification use case, we haven't provided a ground truth generator, as it's unnecessary. For classification,
|
||||
all we require is a training directory with subdirectories, each containing images of its respective classes. We need
|
||||
separate directories for training and evaluation, and the class names (subdirectories) must be consistent across both
|
||||
directories. Additionally, the class names should be specified in the config JSON file, as shown in the following
|
||||
example. If, for instance, we aim to classify "apple" and "orange," with a total of 2 classes, the
|
||||
"classification_classes_name" key in the config file should appear as follows:
|
||||
|
||||
```yaml
|
||||
{
|
||||
"backbone_type" : "nontransformer",
|
||||
"task": "classification",
|
||||
"n_classes" : 2,
|
||||
"n_epochs" : 10,
|
||||
"input_height" : 448,
|
||||
"input_width" : 448,
|
||||
"weight_decay" : 1e-6,
|
||||
"n_batch" : 4,
|
||||
"learning_rate": 1e-4,
|
||||
"f1_threshold_classification": 0.8,
|
||||
"pretraining" : true,
|
||||
"classification_classes_name" : {"0":"apple", "1":"orange"},
|
||||
"dir_train": "./train",
|
||||
"dir_eval": "./eval",
|
||||
"dir_output": "./output"
|
||||
}
|
||||
```
|
||||
|
||||
The "dir_train" should be like this:
|
||||
|
||||
```
|
||||
.
|
||||
└── train # train directory
|
||||
├── apple # directory of images for apple class
|
||||
└── orange # directory of images for orange class
|
||||
```
|
||||
|
||||
And the "dir_eval" the same structure as train directory:
|
||||
|
||||
```
|
||||
.
|
||||
└── eval # evaluation directory
|
||||
├── apple # directory of images for apple class
|
||||
└── orange # directory of images for orange class
|
||||
|
||||
```
|
||||
|
||||
The classification model can be trained using the following command line:
|
||||
|
||||
`python train.py with config_classification.json`
|
||||
|
||||
As evident in the example JSON file above, for classification, we utilize a "f1_threshold_classification" parameter.
|
||||
This parameter is employed to gather all models with an evaluation f1 score surpassing this threshold. Subsequently,
|
||||
an ensemble of these model weights is executed, and a model is saved in the output directory as "model_ens_avg".
|
||||
Additionally, the weight of the best model based on the evaluation f1 score is saved as "model_best".
|
||||
|
||||
### reading order
|
||||
An example config json file for machine based reading order should be like this:
|
||||
|
||||
```yaml
|
||||
{
|
||||
"backbone_type" : "nontransformer",
|
||||
"task": "reading_order",
|
||||
"n_classes" : 1,
|
||||
"n_epochs" : 5,
|
||||
"input_height" : 672,
|
||||
"input_width" : 448,
|
||||
"weight_decay" : 1e-6,
|
||||
"n_batch" : 4,
|
||||
"learning_rate": 1e-4,
|
||||
"pretraining" : true,
|
||||
"dir_train": "./train",
|
||||
"dir_eval": "./eval",
|
||||
"dir_output": "./output"
|
||||
}
|
||||
```
|
||||
|
||||
The "dir_train" should be like this:
|
||||
|
||||
```
|
||||
.
|
||||
└── train # train directory
|
||||
├── images # directory of images
|
||||
└── labels # directory of labels
|
||||
```
|
||||
|
||||
And the "dir_eval" the same structure as train directory:
|
||||
|
||||
```
|
||||
.
|
||||
└── eval # evaluation directory
|
||||
├── images # directory of images
|
||||
└── labels # directory of labels
|
||||
```
|
||||
|
||||
The classification model can be trained like the classification case command line.
|
||||
|
||||
### Segmentation (Textline, Binarization, Page extraction and layout) and enhancement
|
||||
|
||||
#### Parameter configuration for segmentation or enhancement usecases
|
||||
The following parameter configuration can be applied to all segmentation use cases and enhancements. The augmentation,
|
||||
its sub-parameters, and continued training are defined only for segmentation use cases and enhancements, not for
|
||||
classification and machine-based reading order, as you can see in their example config files.
|
||||
|
||||
* backbone_type: For segmentation tasks (such as text line, binarization, and layout detection) and enhancement, we
|
||||
* offer two backbone options: a "nontransformer" and a "transformer" backbone. For the "transformer" backbone, we first
|
||||
* apply a CNN followed by a transformer. In contrast, the "nontransformer" backbone utilizes only a CNN ResNet-50.
|
||||
* task : The task parameter can have values such as "segmentation", "enhancement", "classification", and "reading_order".
|
||||
* patches: If you want to break input images into smaller patches (input size of the model) you need to set this
|
||||
* parameter to ``true``. In the case that the model should see the image once, like page extraction, patches should be
|
||||
* set to ``false``.
|
||||
* n_batch: Number of batches at each iteration.
|
||||
* n_classes: Number of classes. In the case of binary classification this should be 2. In the case of reading_order it
|
||||
* should set to 1. And for the case of layout detection just the unique number of classes should be given.
|
||||
* n_epochs: Number of epochs.
|
||||
* input_height: This indicates the height of model's input.
|
||||
* input_width: This indicates the width of model's input.
|
||||
* weight_decay: Weight decay of l2 regularization of model layers.
|
||||
* pretraining: Set to ``true`` to load pretrained weights of ResNet50 encoder. The downloaded weights should be saved
|
||||
* in a folder named "pretrained_model" in the same directory of "train.py" script.
|
||||
* augmentation: If you want to apply any kind of augmentation this parameter should first set to ``true``.
|
||||
* flip_aug: If ``true``, different types of filp will be applied on image. Type of flips is given with "flip_index" parameter.
|
||||
* blur_aug: If ``true``, different types of blurring will be applied on image. Type of blurrings is given with "blur_k" parameter.
|
||||
* scaling: If ``true``, scaling will be applied on image. Scale of scaling is given with "scales" parameter.
|
||||
* degrading: If ``true``, degrading will be applied to the image. The amount of degrading is defined with "degrade_scales" parameter.
|
||||
* brightening: If ``true``, brightening will be applied to the image. The amount of brightening is defined with "brightness" parameter.
|
||||
* rotation_not_90: If ``true``, rotation (not 90 degree) will be applied on image. Rotation angles are given with "thetha" parameter.
|
||||
* rotation: If ``true``, 90 degree rotation will be applied on image.
|
||||
* binarization: If ``true``,Otsu thresholding will be applied to augment the input data with binarized images.
|
||||
* scaling_bluring: If ``true``, combination of scaling and blurring will be applied on image.
|
||||
* scaling_binarization: If ``true``, combination of scaling and binarization will be applied on image.
|
||||
* scaling_flip: If ``true``, combination of scaling and flip will be applied on image.
|
||||
* flip_index: Type of flips.
|
||||
* blur_k: Type of blurrings.
|
||||
* scales: Scales of scaling.
|
||||
* brightness: The amount of brightenings.
|
||||
* thetha: Rotation angles.
|
||||
* degrade_scales: The amount of degradings.
|
||||
* continue_training: If ``true``, it means that you have already trained a model and you would like to continue the training. So it is needed to provide the dir of trained model with "dir_of_start_model" and index for naming the models. For example if you have already trained for 3 epochs then your last index is 2 and if you want to continue from model_1.h5, you can set ``index_start`` to 3 to start naming model with index 3.
|
||||
* weighted_loss: If ``true``, this means that you want to apply weighted categorical_crossentropy as loss fucntion. Be carefull if you set to ``true``the parameter "is_loss_soft_dice" should be ``false``
|
||||
* data_is_provided: If you have already provided the input data you can set this to ``true``. Be sure that the train and eval data are in "dir_output". Since when once we provide training data we resize and augment them and then we write them in sub-directories train and eval in "dir_output".
|
||||
* dir_train: This is the directory of "images" and "labels" (dir_train should include two subdirectories with names of images and labels ) for raw images and labels. Namely they are not prepared (not resized and not augmented) yet for training the model. When we run this tool these raw data will be transformed to suitable size needed for the model and they will be written in "dir_output" in train and eval directories. Each of train and eval include "images" and "labels" sub-directories.
|
||||
* index_start: Starting index for saved models in the case that "continue_training" is ``true``.
|
||||
* dir_of_start_model: Directory containing pretrained model to continue training the model in the case that "continue_training" is ``true``.
|
||||
* transformer_num_patches_xy: Number of patches for vision transformer in x and y direction respectively.
|
||||
* transformer_patchsize_x: Patch size of vision transformer patches in x direction.
|
||||
* transformer_patchsize_y: Patch size of vision transformer patches in y direction.
|
||||
* transformer_projection_dim: Transformer projection dimension. Default value is 64.
|
||||
* transformer_mlp_head_units: Transformer Multilayer Perceptron (MLP) head units. Default value is [128, 64].
|
||||
* transformer_layers: transformer layers. Default value is 8.
|
||||
* transformer_num_heads: Transformer number of heads. Default value is 4.
|
||||
* transformer_cnn_first: We have two types of vision transformers. In one type, a CNN is applied first, followed by a transformer. In the other type, this order is reversed. If transformer_cnn_first is true, it means the CNN will be applied before the transformer. Default value is true.
|
||||
|
||||
In the case of segmentation and enhancement the train and evaluation directory should be as following.
|
||||
|
||||
The "dir_train" should be like this:
|
||||
|
||||
```
|
||||
.
|
||||
└── train # train directory
|
||||
├── images # directory of images
|
||||
└── labels # directory of labels
|
||||
```
|
||||
|
||||
And the "dir_eval" the same structure as train directory:
|
||||
|
||||
```
|
||||
.
|
||||
└── eval # evaluation directory
|
||||
├── images # directory of images
|
||||
└── labels # directory of labels
|
||||
```
|
||||
|
||||
After configuring the JSON file for segmentation or enhancement, training can be initiated by running the following
|
||||
command, similar to the process for classification and reading order:
|
||||
|
||||
`python train.py with config_classification.json`
|
||||
|
||||
#### Binarization
|
||||
An example config json file for binarization can be like this:
|
||||
|
||||
```yaml
|
||||
{
|
||||
"backbone_type" : "transformer",
|
||||
"task": "binarization",
|
||||
"n_classes" : 2,
|
||||
"n_epochs" : 4,
|
||||
"input_height" : 224,
|
||||
"input_width" : 672,
|
||||
"weight_decay" : 1e-6,
|
||||
"n_batch" : 1,
|
||||
"learning_rate": 1e-4,
|
||||
"patches" : true,
|
||||
"pretraining" : true,
|
||||
"augmentation" : true,
|
||||
"flip_aug" : false,
|
||||
"blur_aug" : false,
|
||||
"scaling" : true,
|
||||
"degrading": false,
|
||||
"brightening": false,
|
||||
"binarization" : false,
|
||||
"scaling_bluring" : false,
|
||||
"scaling_binarization" : false,
|
||||
"scaling_flip" : false,
|
||||
"rotation": false,
|
||||
"rotation_not_90": false,
|
||||
"transformer_num_patches_xy": [7, 7],
|
||||
"transformer_patchsize_x": 3,
|
||||
"transformer_patchsize_y": 1,
|
||||
"transformer_projection_dim": 192,
|
||||
"transformer_mlp_head_units": [128, 64],
|
||||
"transformer_layers": 8,
|
||||
"transformer_num_heads": 4,
|
||||
"transformer_cnn_first": true,
|
||||
"blur_k" : ["blur","guass","median"],
|
||||
"scales" : [0.6, 0.7, 0.8, 0.9, 1.1, 1.2, 1.4],
|
||||
"brightness" : [1.3, 1.5, 1.7, 2],
|
||||
"degrade_scales" : [0.2, 0.4],
|
||||
"flip_index" : [0, 1, -1],
|
||||
"thetha" : [10, -10],
|
||||
"continue_training": false,
|
||||
"index_start" : 0,
|
||||
"dir_of_start_model" : " ",
|
||||
"weighted_loss": false,
|
||||
"is_loss_soft_dice": false,
|
||||
"data_is_provided": false,
|
||||
"dir_train": "./train",
|
||||
"dir_eval": "./eval",
|
||||
"dir_output": "./output"
|
||||
}
|
||||
```
|
||||
|
||||
#### Textline
|
||||
|
||||
```yaml
|
||||
{
|
||||
"backbone_type" : "nontransformer",
|
||||
"task": "segmentation",
|
||||
"n_classes" : 2,
|
||||
"n_epochs" : 4,
|
||||
"input_height" : 448,
|
||||
"input_width" : 224,
|
||||
"weight_decay" : 1e-6,
|
||||
"n_batch" : 1,
|
||||
"learning_rate": 1e-4,
|
||||
"patches" : true,
|
||||
"pretraining" : true,
|
||||
"augmentation" : true,
|
||||
"flip_aug" : false,
|
||||
"blur_aug" : false,
|
||||
"scaling" : true,
|
||||
"degrading": false,
|
||||
"brightening": false,
|
||||
"binarization" : false,
|
||||
"scaling_bluring" : false,
|
||||
"scaling_binarization" : false,
|
||||
"scaling_flip" : false,
|
||||
"rotation": false,
|
||||
"rotation_not_90": false,
|
||||
"blur_k" : ["blur","guass","median"],
|
||||
"scales" : [0.6, 0.7, 0.8, 0.9, 1.1, 1.2, 1.4],
|
||||
"brightness" : [1.3, 1.5, 1.7, 2],
|
||||
"degrade_scales" : [0.2, 0.4],
|
||||
"flip_index" : [0, 1, -1],
|
||||
"thetha" : [10, -10],
|
||||
"continue_training": false,
|
||||
"index_start" : 0,
|
||||
"dir_of_start_model" : " ",
|
||||
"weighted_loss": false,
|
||||
"is_loss_soft_dice": false,
|
||||
"data_is_provided": false,
|
||||
"dir_train": "./train",
|
||||
"dir_eval": "./eval",
|
||||
"dir_output": "./output"
|
||||
}
|
||||
```
|
||||
|
||||
#### Enhancement
|
||||
|
||||
```yaml
|
||||
{
|
||||
"backbone_type" : "nontransformer",
|
||||
"task": "enhancement",
|
||||
"n_classes" : 3,
|
||||
"n_epochs" : 4,
|
||||
"input_height" : 448,
|
||||
"input_width" : 224,
|
||||
"weight_decay" : 1e-6,
|
||||
"n_batch" : 4,
|
||||
"learning_rate": 1e-4,
|
||||
"patches" : true,
|
||||
"pretraining" : true,
|
||||
"augmentation" : true,
|
||||
"flip_aug" : false,
|
||||
"blur_aug" : false,
|
||||
"scaling" : true,
|
||||
"degrading": false,
|
||||
"brightening": false,
|
||||
"binarization" : false,
|
||||
"scaling_bluring" : false,
|
||||
"scaling_binarization" : false,
|
||||
"scaling_flip" : false,
|
||||
"rotation": false,
|
||||
"rotation_not_90": false,
|
||||
"blur_k" : ["blur","guass","median"],
|
||||
"scales" : [0.6, 0.7, 0.8, 0.9, 1.1, 1.2, 1.4],
|
||||
"brightness" : [1.3, 1.5, 1.7, 2],
|
||||
"degrade_scales" : [0.2, 0.4],
|
||||
"flip_index" : [0, 1, -1],
|
||||
"thetha" : [10, -10],
|
||||
"continue_training": false,
|
||||
"index_start" : 0,
|
||||
"dir_of_start_model" : " ",
|
||||
"weighted_loss": false,
|
||||
"is_loss_soft_dice": false,
|
||||
"data_is_provided": false,
|
||||
"dir_train": "./train",
|
||||
"dir_eval": "./eval",
|
||||
"dir_output": "./output"
|
||||
}
|
||||
```
|
||||
|
||||
It's important to mention that the value of n_classes for enhancement should be 3, as the model's output is a 3-channel
|
||||
image.
|
||||
|
||||
#### Page extraction
|
||||
|
||||
```yaml
|
||||
{
|
||||
"backbone_type" : "nontransformer",
|
||||
"task": "segmentation",
|
||||
"n_classes" : 2,
|
||||
"n_epochs" : 4,
|
||||
"input_height" : 448,
|
||||
"input_width" : 224,
|
||||
"weight_decay" : 1e-6,
|
||||
"n_batch" : 1,
|
||||
"learning_rate": 1e-4,
|
||||
"patches" : false,
|
||||
"pretraining" : true,
|
||||
"augmentation" : false,
|
||||
"flip_aug" : false,
|
||||
"blur_aug" : false,
|
||||
"scaling" : true,
|
||||
"degrading": false,
|
||||
"brightening": false,
|
||||
"binarization" : false,
|
||||
"scaling_bluring" : false,
|
||||
"scaling_binarization" : false,
|
||||
"scaling_flip" : false,
|
||||
"rotation": false,
|
||||
"rotation_not_90": false,
|
||||
"blur_k" : ["blur","guass","median"],
|
||||
"scales" : [0.6, 0.7, 0.8, 0.9, 1.1, 1.2, 1.4],
|
||||
"brightness" : [1.3, 1.5, 1.7, 2],
|
||||
"degrade_scales" : [0.2, 0.4],
|
||||
"flip_index" : [0, 1, -1],
|
||||
"thetha" : [10, -10],
|
||||
"continue_training": false,
|
||||
"index_start" : 0,
|
||||
"dir_of_start_model" : " ",
|
||||
"weighted_loss": false,
|
||||
"is_loss_soft_dice": false,
|
||||
"data_is_provided": false,
|
||||
"dir_train": "./train",
|
||||
"dir_eval": "./eval",
|
||||
"dir_output": "./output"
|
||||
}
|
||||
```
|
||||
|
||||
For page segmentation (or printspace or border segmentation), the model needs to view the input image in its entirety,
|
||||
hence the patches parameter should be set to false.
|
||||
|
||||
#### layout segmentation
|
||||
An example config json file for layout segmentation with 5 classes (including background) can be like this:
|
||||
|
||||
```yaml
|
||||
{
|
||||
"backbone_type" : "transformer",
|
||||
"task": "segmentation",
|
||||
"n_classes" : 5,
|
||||
"n_epochs" : 4,
|
||||
"input_height" : 448,
|
||||
"input_width" : 224,
|
||||
"weight_decay" : 1e-6,
|
||||
"n_batch" : 1,
|
||||
"learning_rate": 1e-4,
|
||||
"patches" : true,
|
||||
"pretraining" : true,
|
||||
"augmentation" : true,
|
||||
"flip_aug" : false,
|
||||
"blur_aug" : false,
|
||||
"scaling" : true,
|
||||
"degrading": false,
|
||||
"brightening": false,
|
||||
"binarization" : false,
|
||||
"scaling_bluring" : false,
|
||||
"scaling_binarization" : false,
|
||||
"scaling_flip" : false,
|
||||
"rotation": false,
|
||||
"rotation_not_90": false,
|
||||
"transformer_num_patches_xy": [7, 14],
|
||||
"transformer_patchsize_x": 1,
|
||||
"transformer_patchsize_y": 1,
|
||||
"transformer_projection_dim": 64,
|
||||
"transformer_mlp_head_units": [128, 64],
|
||||
"transformer_layers": 8,
|
||||
"transformer_num_heads": 4,
|
||||
"transformer_cnn_first": true,
|
||||
"blur_k" : ["blur","guass","median"],
|
||||
"scales" : [0.6, 0.7, 0.8, 0.9, 1.1, 1.2, 1.4],
|
||||
"brightness" : [1.3, 1.5, 1.7, 2],
|
||||
"degrade_scales" : [0.2, 0.4],
|
||||
"flip_index" : [0, 1, -1],
|
||||
"thetha" : [10, -10],
|
||||
"continue_training": false,
|
||||
"index_start" : 0,
|
||||
"dir_of_start_model" : " ",
|
||||
"weighted_loss": false,
|
||||
"is_loss_soft_dice": false,
|
||||
"data_is_provided": false,
|
||||
"dir_train": "./train",
|
||||
"dir_eval": "./eval",
|
||||
"dir_output": "./output"
|
||||
}
|
||||
```
|
||||
## Inference with the trained model
|
||||
|
||||
### classification
|
||||
For conducting inference with a trained model, you simply need to execute the following command line, specifying the
|
||||
directory of the model and the image on which to perform inference:
|
||||
|
||||
`python inference.py -m "model dir" -i "image" `
|
||||
|
||||
This will straightforwardly return the class of the image.
|
||||
|
||||
### machine based reading order
|
||||
To infer the reading order using a reading order model, we need a page XML file containing layout information but
|
||||
without the reading order. We simply need to provide the model directory, the XML file, and the output directory.
|
||||
The new XML file with the added reading order will be written to the output directory with the same name.
|
||||
We need to run:
|
||||
|
||||
`python inference.py -m "model dir" -xml "page xml file" -o "output dir to write new xml with reading order" `
|
||||
|
||||
### Segmentation (Textline, Binarization, Page extraction and layout) and enhancement
|
||||
For conducting inference with a trained model for segmentation and enhancement you need to run the following command
|
||||
line:
|
||||
|
||||
`python inference.py -m "model dir" -i "image" -p -s "output image" `
|
||||
|
||||
Note that in the case of page extraction the -p flag is not needed.
|
||||
|
||||
For segmentation or binarization tasks, if a ground truth (GT) label is available, the IoU evaluation metric can be
|
||||
calculated for the output. To do this, you need to provide the GT label using the argument -gt.
|
|
@ -1,92 +0,0 @@
|
|||
# Usage documentation
|
||||
The command-line interface can be called like this:
|
||||
|
||||
```sh
|
||||
eynollah \
|
||||
-i <single image file> | -di <directory containing image files> \
|
||||
-o <output directory> \
|
||||
-m <directory containing model files> \
|
||||
[OPTIONS]
|
||||
```
|
||||
|
||||
## Processing options
|
||||
The following options can be used to further configure the processing:
|
||||
|
||||
| option | description |
|
||||
|-------------------|:-------------------------------------------------------------------------------|
|
||||
| `-fl` | full layout analysis including all steps and segmentation classes |
|
||||
| `-light` | lighter and faster but simpler method for main region detection and deskewing |
|
||||
| `-tab` | apply table detection |
|
||||
| `-ae` | apply enhancement (the resulting image is saved to the output directory) |
|
||||
| `-as` | apply scaling |
|
||||
| `-cl` | apply contour detection for curved text lines instead of bounding boxes |
|
||||
| `-ib` | apply binarization (the resulting image is saved to the output directory) |
|
||||
| `-ep` | enable plotting (MUST always be used with `-sl`, `-sd`, `-sa`, `-si` or `-ae`) |
|
||||
| `-eoi` | extract only images to output directory (other processing will not be done) |
|
||||
| `-ho` | ignore headers for reading order dectection |
|
||||
| `-si <directory>` | save image regions detected to this directory |
|
||||
| `-sd <directory>` | save deskewed image to this directory |
|
||||
| `-sl <directory>` | save layout prediction as plot to this directory |
|
||||
| `-sp <directory>` | save cropped page image to this directory |
|
||||
| `-sa <directory>` | save all (plot, enhanced/binary image, layout) to this directory |
|
||||
|
||||
If no option is set, the tool performs detection of main regions (background, text, images, separators and marginals).
|
||||
|
||||
### `--full-layout` vs `--no-full-layout`
|
||||
|
||||
Here are the difference in elements detected depending on the `--full-layout`/`--no-full-layout` command line flags:
|
||||
|
||||
| | `--full-layout` | `--no-full-layout` |
|
||||
|--------------------------|-----------------|--------------------|
|
||||
| reading order | x | x |
|
||||
| header regions | x | - |
|
||||
| text regions | x | x |
|
||||
| text regions / text line | x | x |
|
||||
| drop-capitals | x | - |
|
||||
| marginals | x | x |
|
||||
| marginals / text line | x | x |
|
||||
| image region | x | x |
|
||||
|
||||
## Use as OCR-D processor
|
||||
Eynollah ships with a CLI interface to be used as [OCR-D](https://ocr-d.de) processor that is described in
|
||||
[`ocrd-tool.json`](https://github.com/qurator-spk/eynollah/tree/main/src/eynollah/ocrd-tool.json).
|
||||
|
||||
The source image file group with (preferably) RGB images should be used as input for Eynollah like this:
|
||||
|
||||
```
|
||||
ocrd-eynollah-segment -I OCR-D-IMG -O SEG-LINE -P models
|
||||
```
|
||||
|
||||
Any image referenced by `@imageFilename` in PAGE-XML is passed on directly to Eynollah as a processor, so that e.g.
|
||||
|
||||
```
|
||||
ocrd-eynollah-segment -I OCR-D-IMG-BIN -O SEG-LINE -P models
|
||||
```
|
||||
|
||||
uses the original (RGB) image despite any binarization that may have occured in previous OCR-D processing steps.
|
||||
|
||||
## Use with Docker
|
||||
TODO
|
||||
|
||||
## Hints
|
||||
* The best output quality is produced when RGB images are used as input rather than greyscale or binarized images.
|
||||
* If none of the parameters is set to `true`, the tool will perform a layout detection of main regions (background,
|
||||
text, images, separators and marginals). An advantage of this tool is that it tries to extract main text regions
|
||||
separately as much as possible.
|
||||
* If you set `-ae` (**a**llow image **e**nhancement) parameter to `true`, the tool will first check the ppi
|
||||
(pixel-per-inch) of the image and when it is less than 300, the tool will resize it and only then image enhancement will
|
||||
occur. Image enhancement can also take place without this option, but by setting this option to `true`, the layout xml
|
||||
data (e.g. coordinates) will be based on the resized and enhanced image instead of the original image.
|
||||
* For some documents, while the quality is good, their scale is very large, and the performance of tool decreases. In
|
||||
such cases you can set `-as` (**a**llow **s**caling) to `true`. With this option enabled, the tool will try to rescale
|
||||
the image and only then the layout detection process will begin.
|
||||
* If you care about drop capitals (initials) and headings, you can set `-fl` (**f**ull **l**ayout) to `true`. With this
|
||||
setting, the tool can currently distinguish 7 document layout classes/elements.
|
||||
* In cases where the document includes curved headers or curved lines, rectangular bounding boxes for textlines will not
|
||||
be a great option. In such cases it is strongly recommended setting the flag `-cl` (**c**urved **l**ines) to `true` to
|
||||
find contours of curved lines instead of rectangular bounding boxes. Be advised that enabling this option increases the
|
||||
processing time of the tool.
|
||||
* To crop and save image regions inside the document, set the parameter `-si` (**s**ave **i**mages) to true and provide
|
||||
a directory path to store the extracted images.
|
||||
* To extract only images from a document, set the parameter `-eoi` (**e**xtract **o**nly **i**mages). Choosing this
|
||||
option disables any other processing. To save the cropped images add `-ep` and `-si`.
|
|
@ -1 +1 @@
|
|||
src/eynollah/ocrd-tool.json
|
||||
qurator/eynollah/ocrd-tool.json
|
|
@ -3,6 +3,7 @@ requires = ["setuptools>=61.0", "wheel", "setuptools-ocrd"]
|
|||
|
||||
[project]
|
||||
name = "eynollah"
|
||||
version = "0.3.1"
|
||||
authors = [
|
||||
{name = "Vahid Rezanezhad"},
|
||||
{name = "Staatsbibliothek zu Berlin - Preußischer Kulturbesitz"},
|
||||
|
@ -13,7 +14,7 @@ license.file = "LICENSE"
|
|||
requires-python = ">=3.8"
|
||||
keywords = ["document layout analysis", "image segmentation"]
|
||||
|
||||
dynamic = ["dependencies", "version"]
|
||||
dynamic = ["dependencies"]
|
||||
|
||||
classifiers = [
|
||||
"Development Status :: 4 - Beta",
|
||||
|
@ -25,14 +26,9 @@ classifiers = [
|
|||
"Topic :: Scientific/Engineering :: Image Processing",
|
||||
]
|
||||
|
||||
[project.optional-dependencies]
|
||||
OCR = ["torch <= 2.0.1", "transformers <= 4.30.2"]
|
||||
plotting = ["matplotlib"]
|
||||
|
||||
[project.scripts]
|
||||
eynollah = "eynollah.cli:main"
|
||||
ocrd-eynollah-segment = "eynollah.ocrd_cli:main"
|
||||
ocrd-sbb-binarize = "eynollah.ocrd_cli_binarization:main"
|
||||
eynollah = "qurator.eynollah.cli:main"
|
||||
ocrd-eynollah-segment = "qurator.eynollah.ocrd_cli:main"
|
||||
|
||||
[project.urls]
|
||||
Homepage = "https://github.com/qurator-spk/eynollah"
|
||||
|
@ -40,14 +36,9 @@ Repository = "https://github.com/qurator-spk/eynollah.git"
|
|||
|
||||
[tool.setuptools.dynamic]
|
||||
dependencies = {file = ["requirements.txt"]}
|
||||
optional-dependencies.test = {file = ["requirements-test.txt"]}
|
||||
|
||||
[tool.setuptools.packages.find]
|
||||
where = ["src"]
|
||||
where = ["qurator"]
|
||||
|
||||
[tool.setuptools.package-data]
|
||||
"*" = ["*.json", '*.yml', '*.xml', '*.xsd']
|
||||
|
||||
[tool.coverage.run]
|
||||
branch = true
|
||||
source = ["eynollah"]
|
||||
|
|
0
qurator/eynollah/__init__.py
Normal file
0
qurator/eynollah/__init__.py
Normal file
208
qurator/eynollah/cli.py
Normal file
208
qurator/eynollah/cli.py
Normal file
|
@ -0,0 +1,208 @@
|
|||
import sys
|
||||
import click
|
||||
from ocrd_utils import initLogging, setOverrideLogLevel
|
||||
from qurator.eynollah.eynollah import Eynollah
|
||||
|
||||
|
||||
@click.command()
|
||||
@click.option(
|
||||
"--image",
|
||||
"-i",
|
||||
help="image filename",
|
||||
type=click.Path(exists=True, dir_okay=False),
|
||||
)
|
||||
@click.option(
|
||||
"--out",
|
||||
"-o",
|
||||
help="directory to write output xml data",
|
||||
type=click.Path(exists=True, file_okay=False),
|
||||
required=True,
|
||||
)
|
||||
@click.option(
|
||||
"--dir_in",
|
||||
"-di",
|
||||
help="directory of images",
|
||||
type=click.Path(exists=True, file_okay=False),
|
||||
)
|
||||
@click.option(
|
||||
"--model",
|
||||
"-m",
|
||||
help="directory of models",
|
||||
type=click.Path(exists=True, file_okay=False),
|
||||
required=True,
|
||||
)
|
||||
@click.option(
|
||||
"--save_images",
|
||||
"-si",
|
||||
help="if a directory is given, images in documents will be cropped and saved there",
|
||||
type=click.Path(exists=True, file_okay=False),
|
||||
)
|
||||
@click.option(
|
||||
"--save_layout",
|
||||
"-sl",
|
||||
help="if a directory is given, plot of layout will be saved there",
|
||||
type=click.Path(exists=True, file_okay=False),
|
||||
)
|
||||
@click.option(
|
||||
"--save_deskewed",
|
||||
"-sd",
|
||||
help="if a directory is given, deskewed image will be saved there",
|
||||
type=click.Path(exists=True, file_okay=False),
|
||||
)
|
||||
@click.option(
|
||||
"--save_all",
|
||||
"-sa",
|
||||
help="if a directory is given, all plots needed for documentation will be saved there",
|
||||
type=click.Path(exists=True, file_okay=False),
|
||||
)
|
||||
@click.option(
|
||||
"--save_page",
|
||||
"-sp",
|
||||
help="if a directory is given, page crop of image will be saved there",
|
||||
type=click.Path(exists=True, file_okay=False),
|
||||
)
|
||||
@click.option(
|
||||
"--enable-plotting/--disable-plotting",
|
||||
"-ep/-noep",
|
||||
is_flag=True,
|
||||
help="If set, will plot intermediary files and images",
|
||||
)
|
||||
@click.option(
|
||||
"--allow-enhancement/--no-allow-enhancement",
|
||||
"-ae/-noae",
|
||||
is_flag=True,
|
||||
help="if this parameter set to true, this tool would check that input image need resizing and enhancement or not. If so output of resized and enhanced image and corresponding layout data will be written in out directory",
|
||||
)
|
||||
@click.option(
|
||||
"--curved-line/--no-curvedline",
|
||||
"-cl/-nocl",
|
||||
is_flag=True,
|
||||
help="if this parameter set to true, this tool will try to return contoure of textlines instead of rectangle bounding box of textline. This should be taken into account that with this option the tool need more time to do process.",
|
||||
)
|
||||
@click.option(
|
||||
"--textline_light/--no-textline_light",
|
||||
"-tll/-notll",
|
||||
is_flag=True,
|
||||
help="if this parameter set to true, this tool will try to return contoure of textlines instead of rectangle bounding box of textline with a faster method.",
|
||||
)
|
||||
@click.option(
|
||||
"--full-layout/--no-full-layout",
|
||||
"-fl/-nofl",
|
||||
is_flag=True,
|
||||
help="if this parameter set to true, this tool will try to return all elements of layout.",
|
||||
)
|
||||
@click.option(
|
||||
"--tables/--no-tables",
|
||||
"-tab/-notab",
|
||||
is_flag=True,
|
||||
help="if this parameter set to true, this tool will try to detect tables.",
|
||||
)
|
||||
@click.option(
|
||||
"--right2left/--left2right",
|
||||
"-r2l/-l2r",
|
||||
is_flag=True,
|
||||
help="if this parameter set to true, this tool will extract right-to-left reading order.",
|
||||
)
|
||||
@click.option(
|
||||
"--input_binary/--input-RGB",
|
||||
"-ib/-irgb",
|
||||
is_flag=True,
|
||||
help="in general, eynollah uses RGB as input but if the input document is strongly dark, bright or for any other reason you can turn binarized input on. This option does not mean that you have to provide a binary image, otherwise this means that the tool itself will binarized the RGB input document.",
|
||||
)
|
||||
@click.option(
|
||||
"--allow_scaling/--no-allow-scaling",
|
||||
"-as/-noas",
|
||||
is_flag=True,
|
||||
help="if this parameter set to true, this tool would check the scale and if needed it will scale it to perform better layout detection",
|
||||
)
|
||||
@click.option(
|
||||
"--headers_off/--headers-on",
|
||||
"-ho/-noho",
|
||||
is_flag=True,
|
||||
help="if this parameter set to true, this tool would ignore headers role in reading order",
|
||||
)
|
||||
@click.option(
|
||||
"--light_version/--original",
|
||||
"-light/-org",
|
||||
is_flag=True,
|
||||
help="if this parameter set to true, this tool would use lighter version",
|
||||
)
|
||||
@click.option(
|
||||
"--ignore_page_extraction/--extract_page_included",
|
||||
"-ipe/-epi",
|
||||
is_flag=True,
|
||||
help="if this parameter set to true, this tool would ignore page extraction",
|
||||
)
|
||||
@click.option(
|
||||
"--log-level",
|
||||
"-l",
|
||||
type=click.Choice(['OFF', 'DEBUG', 'INFO', 'WARN', 'ERROR']),
|
||||
help="Override log level globally to this",
|
||||
)
|
||||
def main(
|
||||
image,
|
||||
out,
|
||||
dir_in,
|
||||
model,
|
||||
save_images,
|
||||
save_layout,
|
||||
save_deskewed,
|
||||
save_all,
|
||||
save_page,
|
||||
enable_plotting,
|
||||
allow_enhancement,
|
||||
curved_line,
|
||||
textline_light,
|
||||
full_layout,
|
||||
tables,
|
||||
right2left,
|
||||
input_binary,
|
||||
allow_scaling,
|
||||
headers_off,
|
||||
light_version,
|
||||
ignore_page_extraction,
|
||||
log_level
|
||||
):
|
||||
if log_level:
|
||||
setOverrideLogLevel(log_level)
|
||||
initLogging()
|
||||
if not enable_plotting and (save_layout or save_deskewed or save_all or save_page or save_images or allow_enhancement):
|
||||
print("Error: You used one of -sl, -sd, -sa, -sp, -si or -ae but did not enable plotting with -ep")
|
||||
sys.exit(1)
|
||||
elif enable_plotting and not (save_layout or save_deskewed or save_all or save_page or save_images or allow_enhancement):
|
||||
print("Error: You used -ep to enable plotting but set none of -sl, -sd, -sa, -sp, -si or -ae")
|
||||
sys.exit(1)
|
||||
if textline_light and not light_version:
|
||||
print('Error: You used -tll to enable light textline detection but -light is not enabled')
|
||||
sys.exit(1)
|
||||
eynollah = Eynollah(
|
||||
image_filename=image,
|
||||
dir_out=out,
|
||||
dir_in=dir_in,
|
||||
dir_models=model,
|
||||
dir_of_cropped_images=save_images,
|
||||
dir_of_layout=save_layout,
|
||||
dir_of_deskewed=save_deskewed,
|
||||
dir_of_all=save_all,
|
||||
dir_save_page=save_page,
|
||||
enable_plotting=enable_plotting,
|
||||
allow_enhancement=allow_enhancement,
|
||||
curved_line=curved_line,
|
||||
textline_light=textline_light,
|
||||
full_layout=full_layout,
|
||||
tables=tables,
|
||||
right2left=right2left,
|
||||
input_binary=input_binary,
|
||||
allow_scaling=allow_scaling,
|
||||
headers_off=headers_off,
|
||||
light_version=light_version,
|
||||
ignore_page_extraction=ignore_page_extraction,
|
||||
)
|
||||
if dir_in:
|
||||
eynollah.run()
|
||||
else:
|
||||
pcgts = eynollah.run()
|
||||
eynollah.writer.write_pagexml(pcgts)
|
||||
|
||||
if __name__ == "__main__":
|
||||
main()
|
3112
qurator/eynollah/eynollah.py
Normal file
3112
qurator/eynollah/eynollah.py
Normal file
File diff suppressed because it is too large
Load diff
65
qurator/eynollah/ocrd-tool.json
Normal file
65
qurator/eynollah/ocrd-tool.json
Normal file
|
@ -0,0 +1,65 @@
|
|||
{
|
||||
"version": "0.3.1",
|
||||
"git_url": "https://github.com/qurator-spk/eynollah",
|
||||
"tools": {
|
||||
"ocrd-eynollah-segment": {
|
||||
"executable": "ocrd-eynollah-segment",
|
||||
"categories": ["Layout analysis"],
|
||||
"description": "Segment page into regions and lines and do reading order detection with eynollah",
|
||||
"input_file_grp": ["OCR-D-IMG", "OCR-D-SEG-PAGE", "OCR-D-GT-SEG-PAGE"],
|
||||
"output_file_grp": ["OCR-D-SEG-LINE"],
|
||||
"steps": ["layout/segmentation/region", "layout/segmentation/line"],
|
||||
"parameters": {
|
||||
"models": {
|
||||
"type": "string",
|
||||
"format": "file",
|
||||
"content-type": "text/directory",
|
||||
"cacheable": true,
|
||||
"description": "Path to directory containing models to be used (See https://qurator-data.de/eynollah)",
|
||||
"required": true
|
||||
},
|
||||
"dpi": {
|
||||
"type": "number",
|
||||
"format": "float",
|
||||
"description": "pixel density in dots per inch (overrides any meta-data in the images); ignored if <= 0 (with fall-back 230)",
|
||||
"default": 0
|
||||
},
|
||||
"full_layout": {
|
||||
"type": "boolean",
|
||||
"default": true,
|
||||
"description": "Try to detect all element subtypes, including drop-caps and headings"
|
||||
},
|
||||
"tables": {
|
||||
"type": "boolean",
|
||||
"default": false,
|
||||
"description": "Try to detect table regions"
|
||||
},
|
||||
"curved_line": {
|
||||
"type": "boolean",
|
||||
"default": false,
|
||||
"description": "try to return contour of textlines instead of just rectangle bounding box. Needs more processing time"
|
||||
},
|
||||
"allow_scaling": {
|
||||
"type": "boolean",
|
||||
"default": false,
|
||||
"description": "check the resolution against the number of detected columns and if needed, scale the image up or down during layout detection (heuristic to improve quality and performance)"
|
||||
},
|
||||
"headers_off": {
|
||||
"type": "boolean",
|
||||
"default": false,
|
||||
"description": "ignore the special role of headings during reading order detection"
|
||||
}
|
||||
},
|
||||
"resources": [
|
||||
{
|
||||
"description": "models for eynollah (TensorFlow format)",
|
||||
"url": "https://github.com/qurator-spk/eynollah/releases/download/v0.3.0/models_eynollah.tar.gz",
|
||||
"name": "default",
|
||||
"size": 1761991295,
|
||||
"type": "archive",
|
||||
"path_in_archive": "models_eynollah"
|
||||
}
|
||||
]
|
||||
}
|
||||
}
|
||||
}
|
|
@ -1,8 +1,5 @@
|
|||
try:
|
||||
import matplotlib.pyplot as plt
|
||||
import matplotlib.patches as mpatches
|
||||
except ImportError:
|
||||
plt = mpatches = None
|
||||
import matplotlib.pyplot as plt
|
||||
import matplotlib.patches as mpatches
|
||||
import numpy as np
|
||||
import os.path
|
||||
import cv2
|
68
qurator/eynollah/processor.py
Normal file
68
qurator/eynollah/processor.py
Normal file
|
@ -0,0 +1,68 @@
|
|||
from json import loads
|
||||
from pkg_resources import resource_string
|
||||
from tempfile import NamedTemporaryFile
|
||||
from pathlib import Path
|
||||
from os.path import join
|
||||
|
||||
from PIL import Image
|
||||
|
||||
from ocrd import Processor
|
||||
from ocrd_modelfactory import page_from_file, exif_from_filename
|
||||
from ocrd_models import OcrdFile, OcrdExif
|
||||
from ocrd_models.ocrd_page import to_xml
|
||||
from ocrd_utils import (
|
||||
getLogger,
|
||||
MIMETYPE_PAGE,
|
||||
assert_file_grp_cardinality,
|
||||
make_file_id
|
||||
)
|
||||
|
||||
from .eynollah import Eynollah
|
||||
from .utils.pil_cv2 import pil2cv
|
||||
|
||||
OCRD_TOOL = loads(resource_string(__name__, 'ocrd-tool.json').decode('utf8'))
|
||||
|
||||
class EynollahProcessor(Processor):
|
||||
|
||||
def __init__(self, *args, **kwargs):
|
||||
kwargs['ocrd_tool'] = OCRD_TOOL['tools']['ocrd-eynollah-segment']
|
||||
kwargs['version'] = OCRD_TOOL['version']
|
||||
super().__init__(*args, **kwargs)
|
||||
|
||||
def process(self):
|
||||
LOG = getLogger('eynollah')
|
||||
assert_file_grp_cardinality(self.input_file_grp, 1)
|
||||
assert_file_grp_cardinality(self.output_file_grp, 1)
|
||||
for n, input_file in enumerate(self.input_files):
|
||||
page_id = input_file.pageId or input_file.ID
|
||||
LOG.info("INPUT FILE %s (%d/%d) ", page_id, n + 1, len(self.input_files))
|
||||
pcgts = page_from_file(self.workspace.download_file(input_file))
|
||||
LOG.debug('width %s height %s', pcgts.get_Page().imageWidth, pcgts.get_Page().imageHeight)
|
||||
self.add_metadata(pcgts)
|
||||
page = pcgts.get_Page()
|
||||
# XXX loses DPI information
|
||||
# page_image, _, _ = self.workspace.image_from_page(page, page_id, feature_filter='binarized')
|
||||
image_filename = self.workspace.download_file(next(self.workspace.mets.find_files(local_filename=page.imageFilename))).local_filename
|
||||
eynollah_kwargs = {
|
||||
'dir_models': self.resolve_resource(self.parameter['models']),
|
||||
'allow_enhancement': False,
|
||||
'curved_line': self.parameter['curved_line'],
|
||||
'full_layout': self.parameter['full_layout'],
|
||||
'allow_scaling': self.parameter['allow_scaling'],
|
||||
'headers_off': self.parameter['headers_off'],
|
||||
'tables': self.parameter['tables'],
|
||||
'override_dpi': self.parameter['dpi'],
|
||||
'logger': LOG,
|
||||
'pcgts': pcgts,
|
||||
'image_filename': image_filename
|
||||
}
|
||||
Eynollah(**eynollah_kwargs).run()
|
||||
file_id = make_file_id(input_file, self.output_file_grp)
|
||||
pcgts.set_pcGtsId(file_id)
|
||||
self.workspace.add_file(
|
||||
ID=file_id,
|
||||
file_grp=self.output_file_grp,
|
||||
pageId=page_id,
|
||||
mimetype=MIMETYPE_PAGE,
|
||||
local_filename=join(self.output_file_grp, file_id) + '.xml',
|
||||
content=to_xml(pcgts))
|
File diff suppressed because it is too large
Load diff
|
@ -1,10 +1,10 @@
|
|||
from functools import partial
|
||||
import cv2
|
||||
import numpy as np
|
||||
from shapely import geometry
|
||||
|
||||
from .rotate import rotate_image, rotation_image_new
|
||||
|
||||
from multiprocessing import Process, Queue, cpu_count
|
||||
from multiprocessing import Pool
|
||||
def contours_in_same_horizon(cy_main_hor):
|
||||
X1 = np.zeros((len(cy_main_hor), len(cy_main_hor)))
|
||||
X2 = np.zeros((len(cy_main_hor), len(cy_main_hor)))
|
||||
|
@ -27,33 +27,37 @@ def find_contours_mean_y_diff(contours_main):
|
|||
cy_main = [(M_main[j]["m01"] / (M_main[j]["m00"] + 1e-32)) for j in range(len(M_main))]
|
||||
return np.mean(np.diff(np.sort(np.array(cy_main))))
|
||||
|
||||
|
||||
def get_text_region_boxes_by_given_contours(contours):
|
||||
|
||||
kernel = np.ones((5, 5), np.uint8)
|
||||
boxes = []
|
||||
contours_new = []
|
||||
for jj in range(len(contours)):
|
||||
box = cv2.boundingRect(contours[jj])
|
||||
boxes.append(box)
|
||||
x, y, w, h = cv2.boundingRect(contours[jj])
|
||||
|
||||
boxes.append([x, y, w, h])
|
||||
contours_new.append(contours[jj])
|
||||
|
||||
del contours
|
||||
return boxes, contours_new
|
||||
|
||||
def filter_contours_area_of_image(image, contours, hierarchy, max_area, min_area):
|
||||
found_polygons_early = []
|
||||
found_polygons_early = list()
|
||||
|
||||
for jv,c in enumerate(contours):
|
||||
if len(c) < 3: # A polygon cannot have less than 3 points
|
||||
continue
|
||||
|
||||
polygon = geometry.Polygon([point[0] for point in c])
|
||||
area = polygon.area
|
||||
if (area >= min_area * np.prod(image.shape[:2]) and
|
||||
area <= max_area * np.prod(image.shape[:2]) and
|
||||
hierarchy[0][jv][3] == -1):
|
||||
found_polygons_early.append(np.array([[point]
|
||||
for point in polygon.exterior.coords], dtype=np.uint))
|
||||
if area >= min_area * np.prod(image.shape[:2]) and area <= max_area * np.prod(image.shape[:2]) and hierarchy[0][jv][3] == -1: # and hierarchy[0][jv][3]==-1 :
|
||||
found_polygons_early.append(np.array([[point] for point in polygon.exterior.coords], dtype=np.uint))
|
||||
return found_polygons_early
|
||||
|
||||
def filter_contours_area_of_image_tables(image, contours, hierarchy, max_area, min_area):
|
||||
found_polygons_early = []
|
||||
found_polygons_early = list()
|
||||
|
||||
for jv,c in enumerate(contours):
|
||||
if len(c) < 3: # A polygon cannot have less than 3 points
|
||||
continue
|
||||
|
@ -64,59 +68,48 @@ def filter_contours_area_of_image_tables(image, contours, hierarchy, max_area, m
|
|||
##print(np.prod(thresh.shape[:2]))
|
||||
# Check that polygon has area greater than minimal area
|
||||
# print(hierarchy[0][jv][3],hierarchy )
|
||||
if (area >= min_area * np.prod(image.shape[:2]) and
|
||||
area <= max_area * np.prod(image.shape[:2]) and
|
||||
# hierarchy[0][jv][3]==-1
|
||||
True):
|
||||
if area >= min_area * np.prod(image.shape[:2]) and area <= max_area * np.prod(image.shape[:2]): # and hierarchy[0][jv][3]==-1 :
|
||||
# print(c[0][0][1])
|
||||
found_polygons_early.append(np.array([[point]
|
||||
for point in polygon.exterior.coords], dtype=np.int32))
|
||||
found_polygons_early.append(np.array([[point] for point in polygon.exterior.coords], dtype=np.int32))
|
||||
return found_polygons_early
|
||||
|
||||
def find_new_features_of_contours(contours_main):
|
||||
areas_main = np.array([cv2.contourArea(contours_main[j])
|
||||
for j in range(len(contours_main))])
|
||||
M_main = [cv2.moments(contours_main[j])
|
||||
for j in range(len(contours_main))]
|
||||
cx_main = [(M_main[j]["m10"] / (M_main[j]["m00"] + 1e-32))
|
||||
for j in range(len(M_main))]
|
||||
cy_main = [(M_main[j]["m01"] / (M_main[j]["m00"] + 1e-32))
|
||||
for j in range(len(M_main))]
|
||||
|
||||
areas_main = np.array([cv2.contourArea(contours_main[j]) for j in range(len(contours_main))])
|
||||
M_main = [cv2.moments(contours_main[j]) for j in range(len(contours_main))]
|
||||
cx_main = [(M_main[j]["m10"] / (M_main[j]["m00"] + 1e-32)) for j in range(len(M_main))]
|
||||
cy_main = [(M_main[j]["m01"] / (M_main[j]["m00"] + 1e-32)) for j in range(len(M_main))]
|
||||
try:
|
||||
x_min_main = np.array([np.min(contours_main[j][:, 0, 0])
|
||||
for j in range(len(contours_main))])
|
||||
argmin_x_main = np.array([np.argmin(contours_main[j][:, 0, 0])
|
||||
for j in range(len(contours_main))])
|
||||
x_min_from_argmin = np.array([contours_main[j][argmin_x_main[j], 0, 0]
|
||||
for j in range(len(contours_main))])
|
||||
y_corr_x_min_from_argmin = np.array([contours_main[j][argmin_x_main[j], 0, 1]
|
||||
for j in range(len(contours_main))])
|
||||
x_max_main = np.array([np.max(contours_main[j][:, 0, 0])
|
||||
for j in range(len(contours_main))])
|
||||
y_min_main = np.array([np.min(contours_main[j][:, 0, 1])
|
||||
for j in range(len(contours_main))])
|
||||
y_max_main = np.array([np.max(contours_main[j][:, 0, 1])
|
||||
for j in range(len(contours_main))])
|
||||
x_min_main = np.array([np.min(contours_main[j][:, 0, 0]) for j in range(len(contours_main))])
|
||||
|
||||
argmin_x_main = np.array([np.argmin(contours_main[j][:, 0, 0]) for j in range(len(contours_main))])
|
||||
|
||||
x_min_from_argmin = np.array([contours_main[j][argmin_x_main[j], 0, 0] for j in range(len(contours_main))])
|
||||
y_corr_x_min_from_argmin = np.array([contours_main[j][argmin_x_main[j], 0, 1] for j in range(len(contours_main))])
|
||||
|
||||
x_max_main = np.array([np.max(contours_main[j][:, 0, 0]) for j in range(len(contours_main))])
|
||||
|
||||
y_min_main = np.array([np.min(contours_main[j][:, 0, 1]) for j in range(len(contours_main))])
|
||||
y_max_main = np.array([np.max(contours_main[j][:, 0, 1]) for j in range(len(contours_main))])
|
||||
except:
|
||||
x_min_main = np.array([np.min(contours_main[j][:, 0])
|
||||
for j in range(len(contours_main))])
|
||||
argmin_x_main = np.array([np.argmin(contours_main[j][:, 0])
|
||||
for j in range(len(contours_main))])
|
||||
x_min_from_argmin = np.array([contours_main[j][argmin_x_main[j], 0]
|
||||
for j in range(len(contours_main))])
|
||||
y_corr_x_min_from_argmin = np.array([contours_main[j][argmin_x_main[j], 1]
|
||||
for j in range(len(contours_main))])
|
||||
x_max_main = np.array([np.max(contours_main[j][:, 0])
|
||||
for j in range(len(contours_main))])
|
||||
y_min_main = np.array([np.min(contours_main[j][:, 1])
|
||||
for j in range(len(contours_main))])
|
||||
y_max_main = np.array([np.max(contours_main[j][:, 1])
|
||||
for j in range(len(contours_main))])
|
||||
x_min_main = np.array([np.min(contours_main[j][:, 0]) for j in range(len(contours_main))])
|
||||
|
||||
argmin_x_main = np.array([np.argmin(contours_main[j][:, 0]) for j in range(len(contours_main))])
|
||||
|
||||
x_min_from_argmin = np.array([contours_main[j][argmin_x_main[j], 0] for j in range(len(contours_main))])
|
||||
y_corr_x_min_from_argmin = np.array([contours_main[j][argmin_x_main[j], 1] for j in range(len(contours_main))])
|
||||
|
||||
x_max_main = np.array([np.max(contours_main[j][:, 0]) for j in range(len(contours_main))])
|
||||
|
||||
y_min_main = np.array([np.min(contours_main[j][:, 1]) for j in range(len(contours_main))])
|
||||
y_max_main = np.array([np.max(contours_main[j][:, 1]) for j in range(len(contours_main))])
|
||||
|
||||
# dis_x=np.abs(x_max_main-x_min_main)
|
||||
|
||||
return cx_main, cy_main, x_min_main, x_max_main, y_min_main, y_max_main, y_corr_x_min_from_argmin
|
||||
|
||||
def find_features_of_contours(contours_main):
|
||||
|
||||
|
||||
areas_main=np.array([cv2.contourArea(contours_main[j]) for j in range(len(contours_main))])
|
||||
M_main=[cv2.moments(contours_main[j]) for j in range(len(contours_main))]
|
||||
cx_main=[(M_main[j]['m10']/(M_main[j]['m00']+1e-32)) for j in range(len(M_main))]
|
||||
|
@ -127,15 +120,14 @@ def find_features_of_contours(contours_main):
|
|||
y_min_main=np.array([np.min(contours_main[j][:,0,1]) for j in range(len(contours_main))])
|
||||
y_max_main=np.array([np.max(contours_main[j][:,0,1]) for j in range(len(contours_main))])
|
||||
|
||||
|
||||
return y_min_main, y_max_main
|
||||
|
||||
def return_parent_contours(contours, hierarchy):
|
||||
contours_parent = [contours[i]
|
||||
for i in range(len(contours))
|
||||
if hierarchy[0][i][3] == -1]
|
||||
contours_parent = [contours[i] for i in range(len(contours)) if hierarchy[0][i][3] == -1]
|
||||
return contours_parent
|
||||
|
||||
def return_contours_of_interested_region(region_pre_p, pixel, min_area=0.0002):
|
||||
|
||||
# pixels of images are identified by 5
|
||||
if len(region_pre_p.shape) == 3:
|
||||
cnts_images = (region_pre_p[:, :, 0] == pixel) * 1
|
||||
|
@ -147,16 +139,80 @@ def return_contours_of_interested_region(region_pre_p, pixel, min_area=0.0002):
|
|||
ret, thresh = cv2.threshold(imgray, 0, 255, 0)
|
||||
|
||||
contours_imgs, hierarchy = cv2.findContours(thresh, cv2.RETR_TREE, cv2.CHAIN_APPROX_SIMPLE)
|
||||
|
||||
contours_imgs = return_parent_contours(contours_imgs, hierarchy)
|
||||
contours_imgs = filter_contours_area_of_image_tables(thresh, contours_imgs, hierarchy,
|
||||
max_area=1, min_area=min_area)
|
||||
contours_imgs = filter_contours_area_of_image_tables(thresh, contours_imgs, hierarchy, max_area=1, min_area=min_area)
|
||||
|
||||
return contours_imgs
|
||||
|
||||
def do_work_of_contours_in_image(contour, index_r_con, img, slope_first):
|
||||
img_copy = np.zeros(img.shape)
|
||||
img_copy = cv2.fillPoly(img_copy, pts=[contour], color=(1, 1, 1))
|
||||
def do_work_of_contours_in_image(queue_of_all_params, contours_per_process, indexes_r_con_per_pro, img, slope_first):
|
||||
cnts_org_per_each_subprocess = []
|
||||
index_by_text_region_contours = []
|
||||
for mv in range(len(contours_per_process)):
|
||||
index_by_text_region_contours.append(indexes_r_con_per_pro[mv])
|
||||
|
||||
img_copy = np.zeros(img.shape)
|
||||
img_copy = cv2.fillPoly(img_copy, pts=[contours_per_process[mv]], color=(1, 1, 1))
|
||||
|
||||
img_copy = rotation_image_new(img_copy, -slope_first)
|
||||
|
||||
img_copy = img_copy.astype(np.uint8)
|
||||
imgray = cv2.cvtColor(img_copy, cv2.COLOR_BGR2GRAY)
|
||||
ret, thresh = cv2.threshold(imgray, 0, 255, 0)
|
||||
|
||||
cont_int, _ = cv2.findContours(thresh, cv2.RETR_TREE, cv2.CHAIN_APPROX_SIMPLE)
|
||||
|
||||
cont_int[0][:, 0, 0] = cont_int[0][:, 0, 0] + np.abs(img_copy.shape[1] - img.shape[1])
|
||||
cont_int[0][:, 0, 1] = cont_int[0][:, 0, 1] + np.abs(img_copy.shape[0] - img.shape[0])
|
||||
|
||||
|
||||
cnts_org_per_each_subprocess.append(cont_int[0])
|
||||
|
||||
queue_of_all_params.put([ cnts_org_per_each_subprocess, index_by_text_region_contours])
|
||||
|
||||
|
||||
def get_textregion_contours_in_org_image_multi(cnts, img, slope_first):
|
||||
|
||||
num_cores = cpu_count()
|
||||
queue_of_all_params = Queue()
|
||||
|
||||
processes = []
|
||||
nh = np.linspace(0, len(cnts), num_cores + 1)
|
||||
indexes_by_text_con = np.array(range(len(cnts)))
|
||||
for i in range(num_cores):
|
||||
contours_per_process = cnts[int(nh[i]) : int(nh[i + 1])]
|
||||
indexes_text_con_per_process = indexes_by_text_con[int(nh[i]) : int(nh[i + 1])]
|
||||
|
||||
processes.append(Process(target=do_work_of_contours_in_image, args=(queue_of_all_params, contours_per_process, indexes_text_con_per_process, img,slope_first )))
|
||||
for i in range(num_cores):
|
||||
processes[i].start()
|
||||
cnts_org = []
|
||||
all_index_text_con = []
|
||||
for i in range(num_cores):
|
||||
list_all_par = queue_of_all_params.get(True)
|
||||
contours_for_sub_process = list_all_par[0]
|
||||
indexes_for_sub_process = list_all_par[1]
|
||||
for j in range(len(contours_for_sub_process)):
|
||||
cnts_org.append(contours_for_sub_process[j])
|
||||
all_index_text_con.append(indexes_for_sub_process[j])
|
||||
for i in range(num_cores):
|
||||
processes[i].join()
|
||||
|
||||
print(all_index_text_con)
|
||||
return cnts_org
|
||||
def loop_contour_image(index_l, cnts,img, slope_first):
|
||||
img_copy = np.zeros(img.shape)
|
||||
img_copy = cv2.fillPoly(img_copy, pts=[cnts[index_l]], color=(1, 1, 1))
|
||||
|
||||
# plt.imshow(img_copy)
|
||||
# plt.show()
|
||||
|
||||
# print(img.shape,'img')
|
||||
img_copy = rotation_image_new(img_copy, -slope_first)
|
||||
##print(img_copy.shape,'img_copy')
|
||||
# plt.imshow(img_copy)
|
||||
# plt.show()
|
||||
|
||||
img_copy = img_copy.astype(np.uint8)
|
||||
imgray = cv2.cvtColor(img_copy, cv2.COLOR_BGR2GRAY)
|
||||
ret, thresh = cv2.threshold(imgray, 0, 255, 0)
|
||||
|
@ -165,20 +221,20 @@ def do_work_of_contours_in_image(contour, index_r_con, img, slope_first):
|
|||
|
||||
cont_int[0][:, 0, 0] = cont_int[0][:, 0, 0] + np.abs(img_copy.shape[1] - img.shape[1])
|
||||
cont_int[0][:, 0, 1] = cont_int[0][:, 0, 1] + np.abs(img_copy.shape[0] - img.shape[0])
|
||||
# print(np.shape(cont_int[0]))
|
||||
return cont_int[0]
|
||||
|
||||
return cont_int[0], index_r_con
|
||||
def get_textregion_contours_in_org_image_multi2(cnts, img, slope_first):
|
||||
|
||||
def get_textregion_contours_in_org_image_multi(cnts, img, slope_first, map=map):
|
||||
if not len(cnts):
|
||||
return [], []
|
||||
results = map(partial(do_work_of_contours_in_image,
|
||||
img=img,
|
||||
slope_first=slope_first,
|
||||
),
|
||||
cnts, range(len(cnts)))
|
||||
return tuple(zip(*results))
|
||||
cnts_org = []
|
||||
# print(cnts,'cnts')
|
||||
with Pool(cpu_count()) as p:
|
||||
cnts_org = p.starmap(loop_contour_image, [(index_l,cnts, img,slope_first) for index_l in range(len(cnts))])
|
||||
|
||||
return cnts_org
|
||||
|
||||
def get_textregion_contours_in_org_image(cnts, img, slope_first):
|
||||
|
||||
cnts_org = []
|
||||
# print(cnts,'cnts')
|
||||
for i in range(len(cnts)):
|
||||
|
@ -199,6 +255,7 @@ def get_textregion_contours_in_org_image(cnts, img, slope_first):
|
|||
ret, thresh = cv2.threshold(imgray, 0, 255, 0)
|
||||
|
||||
cont_int, _ = cv2.findContours(thresh, cv2.RETR_TREE, cv2.CHAIN_APPROX_SIMPLE)
|
||||
|
||||
cont_int[0][:, 0, 0] = cont_int[0][:, 0, 0] + np.abs(img_copy.shape[1] - img.shape[1])
|
||||
cont_int[0][:, 0, 1] = cont_int[0][:, 0, 1] + np.abs(img_copy.shape[0] - img.shape[0])
|
||||
# print(np.shape(cont_int[0]))
|
||||
|
@ -206,66 +263,45 @@ def get_textregion_contours_in_org_image(cnts, img, slope_first):
|
|||
|
||||
return cnts_org
|
||||
|
||||
def get_textregion_contours_in_org_image_light_old(cnts, img, slope_first):
|
||||
zoom = 3
|
||||
img = cv2.resize(img, (img.shape[1] // zoom,
|
||||
img.shape[0] // zoom),
|
||||
interpolation=cv2.INTER_NEAREST)
|
||||
def get_textregion_contours_in_org_image_light(cnts, img, slope_first):
|
||||
|
||||
h_o = img.shape[0]
|
||||
w_o = img.shape[1]
|
||||
|
||||
img = cv2.resize(img, (int(img.shape[1]/3.), int(img.shape[0]/3.)), interpolation=cv2.INTER_NEAREST)
|
||||
##cnts = list( (np.array(cnts)/2).astype(np.int16) )
|
||||
#cnts = cnts/2
|
||||
cnts = [(i/ 3).astype(np.int32) for i in cnts]
|
||||
cnts_org = []
|
||||
for cnt in cnts:
|
||||
#print(cnts,'cnts')
|
||||
for i in range(len(cnts)):
|
||||
img_copy = np.zeros(img.shape)
|
||||
img_copy = cv2.fillPoly(img_copy, pts=[(cnt / zoom).astype(int)], color=(1, 1, 1))
|
||||
img_copy = cv2.fillPoly(img_copy, pts=[cnts[i]], color=(1, 1, 1))
|
||||
|
||||
img_copy = rotation_image_new(img_copy, -slope_first).astype(np.uint8)
|
||||
# plt.imshow(img_copy)
|
||||
# plt.show()
|
||||
|
||||
# print(img.shape,'img')
|
||||
img_copy = rotation_image_new(img_copy, -slope_first)
|
||||
##print(img_copy.shape,'img_copy')
|
||||
# plt.imshow(img_copy)
|
||||
# plt.show()
|
||||
|
||||
img_copy = img_copy.astype(np.uint8)
|
||||
imgray = cv2.cvtColor(img_copy, cv2.COLOR_BGR2GRAY)
|
||||
ret, thresh = cv2.threshold(imgray, 0, 255, 0)
|
||||
|
||||
cont_int, _ = cv2.findContours(thresh, cv2.RETR_TREE, cv2.CHAIN_APPROX_SIMPLE)
|
||||
|
||||
cont_int[0][:, 0, 0] = cont_int[0][:, 0, 0] + np.abs(img_copy.shape[1] - img.shape[1])
|
||||
cont_int[0][:, 0, 1] = cont_int[0][:, 0, 1] + np.abs(img_copy.shape[0] - img.shape[0])
|
||||
cnts_org.append(cont_int[0] * zoom)
|
||||
# print(np.shape(cont_int[0]))
|
||||
cnts_org.append(cont_int[0]*3)
|
||||
|
||||
return cnts_org
|
||||
|
||||
def do_back_rotation_and_get_cnt_back(contour_par, index_r_con, img, slope_first, confidence_matrix):
|
||||
img_copy = np.zeros(img.shape)
|
||||
img_copy = cv2.fillPoly(img_copy, pts=[contour_par], color=(1, 1, 1))
|
||||
confidence_matrix_mapped_with_contour = confidence_matrix * img_copy[:,:,0]
|
||||
confidence_contour = np.sum(confidence_matrix_mapped_with_contour) / float(np.sum(img_copy[:,:,0]))
|
||||
|
||||
img_copy = rotation_image_new(img_copy, -slope_first).astype(np.uint8)
|
||||
imgray = cv2.cvtColor(img_copy, cv2.COLOR_BGR2GRAY)
|
||||
ret, thresh = cv2.threshold(imgray, 0, 255, 0)
|
||||
|
||||
cont_int, _ = cv2.findContours(thresh, cv2.RETR_TREE, cv2.CHAIN_APPROX_SIMPLE)
|
||||
if len(cont_int)==0:
|
||||
cont_int = []
|
||||
cont_int.append(contour_par)
|
||||
confidence_contour = 0
|
||||
else:
|
||||
cont_int[0][:, 0, 0] = cont_int[0][:, 0, 0] + np.abs(img_copy.shape[1] - img.shape[1])
|
||||
cont_int[0][:, 0, 1] = cont_int[0][:, 0, 1] + np.abs(img_copy.shape[0] - img.shape[0])
|
||||
return cont_int[0], index_r_con, confidence_contour
|
||||
|
||||
def get_textregion_contours_in_org_image_light(cnts, img, slope_first, confidence_matrix, map=map):
|
||||
if not len(cnts):
|
||||
return [], []
|
||||
|
||||
confidence_matrix = cv2.resize(confidence_matrix, (int(img.shape[1]/6), int(img.shape[0]/6)), interpolation=cv2.INTER_NEAREST)
|
||||
img = cv2.resize(img, (int(img.shape[1]/6), int(img.shape[0]/6)), interpolation=cv2.INTER_NEAREST)
|
||||
##cnts = list( (np.array(cnts)/2).astype(np.int16) )
|
||||
#cnts = cnts/2
|
||||
cnts = [(i/6).astype(int) for i in cnts]
|
||||
results = map(partial(do_back_rotation_and_get_cnt_back,
|
||||
img=img,
|
||||
slope_first=slope_first,
|
||||
confidence_matrix=confidence_matrix,
|
||||
),
|
||||
cnts, range(len(cnts)))
|
||||
contours, indexes, conf_contours = tuple(zip(*results))
|
||||
return [i*6 for i in contours], list(conf_contours)
|
||||
|
||||
def return_contours_of_interested_textline(region_pre_p, pixel):
|
||||
|
||||
# pixels of images are identified by 5
|
||||
if len(region_pre_p.shape) == 3:
|
||||
cnts_images = (region_pre_p[:, :, 0] == pixel) * 1
|
||||
|
@ -278,11 +314,11 @@ def return_contours_of_interested_textline(region_pre_p, pixel):
|
|||
contours_imgs, hierarchy = cv2.findContours(thresh, cv2.RETR_TREE, cv2.CHAIN_APPROX_SIMPLE)
|
||||
|
||||
contours_imgs = return_parent_contours(contours_imgs, hierarchy)
|
||||
contours_imgs = filter_contours_area_of_image_tables(
|
||||
thresh, contours_imgs, hierarchy, max_area=1, min_area=0.000000003)
|
||||
contours_imgs = filter_contours_area_of_image_tables(thresh, contours_imgs, hierarchy, max_area=1, min_area=0.000000003)
|
||||
return contours_imgs
|
||||
|
||||
def return_contours_of_image(image):
|
||||
|
||||
if len(image.shape) == 2:
|
||||
image = np.repeat(image[:, :, np.newaxis], 3, axis=2)
|
||||
image = image.astype(np.uint8)
|
||||
|
@ -294,6 +330,7 @@ def return_contours_of_image(image):
|
|||
return contours, hierarchy
|
||||
|
||||
def return_contours_of_interested_region_by_min_size(region_pre_p, pixel, min_size=0.00003):
|
||||
|
||||
# pixels of images are identified by 5
|
||||
if len(region_pre_p.shape) == 3:
|
||||
cnts_images = (region_pre_p[:, :, 0] == pixel) * 1
|
||||
|
@ -305,13 +342,14 @@ def return_contours_of_interested_region_by_min_size(region_pre_p, pixel, min_si
|
|||
ret, thresh = cv2.threshold(imgray, 0, 255, 0)
|
||||
|
||||
contours_imgs, hierarchy = cv2.findContours(thresh, cv2.RETR_TREE, cv2.CHAIN_APPROX_SIMPLE)
|
||||
|
||||
contours_imgs = return_parent_contours(contours_imgs, hierarchy)
|
||||
contours_imgs = filter_contours_area_of_image_tables(
|
||||
thresh, contours_imgs, hierarchy, max_area=1, min_area=min_size)
|
||||
contours_imgs = filter_contours_area_of_image_tables(thresh, contours_imgs, hierarchy, max_area=1, min_area=min_size)
|
||||
|
||||
return contours_imgs
|
||||
|
||||
def return_contours_of_interested_region_by_size(region_pre_p, pixel, min_area, max_area):
|
||||
|
||||
# pixels of images are identified by 5
|
||||
if len(region_pre_p.shape) == 3:
|
||||
cnts_images = (region_pre_p[:, :, 0] == pixel) * 1
|
||||
|
@ -324,11 +362,9 @@ def return_contours_of_interested_region_by_size(region_pre_p, pixel, min_area,
|
|||
contours_imgs, hierarchy = cv2.findContours(thresh, cv2.RETR_TREE, cv2.CHAIN_APPROX_SIMPLE)
|
||||
|
||||
contours_imgs = return_parent_contours(contours_imgs, hierarchy)
|
||||
contours_imgs = filter_contours_area_of_image_tables(
|
||||
thresh, contours_imgs, hierarchy, max_area=max_area, min_area=min_area)
|
||||
contours_imgs = filter_contours_area_of_image_tables(thresh, contours_imgs, hierarchy, max_area=max_area, min_area=min_area)
|
||||
|
||||
img_ret = np.zeros((region_pre_p.shape[0], region_pre_p.shape[1], 3))
|
||||
img_ret = cv2.fillPoly(img_ret, pts=contours_imgs, color=(1, 1, 1))
|
||||
|
||||
return img_ret[:, :, 0]
|
||||
|
|
@ -4,7 +4,6 @@ from .contour import (
|
|||
find_new_features_of_contours,
|
||||
return_contours_of_image,
|
||||
return_parent_contours,
|
||||
return_contours_of_interested_region,
|
||||
)
|
||||
|
||||
def adhere_drop_capital_region_into_corresponding_textline(
|
||||
|
@ -18,7 +17,6 @@ def adhere_drop_capital_region_into_corresponding_textline(
|
|||
all_found_textline_polygons_h,
|
||||
kernel=None,
|
||||
curved_line=False,
|
||||
textline_light=False,
|
||||
):
|
||||
# print(np.shape(all_found_textline_polygons),np.shape(all_found_textline_polygons[3]),'all_found_textline_polygonsshape')
|
||||
# print(all_found_textline_polygons[3])
|
||||
|
@ -78,7 +76,7 @@ def adhere_drop_capital_region_into_corresponding_textline(
|
|||
# region_with_intersected_drop=region_with_intersected_drop/3
|
||||
region_with_intersected_drop = region_with_intersected_drop.astype(np.uint8)
|
||||
# print(np.unique(img_con_all_copy[:,:,0]))
|
||||
if curved_line or textline_light:
|
||||
if curved_line:
|
||||
|
||||
if len(region_with_intersected_drop) > 1:
|
||||
sum_pixels_of_intersection = []
|
||||
|
@ -116,17 +114,12 @@ def adhere_drop_capital_region_into_corresponding_textline(
|
|||
img_textlines = cv2.fillPoly(img_textlines, pts=[polygons_of_drop_capitals[i_drop]], color=(255, 255, 255))
|
||||
|
||||
img_textlines = img_textlines.astype(np.uint8)
|
||||
|
||||
contours_combined = return_contours_of_interested_region(img_textlines, 255, 0)
|
||||
|
||||
#plt.imshow(img_textlines)
|
||||
#plt.show()
|
||||
|
||||
#imgray = cv2.cvtColor(img_textlines, cv2.COLOR_BGR2GRAY)
|
||||
#ret, thresh = cv2.threshold(imgray, 0, 255, 0)
|
||||
imgray = cv2.cvtColor(img_textlines, cv2.COLOR_BGR2GRAY)
|
||||
ret, thresh = cv2.threshold(imgray, 0, 255, 0)
|
||||
|
||||
#contours_combined, hierarchy = cv2.findContours(thresh, cv2.RETR_TREE, cv2.CHAIN_APPROX_SIMPLE)
|
||||
contours_combined, hierarchy = cv2.findContours(thresh, cv2.RETR_TREE, cv2.CHAIN_APPROX_SIMPLE)
|
||||
|
||||
# print(len(contours_combined),'len textlines mixed')
|
||||
areas_cnt_text = np.array([cv2.contourArea(contours_combined[j]) for j in range(len(contours_combined))])
|
||||
|
||||
contours_biggest = contours_combined[np.argmax(areas_cnt_text)]
|
||||
|
@ -137,13 +130,8 @@ def adhere_drop_capital_region_into_corresponding_textline(
|
|||
# contours_biggest[:,0,1]=contours_biggest[:,0,1]#-all_box_coord[int(region_final)][0]
|
||||
|
||||
# contours_biggest=contours_biggest.reshape(np.shape(contours_biggest)[0],np.shape(contours_biggest)[2])
|
||||
|
||||
if len(contours_combined)==1:
|
||||
all_found_textline_polygons[int(region_final)][arg_min] = contours_biggest
|
||||
elif len(contours_combined)==2:
|
||||
all_found_textline_polygons[int(region_final)].insert(arg_min, polygons_of_drop_capitals[i_drop] )
|
||||
else:
|
||||
pass
|
||||
|
||||
all_found_textline_polygons[int(region_final)][arg_min] = contours_biggest
|
||||
|
||||
except:
|
||||
# print('gordun1')
|
||||
|
@ -179,13 +167,14 @@ def adhere_drop_capital_region_into_corresponding_textline(
|
|||
|
||||
img_textlines = img_textlines.astype(np.uint8)
|
||||
|
||||
|
||||
contours_combined = return_contours_of_interested_region(img_textlines, 255, 0)
|
||||
##imgray = cv2.cvtColor(img_textlines, cv2.COLOR_BGR2GRAY)
|
||||
##ret, thresh = cv2.threshold(imgray, 0, 255, 0)
|
||||
# plt.imshow(img_textlines)
|
||||
# plt.show()
|
||||
imgray = cv2.cvtColor(img_textlines, cv2.COLOR_BGR2GRAY)
|
||||
ret, thresh = cv2.threshold(imgray, 0, 255, 0)
|
||||
|
||||
##contours_combined, hierarchy = cv2.findContours(thresh, cv2.RETR_TREE, cv2.CHAIN_APPROX_SIMPLE)
|
||||
contours_combined, hierarchy = cv2.findContours(thresh, cv2.RETR_TREE, cv2.CHAIN_APPROX_SIMPLE)
|
||||
|
||||
# print(len(contours_combined),'len textlines mixed')
|
||||
areas_cnt_text = np.array([cv2.contourArea(contours_combined[j]) for j in range(len(contours_combined))])
|
||||
|
||||
contours_biggest = contours_combined[np.argmax(areas_cnt_text)]
|
||||
|
@ -197,12 +186,7 @@ def adhere_drop_capital_region_into_corresponding_textline(
|
|||
# print(np.shape(contours_biggest),'contours_biggest')
|
||||
# print(np.shape(all_found_textline_polygons[int(region_final)][arg_min]))
|
||||
##contours_biggest=contours_biggest.reshape(np.shape(contours_biggest)[0],np.shape(contours_biggest)[2])
|
||||
if len(contours_combined)==1:
|
||||
all_found_textline_polygons[int(region_final)][arg_min] = contours_biggest
|
||||
elif len(contours_combined)==2:
|
||||
all_found_textline_polygons[int(region_final)].insert(arg_min, polygons_of_drop_capitals[i_drop] )
|
||||
else:
|
||||
pass
|
||||
all_found_textline_polygons[int(region_final)][arg_min] = contours_biggest
|
||||
except:
|
||||
pass
|
||||
|
||||
|
@ -231,11 +215,10 @@ def adhere_drop_capital_region_into_corresponding_textline(
|
|||
img_textlines = cv2.fillPoly(img_textlines, pts=[polygons_of_drop_capitals[i_drop]], color=(255, 255, 255))
|
||||
|
||||
img_textlines = img_textlines.astype(np.uint8)
|
||||
contours_combined = return_contours_of_interested_region(img_textlines, 255, 0)
|
||||
#imgray = cv2.cvtColor(img_textlines, cv2.COLOR_BGR2GRAY)
|
||||
#ret, thresh = cv2.threshold(imgray, 0, 255, 0)
|
||||
imgray = cv2.cvtColor(img_textlines, cv2.COLOR_BGR2GRAY)
|
||||
ret, thresh = cv2.threshold(imgray, 0, 255, 0)
|
||||
|
||||
#contours_combined, hierarchy = cv2.findContours(thresh, cv2.RETR_TREE, cv2.CHAIN_APPROX_SIMPLE)
|
||||
contours_combined, hierarchy = cv2.findContours(thresh, cv2.RETR_TREE, cv2.CHAIN_APPROX_SIMPLE)
|
||||
|
||||
# print(len(contours_combined),'len textlines mixed')
|
||||
areas_cnt_text = np.array([cv2.contourArea(contours_combined[j]) for j in range(len(contours_combined))])
|
||||
|
@ -248,12 +231,7 @@ def adhere_drop_capital_region_into_corresponding_textline(
|
|||
contours_biggest[:, 0, 1] = contours_biggest[:, 0, 1] # -all_box_coord[int(region_final)][0]
|
||||
|
||||
##contours_biggest=contours_biggest.reshape(np.shape(contours_biggest)[0],np.shape(contours_biggest)[2])
|
||||
if len(contours_combined)==1:
|
||||
all_found_textline_polygons[int(region_final)][arg_min] = contours_biggest
|
||||
elif len(contours_combined)==2:
|
||||
all_found_textline_polygons[int(region_final)].insert(arg_min, polygons_of_drop_capitals[i_drop] )
|
||||
else:
|
||||
pass
|
||||
all_found_textline_polygons[int(region_final)][arg_min] = contours_biggest
|
||||
# all_found_textline_polygons[int(region_final)][arg_min]=contours_biggest
|
||||
|
||||
except:
|
||||
|
@ -342,12 +320,10 @@ def adhere_drop_capital_region_into_corresponding_textline(
|
|||
img_textlines = cv2.fillPoly(img_textlines, pts=[polygons_of_drop_capitals[i_drop]], color=(255, 255, 255))
|
||||
|
||||
img_textlines = img_textlines.astype(np.uint8)
|
||||
contours_combined = return_contours_of_interested_region(img_textlines, 255, 0)
|
||||
|
||||
#imgray = cv2.cvtColor(img_textlines, cv2.COLOR_BGR2GRAY)
|
||||
#ret, thresh = cv2.threshold(imgray, 0, 255, 0)
|
||||
imgray = cv2.cvtColor(img_textlines, cv2.COLOR_BGR2GRAY)
|
||||
ret, thresh = cv2.threshold(imgray, 0, 255, 0)
|
||||
|
||||
#contours_combined, hierarchy = cv2.findContours(thresh, cv2.RETR_TREE, cv2.CHAIN_APPROX_SIMPLE)
|
||||
contours_combined, hierarchy = cv2.findContours(thresh, cv2.RETR_TREE, cv2.CHAIN_APPROX_SIMPLE)
|
||||
|
||||
# print(len(contours_combined),'len textlines mixed')
|
||||
areas_cnt_text = np.array([cv2.contourArea(contours_combined[j]) for j in range(len(contours_combined))])
|
||||
|
@ -360,12 +336,8 @@ def adhere_drop_capital_region_into_corresponding_textline(
|
|||
contours_biggest[:, 0, 1] = contours_biggest[:, 0, 1] - all_box_coord[int(region_final)][0]
|
||||
|
||||
contours_biggest = contours_biggest.reshape(np.shape(contours_biggest)[0], np.shape(contours_biggest)[2])
|
||||
if len(contours_combined)==1:
|
||||
all_found_textline_polygons[int(region_final)][arg_min] = contours_biggest
|
||||
elif len(contours_combined)==2:
|
||||
all_found_textline_polygons[int(region_final)].insert(arg_min, polygons_of_drop_capitals[i_drop] )
|
||||
else:
|
||||
pass
|
||||
|
||||
all_found_textline_polygons[int(region_final)][arg_min] = contours_biggest
|
||||
|
||||
except:
|
||||
# print('gordun1')
|
||||
|
@ -403,12 +375,10 @@ def adhere_drop_capital_region_into_corresponding_textline(
|
|||
img_textlines = cv2.fillPoly(img_textlines, pts=[polygons_of_drop_capitals[i_drop]], color=(255, 255, 255))
|
||||
|
||||
img_textlines = img_textlines.astype(np.uint8)
|
||||
contours_combined = return_contours_of_interested_region(img_textlines, 255, 0)
|
||||
|
||||
#imgray = cv2.cvtColor(img_textlines, cv2.COLOR_BGR2GRAY)
|
||||
#ret, thresh = cv2.threshold(imgray, 0, 255, 0)
|
||||
imgray = cv2.cvtColor(img_textlines, cv2.COLOR_BGR2GRAY)
|
||||
ret, thresh = cv2.threshold(imgray, 0, 255, 0)
|
||||
|
||||
#contours_combined, hierarchy = cv2.findContours(thresh, cv2.RETR_TREE, cv2.CHAIN_APPROX_SIMPLE)
|
||||
contours_combined, hierarchy = cv2.findContours(thresh, cv2.RETR_TREE, cv2.CHAIN_APPROX_SIMPLE)
|
||||
|
||||
# print(len(contours_combined),'len textlines mixed')
|
||||
areas_cnt_text = np.array([cv2.contourArea(contours_combined[j]) for j in range(len(contours_combined))])
|
||||
|
@ -421,12 +391,7 @@ def adhere_drop_capital_region_into_corresponding_textline(
|
|||
contours_biggest[:, 0, 1] = contours_biggest[:, 0, 1] - all_box_coord[int(region_final)][0]
|
||||
|
||||
contours_biggest = contours_biggest.reshape(np.shape(contours_biggest)[0], np.shape(contours_biggest)[2])
|
||||
if len(contours_combined)==1:
|
||||
all_found_textline_polygons[int(region_final)][arg_min] = contours_biggest
|
||||
elif len(contours_combined)==2:
|
||||
all_found_textline_polygons[int(region_final)].insert(arg_min, polygons_of_drop_capitals[i_drop] )
|
||||
else:
|
||||
pass
|
||||
all_found_textline_polygons[int(region_final)][arg_min] = contours_biggest
|
||||
# all_found_textline_polygons[int(region_final)][arg_min]=contours_biggest
|
||||
|
||||
except:
|
|
@ -2,11 +2,13 @@ import numpy as np
|
|||
import cv2
|
||||
from scipy.signal import find_peaks
|
||||
from scipy.ndimage import gaussian_filter1d
|
||||
|
||||
|
||||
from .contour import find_new_features_of_contours, return_contours_of_interested_region
|
||||
from .resize import resize_image
|
||||
from .rotate import rotate_image
|
||||
|
||||
def get_marginals(text_with_lines, text_regions, num_col, slope_deskew, light_version=False, kernel=None):
|
||||
def get_marginals(text_with_lines, text_regions, num_col, slope_deskew, kernel=None):
|
||||
mask_marginals=np.zeros((text_with_lines.shape[0],text_with_lines.shape[1]))
|
||||
mask_marginals=mask_marginals.astype(np.uint8)
|
||||
|
||||
|
@ -47,14 +49,27 @@ def get_marginals(text_with_lines, text_regions, num_col, slope_deskew, light_ve
|
|||
if thickness_along_y_percent>=14:
|
||||
|
||||
text_with_lines_y_rev=-1*text_with_lines_y[:]
|
||||
#print(text_with_lines_y)
|
||||
#print(text_with_lines_y_rev)
|
||||
|
||||
|
||||
|
||||
|
||||
#plt.plot(text_with_lines_y)
|
||||
#plt.show()
|
||||
|
||||
|
||||
text_with_lines_y_rev=text_with_lines_y_rev-np.min(text_with_lines_y_rev)
|
||||
|
||||
#plt.plot(text_with_lines_y_rev)
|
||||
#plt.show()
|
||||
sigma_gaus=1
|
||||
region_sum_0= gaussian_filter1d(text_with_lines_y, sigma_gaus)
|
||||
|
||||
region_sum_0_rev=gaussian_filter1d(text_with_lines_y_rev, sigma_gaus)
|
||||
|
||||
#plt.plot(region_sum_0_rev)
|
||||
#plt.show()
|
||||
region_sum_0_updown=region_sum_0[len(region_sum_0)::-1]
|
||||
|
||||
first_nonzero=(next((i for i, x in enumerate(region_sum_0) if x), None))
|
||||
|
@ -63,18 +78,44 @@ def get_marginals(text_with_lines, text_regions, num_col, slope_deskew, light_ve
|
|||
|
||||
last_nonzero=len(region_sum_0)-last_nonzero
|
||||
|
||||
##img_sum_0_smooth_rev=-region_sum_0
|
||||
|
||||
|
||||
mid_point=(last_nonzero+first_nonzero)/2.
|
||||
|
||||
|
||||
one_third_right=(last_nonzero-mid_point)/3.0
|
||||
one_third_left=(mid_point-first_nonzero)/3.0
|
||||
|
||||
peaks, _ = find_peaks(text_with_lines_y_rev, height=0)
|
||||
peaks=np.array(peaks)
|
||||
peaks=peaks[(peaks>first_nonzero) & ((peaks<last_nonzero))]
|
||||
peaks=peaks[region_sum_0[peaks]<min_textline_thickness ]
|
||||
#img_sum_0_smooth_rev=img_sum_0_smooth_rev-np.min(img_sum_0_smooth_rev)
|
||||
|
||||
|
||||
|
||||
|
||||
peaks, _ = find_peaks(text_with_lines_y_rev, height=0)
|
||||
|
||||
|
||||
peaks=np.array(peaks)
|
||||
|
||||
|
||||
#print(region_sum_0[peaks])
|
||||
##plt.plot(region_sum_0)
|
||||
##plt.plot(peaks,region_sum_0[peaks],'*')
|
||||
##plt.show()
|
||||
#print(first_nonzero,last_nonzero,peaks)
|
||||
peaks=peaks[(peaks>first_nonzero) & ((peaks<last_nonzero))]
|
||||
|
||||
#print(first_nonzero,last_nonzero,peaks)
|
||||
|
||||
|
||||
#print(region_sum_0[peaks]<10)
|
||||
####peaks=peaks[region_sum_0[peaks]<25 ]
|
||||
|
||||
#print(region_sum_0[peaks])
|
||||
peaks=peaks[region_sum_0[peaks]<min_textline_thickness ]
|
||||
#print(peaks)
|
||||
#print(first_nonzero,last_nonzero,one_third_right,one_third_left)
|
||||
|
||||
if num_col==1:
|
||||
peaks_right=peaks[peaks>mid_point]
|
||||
peaks_left=peaks[peaks<mid_point]
|
||||
|
@ -96,6 +137,9 @@ def get_marginals(text_with_lines, text_regions, num_col, slope_deskew, light_ve
|
|||
|
||||
|
||||
|
||||
|
||||
#print(point_left,point_right)
|
||||
#print(text_regions.shape)
|
||||
if point_right>=mask_marginals.shape[1]:
|
||||
point_right=mask_marginals.shape[1]-1
|
||||
|
||||
|
@ -104,8 +148,10 @@ def get_marginals(text_with_lines, text_regions, num_col, slope_deskew, light_ve
|
|||
except:
|
||||
mask_marginals[:,:]=1
|
||||
|
||||
#print(mask_marginals.shape,point_left,point_right,'nadosh')
|
||||
mask_marginals_rotated=rotate_image(mask_marginals,-slope_deskew)
|
||||
|
||||
#print(mask_marginals_rotated.shape,'nadosh')
|
||||
mask_marginals_rotated_sum=mask_marginals_rotated.sum(axis=0)
|
||||
|
||||
mask_marginals_rotated_sum[mask_marginals_rotated_sum!=0]=1
|
||||
|
@ -121,92 +167,73 @@ def get_marginals(text_with_lines, text_regions, num_col, slope_deskew, light_ve
|
|||
if max_point_of_right_marginal>=text_regions.shape[1]:
|
||||
max_point_of_right_marginal=text_regions.shape[1]-1
|
||||
|
||||
if light_version:
|
||||
text_regions_org = np.copy(text_regions)
|
||||
text_regions[text_regions[:,:]==1]=4
|
||||
|
||||
pixel_img=4
|
||||
min_area_text=0.00001
|
||||
|
||||
polygon_mask_marginals_rotated = return_contours_of_interested_region(mask_marginals,1,min_area_text)
|
||||
|
||||
polygon_mask_marginals_rotated = polygon_mask_marginals_rotated[0]
|
||||
|
||||
polygons_of_marginals=return_contours_of_interested_region(text_regions,pixel_img,min_area_text)
|
||||
#print(np.min(index_x_interest) ,np.max(index_x_interest),'minmaxnew')
|
||||
#print(mask_marginals_rotated.shape,text_regions.shape,'mask_marginals_rotated')
|
||||
#plt.imshow(mask_marginals)
|
||||
#plt.show()
|
||||
|
||||
cx_text_only,cy_text_only ,x_min_text_only,x_max_text_only, y_min_text_only ,y_max_text_only,y_cor_x_min_main=find_new_features_of_contours(polygons_of_marginals)
|
||||
#plt.imshow(mask_marginals_rotated)
|
||||
#plt.show()
|
||||
|
||||
text_regions[(text_regions[:,:]==4)]=1
|
||||
text_regions[(mask_marginals_rotated[:,:]!=1) & (text_regions[:,:]==1)]=4
|
||||
|
||||
marginlas_should_be_main_text=[]
|
||||
#plt.imshow(text_regions)
|
||||
#plt.show()
|
||||
|
||||
x_min_marginals_left=[]
|
||||
x_min_marginals_right=[]
|
||||
pixel_img=4
|
||||
min_area_text=0.00001
|
||||
polygons_of_marginals=return_contours_of_interested_region(text_regions,pixel_img,min_area_text)
|
||||
|
||||
for i in range(len(cx_text_only)):
|
||||
results = cv2.pointPolygonTest(polygon_mask_marginals_rotated, (cx_text_only[i], cy_text_only[i]), False)
|
||||
cx_text_only,cy_text_only ,x_min_text_only,x_max_text_only, y_min_text_only ,y_max_text_only,y_cor_x_min_main=find_new_features_of_contours(polygons_of_marginals)
|
||||
|
||||
if results == -1:
|
||||
marginlas_should_be_main_text.append(polygons_of_marginals[i])
|
||||
text_regions[(text_regions[:,:]==4)]=1
|
||||
|
||||
marginlas_should_be_main_text=[]
|
||||
|
||||
x_min_marginals_left=[]
|
||||
x_min_marginals_right=[]
|
||||
|
||||
text_regions_org=cv2.fillPoly(text_regions_org, pts =marginlas_should_be_main_text, color=(4,4))
|
||||
text_regions = np.copy(text_regions_org)
|
||||
|
||||
for i in range(len(cx_text_only)):
|
||||
|
||||
else:
|
||||
|
||||
text_regions[(mask_marginals_rotated[:,:]!=1) & (text_regions[:,:]==1)]=4
|
||||
|
||||
pixel_img=4
|
||||
min_area_text=0.00001
|
||||
|
||||
polygons_of_marginals=return_contours_of_interested_region(text_regions,pixel_img,min_area_text)
|
||||
|
||||
cx_text_only,cy_text_only ,x_min_text_only,x_max_text_only, y_min_text_only ,y_max_text_only,y_cor_x_min_main=find_new_features_of_contours(polygons_of_marginals)
|
||||
|
||||
text_regions[(text_regions[:,:]==4)]=1
|
||||
|
||||
marginlas_should_be_main_text=[]
|
||||
|
||||
x_min_marginals_left=[]
|
||||
x_min_marginals_right=[]
|
||||
|
||||
for i in range(len(cx_text_only)):
|
||||
x_width_mar=abs(x_min_text_only[i]-x_max_text_only[i])
|
||||
y_height_mar=abs(y_min_text_only[i]-y_max_text_only[i])
|
||||
|
||||
if x_width_mar>16 and y_height_mar/x_width_mar<18:
|
||||
marginlas_should_be_main_text.append(polygons_of_marginals[i])
|
||||
if x_min_text_only[i]<(mid_point-one_third_left):
|
||||
x_min_marginals_left_new=x_min_text_only[i]
|
||||
if len(x_min_marginals_left)==0:
|
||||
x_min_marginals_left.append(x_min_marginals_left_new)
|
||||
else:
|
||||
x_min_marginals_left[0]=min(x_min_marginals_left[0],x_min_marginals_left_new)
|
||||
x_width_mar=abs(x_min_text_only[i]-x_max_text_only[i])
|
||||
y_height_mar=abs(y_min_text_only[i]-y_max_text_only[i])
|
||||
#print(x_width_mar,y_height_mar,y_height_mar/x_width_mar,'y_height_mar')
|
||||
if x_width_mar>16 and y_height_mar/x_width_mar<18:
|
||||
marginlas_should_be_main_text.append(polygons_of_marginals[i])
|
||||
if x_min_text_only[i]<(mid_point-one_third_left):
|
||||
x_min_marginals_left_new=x_min_text_only[i]
|
||||
if len(x_min_marginals_left)==0:
|
||||
x_min_marginals_left.append(x_min_marginals_left_new)
|
||||
else:
|
||||
x_min_marginals_right_new=x_min_text_only[i]
|
||||
if len(x_min_marginals_right)==0:
|
||||
x_min_marginals_right.append(x_min_marginals_right_new)
|
||||
else:
|
||||
x_min_marginals_right[0]=min(x_min_marginals_right[0],x_min_marginals_right_new)
|
||||
x_min_marginals_left[0]=min(x_min_marginals_left[0],x_min_marginals_left_new)
|
||||
else:
|
||||
x_min_marginals_right_new=x_min_text_only[i]
|
||||
if len(x_min_marginals_right)==0:
|
||||
x_min_marginals_right.append(x_min_marginals_right_new)
|
||||
else:
|
||||
x_min_marginals_right[0]=min(x_min_marginals_right[0],x_min_marginals_right_new)
|
||||
|
||||
if len(x_min_marginals_left)==0:
|
||||
x_min_marginals_left=[0]
|
||||
if len(x_min_marginals_right)==0:
|
||||
x_min_marginals_right=[text_regions.shape[1]-1]
|
||||
if len(x_min_marginals_left)==0:
|
||||
x_min_marginals_left=[0]
|
||||
if len(x_min_marginals_right)==0:
|
||||
x_min_marginals_right=[text_regions.shape[1]-1]
|
||||
|
||||
|
||||
text_regions=cv2.fillPoly(text_regions, pts =marginlas_should_be_main_text, color=(4,4))
|
||||
|
||||
|
||||
#text_regions[:,:int(x_min_marginals_left[0])][text_regions[:,:int(x_min_marginals_left[0])]==1]=0
|
||||
#text_regions[:,int(x_min_marginals_right[0]):][text_regions[:,int(x_min_marginals_right[0]):]==1]=0
|
||||
|
||||
|
||||
text_regions[:,:int(min_point_of_left_marginal)][text_regions[:,:int(min_point_of_left_marginal)]==1]=0
|
||||
text_regions[:,int(max_point_of_right_marginal):][text_regions[:,int(max_point_of_right_marginal):]==1]=0
|
||||
#print(x_min_marginals_left[0],x_min_marginals_right[0],'margo')
|
||||
|
||||
#print(marginlas_should_be_main_text,'marginlas_should_be_main_text')
|
||||
text_regions=cv2.fillPoly(text_regions, pts =marginlas_should_be_main_text, color=(4,4))
|
||||
|
||||
#print(np.unique(text_regions))
|
||||
|
||||
#text_regions[:,:int(x_min_marginals_left[0])][text_regions[:,:int(x_min_marginals_left[0])]==1]=0
|
||||
#text_regions[:,int(x_min_marginals_right[0]):][text_regions[:,int(x_min_marginals_right[0]):]==1]=0
|
||||
|
||||
text_regions[:,:int(min_point_of_left_marginal)][text_regions[:,:int(min_point_of_left_marginal)]==1]=0
|
||||
text_regions[:,int(max_point_of_right_marginal):][text_regions[:,int(max_point_of_right_marginal):]==1]=0
|
||||
|
||||
###text_regions[:,0:point_left][text_regions[:,0:point_left]==1]=4
|
||||
|
|
@ -1,4 +1,3 @@
|
|||
from contextlib import nullcontext
|
||||
from PIL import Image
|
||||
import numpy as np
|
||||
from ocrd_models import OcrdExif
|
||||
|
@ -18,13 +17,12 @@ def pil2cv(img):
|
|||
def check_dpi(img):
|
||||
try:
|
||||
if isinstance(img, Image.Image):
|
||||
pil_image = nullcontext(img)
|
||||
pil_image = img
|
||||
elif isinstance(img, str):
|
||||
pil_image = Image.open(img)
|
||||
else:
|
||||
pil_image = nullcontext(cv2pil(img))
|
||||
with pil_image:
|
||||
exif = OcrdExif(pil_image)
|
||||
pil_image = cv2pil(img)
|
||||
exif = OcrdExif(pil_image)
|
||||
resolution = exif.resolution
|
||||
if resolution == 1:
|
||||
raise Exception()
|
|
@ -1,4 +1,6 @@
|
|||
import math
|
||||
|
||||
import imutils
|
||||
import cv2
|
||||
|
||||
def rotatedRectWithMaxArea(w, h, angle):
|
||||
|
@ -33,14 +35,14 @@ def rotate_max_area_new(image, rotated, angle):
|
|||
return rotated[y1:y2, x1:x2]
|
||||
|
||||
def rotation_image_new(img, thetha):
|
||||
rotated = rotate_image(img, thetha)
|
||||
rotated = imutils.rotate(img, thetha)
|
||||
return rotate_max_area_new(img, rotated, thetha)
|
||||
|
||||
def rotate_image(img_patch, slope):
|
||||
(h, w) = img_patch.shape[:2]
|
||||
center = (w // 2, h // 2)
|
||||
M = cv2.getRotationMatrix2D(center, slope, 1.0)
|
||||
return cv2.warpAffine(img_patch, M, (w, h) )
|
||||
return cv2.warpAffine(img_patch, M, (w, h), flags=cv2.INTER_CUBIC, borderMode=cv2.BORDER_REPLICATE)
|
||||
|
||||
def rotate_image_different( img, slope):
|
||||
# img = cv2.imread('images/input.jpg')
|
||||
|
@ -60,17 +62,17 @@ def rotate_max_area(image, rotated, rotated_textline, rotated_layout, rotated_ta
|
|||
return rotated[y1:y2, x1:x2], rotated_textline[y1:y2, x1:x2], rotated_layout[y1:y2, x1:x2], rotated_table_prediction[y1:y2, x1:x2]
|
||||
|
||||
def rotation_not_90_func(img, textline, text_regions_p_1, table_prediction, thetha):
|
||||
rotated = rotate_image(img, thetha)
|
||||
rotated_textline = rotate_image(textline, thetha)
|
||||
rotated_layout = rotate_image(text_regions_p_1, thetha)
|
||||
rotated_table_prediction = rotate_image(table_prediction, thetha)
|
||||
rotated = imutils.rotate(img, thetha)
|
||||
rotated_textline = imutils.rotate(textline, thetha)
|
||||
rotated_layout = imutils.rotate(text_regions_p_1, thetha)
|
||||
rotated_table_prediction = imutils.rotate(table_prediction, thetha)
|
||||
return rotate_max_area(img, rotated, rotated_textline, rotated_layout, rotated_table_prediction, thetha)
|
||||
|
||||
def rotation_not_90_func_full_layout(img, textline, text_regions_p_1, text_regions_p_fully, thetha):
|
||||
rotated = rotate_image(img, thetha)
|
||||
rotated_textline = rotate_image(textline, thetha)
|
||||
rotated_layout = rotate_image(text_regions_p_1, thetha)
|
||||
rotated_layout_full = rotate_image(text_regions_p_fully, thetha)
|
||||
rotated = imutils.rotate(img, thetha)
|
||||
rotated_textline = imutils.rotate(textline, thetha)
|
||||
rotated_layout = imutils.rotate(text_regions_p_1, thetha)
|
||||
rotated_layout_full = imutils.rotate(text_regions_p_fully, thetha)
|
||||
return rotate_max_area_full_layout(img, rotated, rotated_textline, rotated_layout, rotated_layout_full, thetha)
|
||||
|
||||
def rotate_max_area_full_layout(image, rotated, rotated_textline, rotated_layout, rotated_layout_full, angle):
|
File diff suppressed because it is too large
Load diff
|
@ -72,7 +72,7 @@ def order_and_id_of_texts(found_polygons_text_region, found_polygons_text_region
|
|||
|
||||
index_of_types_2 = index_of_types[kind_of_texts == 2]
|
||||
indexes_sorted_2 = indexes_sorted[kind_of_texts == 2]
|
||||
|
||||
|
||||
counter = EynollahIdCounter(region_idx=ref_point)
|
||||
for idx_textregion, _ in enumerate(found_polygons_text_region):
|
||||
id_of_texts.append(counter.next_region_id)
|
|
@ -2,7 +2,7 @@
|
|||
# pylint: disable=import-error
|
||||
from pathlib import Path
|
||||
import os.path
|
||||
import xml.etree.ElementTree as ET
|
||||
|
||||
from .utils.xml import create_page_xml, xml_reading_order
|
||||
from .utils.counter import EynollahIdCounter
|
||||
|
||||
|
@ -12,7 +12,6 @@ from ocrd_models.ocrd_page import (
|
|||
CoordsType,
|
||||
PcGtsType,
|
||||
TextLineType,
|
||||
TextEquivType,
|
||||
TextRegionType,
|
||||
ImageRegionType,
|
||||
TableRegionType,
|
||||
|
@ -28,7 +27,6 @@ class EynollahXmlWriter():
|
|||
self.counter = EynollahIdCounter()
|
||||
self.dir_out = dir_out
|
||||
self.image_filename = image_filename
|
||||
self.output_filename = os.path.join(self.dir_out or "", self.image_filename_stem) + ".xml"
|
||||
self.curved_line = curved_line
|
||||
self.textline_light = textline_light
|
||||
self.pcgts = pcgts
|
||||
|
@ -61,7 +59,6 @@ class EynollahXmlWriter():
|
|||
coords = CoordsType()
|
||||
textline = TextLineType(id=counter.next_line_id, Coords=coords)
|
||||
marginal_region.add_TextLine(textline)
|
||||
marginal_region.set_orientation(-slopes_marginals[marginal_idx])
|
||||
points_co = ''
|
||||
for l in range(len(all_found_textline_polygons_marginals[marginal_idx][j])):
|
||||
if not (self.curved_line or self.textline_light):
|
||||
|
@ -96,15 +93,12 @@ class EynollahXmlWriter():
|
|||
points_co += ' '
|
||||
coords.set_points(points_co[:-1])
|
||||
|
||||
def serialize_lines_in_region(self, text_region, all_found_textline_polygons, region_idx, page_coord, all_box_coord, slopes, counter, ocr_all_textlines_textregion):
|
||||
def serialize_lines_in_region(self, text_region, all_found_textline_polygons, region_idx, page_coord, all_box_coord, slopes, counter):
|
||||
self.logger.debug('enter serialize_lines_in_region')
|
||||
for j in range(len(all_found_textline_polygons[region_idx])):
|
||||
coords = CoordsType()
|
||||
textline = TextLineType(id=counter.next_line_id, Coords=coords)
|
||||
if ocr_all_textlines_textregion:
|
||||
textline.set_TextEquiv( [ TextEquivType(Unicode=ocr_all_textlines_textregion[j]) ] )
|
||||
text_region.add_TextLine(textline)
|
||||
text_region.set_orientation(-slopes[region_idx])
|
||||
region_bboxes = all_box_coord[region_idx]
|
||||
points_co = ''
|
||||
for idx_contour_textline, contour_textline in enumerate(all_found_textline_polygons[region_idx][j]):
|
||||
|
@ -140,35 +134,13 @@ class EynollahXmlWriter():
|
|||
points_co += ' '
|
||||
coords.set_points(points_co[:-1])
|
||||
|
||||
def serialize_lines_in_dropcapital(self, text_region, all_found_textline_polygons, region_idx, page_coord, all_box_coord, slopes, counter, ocr_all_textlines_textregion):
|
||||
self.logger.debug('enter serialize_lines_in_region')
|
||||
for j in range(1):
|
||||
coords = CoordsType()
|
||||
textline = TextLineType(id=counter.next_line_id, Coords=coords)
|
||||
if ocr_all_textlines_textregion:
|
||||
textline.set_TextEquiv( [ TextEquivType(Unicode=ocr_all_textlines_textregion[j]) ] )
|
||||
text_region.add_TextLine(textline)
|
||||
#region_bboxes = all_box_coord[region_idx]
|
||||
points_co = ''
|
||||
for idx_contour_textline, contour_textline in enumerate(all_found_textline_polygons[j]):
|
||||
if len(contour_textline) == 2:
|
||||
points_co += str(int((contour_textline[0] + page_coord[2]) / self.scale_x))
|
||||
points_co += ','
|
||||
points_co += str(int((contour_textline[1] + page_coord[0]) / self.scale_y))
|
||||
else:
|
||||
points_co += str(int((contour_textline[0][0] + page_coord[2]) / self.scale_x))
|
||||
points_co += ','
|
||||
points_co += str(int((contour_textline[0][1] + page_coord[0])/self.scale_y))
|
||||
|
||||
points_co += ' '
|
||||
coords.set_points(points_co[:-1])
|
||||
|
||||
def write_pagexml(self, pcgts):
|
||||
self.logger.info("output filename: '%s'", self.output_filename)
|
||||
with open(self.output_filename, 'w') as f:
|
||||
out_fname = os.path.join(self.dir_out, self.image_filename_stem) + ".xml"
|
||||
self.logger.info("output filename: '%s'", out_fname)
|
||||
with open(out_fname, 'w') as f:
|
||||
f.write(to_xml(pcgts))
|
||||
|
||||
def build_pagexml_no_full_layout(self, found_polygons_text_region, page_coord, order_of_texts, id_of_texts, all_found_textline_polygons, all_box_coord, found_polygons_text_region_img, found_polygons_marginals, all_found_textline_polygons_marginals, all_box_coord_marginals, slopes, slopes_marginals, cont_page, polygons_lines_to_be_written_in_xml, found_polygons_tables, ocr_all_textlines, conf_contours_textregion):
|
||||
def build_pagexml_no_full_layout(self, found_polygons_text_region, page_coord, order_of_texts, id_of_texts, all_found_textline_polygons, all_box_coord, found_polygons_text_region_img, found_polygons_marginals, all_found_textline_polygons_marginals, all_box_coord_marginals, slopes, slopes_marginals, cont_page, polygons_lines_to_be_written_in_xml, found_polygons_tables):
|
||||
self.logger.debug('enter build_pagexml_no_full_layout')
|
||||
|
||||
# create the file structure
|
||||
|
@ -184,15 +156,10 @@ class EynollahXmlWriter():
|
|||
|
||||
for mm in range(len(found_polygons_text_region)):
|
||||
textregion = TextRegionType(id=counter.next_region_id, type_='paragraph',
|
||||
Coords=CoordsType(points=self.calculate_polygon_coords(found_polygons_text_region[mm], page_coord), conf=conf_contours_textregion[mm]),
|
||||
Coords=CoordsType(points=self.calculate_polygon_coords(found_polygons_text_region[mm], page_coord)),
|
||||
)
|
||||
#textregion.set_conf(conf_contours_textregion[mm])
|
||||
page.add_TextRegion(textregion)
|
||||
if ocr_all_textlines:
|
||||
ocr_textlines = ocr_all_textlines[mm]
|
||||
else:
|
||||
ocr_textlines = None
|
||||
self.serialize_lines_in_region(textregion, all_found_textline_polygons, mm, page_coord, all_box_coord, slopes, counter, ocr_textlines)
|
||||
self.serialize_lines_in_region(textregion, all_found_textline_polygons, mm, page_coord, all_box_coord, slopes, counter)
|
||||
|
||||
for mm in range(len(found_polygons_marginals)):
|
||||
marginal = TextRegionType(id=counter.next_region_id, type_='marginalia',
|
||||
|
@ -205,20 +172,12 @@ class EynollahXmlWriter():
|
|||
page.add_ImageRegion(img_region)
|
||||
points_co = ''
|
||||
for lmm in range(len(found_polygons_text_region_img[mm])):
|
||||
try:
|
||||
points_co += str(int((found_polygons_text_region_img[mm][lmm,0,0] + page_coord[2]) / self.scale_x))
|
||||
points_co += ','
|
||||
points_co += str(int((found_polygons_text_region_img[mm][lmm,0,1] + page_coord[0]) / self.scale_y))
|
||||
points_co += ' '
|
||||
except:
|
||||
|
||||
points_co += str(int((found_polygons_text_region_img[mm][lmm][0] + page_coord[2])/ self.scale_x ))
|
||||
points_co += ','
|
||||
points_co += str(int((found_polygons_text_region_img[mm][lmm][1] + page_coord[0])/ self.scale_y ))
|
||||
points_co += ' '
|
||||
|
||||
points_co += str(int((found_polygons_text_region_img[mm][lmm,0,0] + page_coord[2]) / self.scale_x))
|
||||
points_co += ','
|
||||
points_co += str(int((found_polygons_text_region_img[mm][lmm,0,1] + page_coord[0]) / self.scale_y))
|
||||
points_co += ' '
|
||||
img_region.get_Coords().set_points(points_co[:-1])
|
||||
|
||||
|
||||
for mm in range(len(polygons_lines_to_be_written_in_xml)):
|
||||
sep_hor = SeparatorRegionType(id=counter.next_region_id, Coords=CoordsType())
|
||||
page.add_SeparatorRegion(sep_hor)
|
||||
|
@ -242,7 +201,7 @@ class EynollahXmlWriter():
|
|||
|
||||
return pcgts
|
||||
|
||||
def build_pagexml_full_layout(self, found_polygons_text_region, found_polygons_text_region_h, page_coord, order_of_texts, id_of_texts, all_found_textline_polygons, all_found_textline_polygons_h, all_box_coord, all_box_coord_h, found_polygons_text_region_img, found_polygons_tables, found_polygons_drop_capitals, found_polygons_marginals, all_found_textline_polygons_marginals, all_box_coord_marginals, slopes, slopes_h, slopes_marginals, cont_page, polygons_lines_to_be_written_in_xml, ocr_all_textlines, conf_contours_textregion, conf_contours_textregion_h):
|
||||
def build_pagexml_full_layout(self, found_polygons_text_region, found_polygons_text_region_h, page_coord, order_of_texts, id_of_texts, all_found_textline_polygons, all_found_textline_polygons_h, all_box_coord, all_box_coord_h, found_polygons_text_region_img, found_polygons_tables, found_polygons_drop_capitals, found_polygons_marginals, all_found_textline_polygons_marginals, all_box_coord_marginals, slopes, slopes_h, slopes_marginals, cont_page, polygons_lines_to_be_written_in_xml):
|
||||
self.logger.debug('enter build_pagexml_full_layout')
|
||||
|
||||
# create the file structure
|
||||
|
@ -257,26 +216,16 @@ class EynollahXmlWriter():
|
|||
|
||||
for mm in range(len(found_polygons_text_region)):
|
||||
textregion = TextRegionType(id=counter.next_region_id, type_='paragraph',
|
||||
Coords=CoordsType(points=self.calculate_polygon_coords(found_polygons_text_region[mm], page_coord), conf=conf_contours_textregion[mm]))
|
||||
Coords=CoordsType(points=self.calculate_polygon_coords(found_polygons_text_region[mm], page_coord)))
|
||||
page.add_TextRegion(textregion)
|
||||
|
||||
if ocr_all_textlines:
|
||||
ocr_textlines = ocr_all_textlines[mm]
|
||||
else:
|
||||
ocr_textlines = None
|
||||
self.serialize_lines_in_region(textregion, all_found_textline_polygons, mm, page_coord, all_box_coord, slopes, counter, ocr_textlines)
|
||||
self.serialize_lines_in_region(textregion, all_found_textline_polygons, mm, page_coord, all_box_coord, slopes, counter)
|
||||
|
||||
self.logger.debug('len(found_polygons_text_region_h) %s', len(found_polygons_text_region_h))
|
||||
for mm in range(len(found_polygons_text_region_h)):
|
||||
textregion = TextRegionType(id=counter.next_region_id, type_='header',
|
||||
Coords=CoordsType(points=self.calculate_polygon_coords(found_polygons_text_region_h[mm], page_coord)))
|
||||
page.add_TextRegion(textregion)
|
||||
|
||||
if ocr_all_textlines:
|
||||
ocr_textlines = ocr_all_textlines[mm]
|
||||
else:
|
||||
ocr_textlines = None
|
||||
self.serialize_lines_in_region(textregion, all_found_textline_polygons_h, mm, page_coord, all_box_coord_h, slopes_h, counter, ocr_textlines)
|
||||
self.serialize_lines_in_region(textregion, all_found_textline_polygons_h, mm, page_coord, all_box_coord_h, slopes_h, counter)
|
||||
|
||||
for mm in range(len(found_polygons_marginals)):
|
||||
marginal = TextRegionType(id=counter.next_region_id, type_='marginalia',
|
||||
|
@ -285,19 +234,15 @@ class EynollahXmlWriter():
|
|||
self.serialize_lines_in_marginal(marginal, all_found_textline_polygons_marginals, mm, page_coord, all_box_coord_marginals, slopes_marginals, counter)
|
||||
|
||||
for mm in range(len(found_polygons_drop_capitals)):
|
||||
dropcapital = TextRegionType(id=counter.next_region_id, type_='drop-capital',
|
||||
Coords=CoordsType(points=self.calculate_polygon_coords(found_polygons_drop_capitals[mm], page_coord)))
|
||||
page.add_TextRegion(dropcapital)
|
||||
###all_box_coord_drop = None
|
||||
###slopes_drop = None
|
||||
###self.serialize_lines_in_dropcapital(dropcapital, [found_polygons_drop_capitals[mm]], mm, page_coord, all_box_coord_drop, slopes_drop, counter, ocr_all_textlines_textregion=None)
|
||||
page.add_TextRegion(TextRegionType(id=counter.next_region_id, type_='drop-capital',
|
||||
Coords=CoordsType(points=self.calculate_polygon_coords(found_polygons_drop_capitals[mm], page_coord))))
|
||||
|
||||
for mm in range(len(found_polygons_text_region_img)):
|
||||
page.add_ImageRegion(ImageRegionType(id=counter.next_region_id, Coords=CoordsType(points=self.calculate_polygon_coords(found_polygons_text_region_img[mm], page_coord))))
|
||||
|
||||
|
||||
for mm in range(len(polygons_lines_to_be_written_in_xml)):
|
||||
page.add_SeparatorRegion(ImageRegionType(id=counter.next_region_id, Coords=CoordsType(points=self.calculate_polygon_coords(polygons_lines_to_be_written_in_xml[mm], [0 , 0, 0, 0]))))
|
||||
|
||||
|
||||
for mm in range(len(found_polygons_tables)):
|
||||
page.add_TableRegion(TableRegionType(id=counter.next_region_id, Coords=CoordsType(points=self.calculate_polygon_coords(found_polygons_tables[mm], page_coord))))
|
||||
|
|
@ -1,4 +1,2 @@
|
|||
pytest
|
||||
pytest-subtests
|
||||
coverage[toml]
|
||||
black
|
||||
|
|
|
@ -1,7 +1,8 @@
|
|||
# ocrd includes opencv, numpy, shapely, click
|
||||
ocrd >= 3.3.0
|
||||
ocrd >= 2.23.3
|
||||
numpy <1.24.0
|
||||
scikit-learn >= 0.23.2
|
||||
tensorflow < 2.13
|
||||
numba <= 0.58.1
|
||||
loky
|
||||
tensorflow == 2.12.1
|
||||
imutils >= 0.5.3
|
||||
matplotlib
|
||||
setuptools >= 50
|
||||
|
|
27
setup.py
Normal file
27
setup.py
Normal file
|
@ -0,0 +1,27 @@
|
|||
from setuptools import setup, find_namespace_packages
|
||||
from json import load
|
||||
|
||||
install_requires = open('requirements.txt').read().split('\n')
|
||||
with open('ocrd-tool.json', 'r', encoding='utf-8') as f:
|
||||
version = load(f)['version']
|
||||
|
||||
setup(
|
||||
name='eynollah',
|
||||
version=version,
|
||||
long_description=open('README.md').read(),
|
||||
long_description_content_type='text/markdown',
|
||||
author='Vahid Rezanezhad',
|
||||
url='https://github.com/qurator-spk/eynollah',
|
||||
license='Apache License 2.0',
|
||||
packages=find_namespace_packages(include=['qurator']),
|
||||
install_requires=install_requires,
|
||||
package_data={
|
||||
'': ['*.json']
|
||||
},
|
||||
entry_points={
|
||||
'console_scripts': [
|
||||
'eynollah=qurator.eynollah.cli:main',
|
||||
'ocrd-eynollah-segment=qurator.eynollah.ocrd_cli:main',
|
||||
]
|
||||
},
|
||||
)
|
|
@ -1,404 +0,0 @@
|
|||
import sys
|
||||
import click
|
||||
from ocrd_utils import initLogging, getLevelName, getLogger
|
||||
from eynollah.eynollah import Eynollah, Eynollah_ocr
|
||||
from eynollah.sbb_binarize import SbbBinarizer
|
||||
|
||||
@click.group()
|
||||
def main():
|
||||
pass
|
||||
|
||||
@main.command()
|
||||
@click.option(
|
||||
"--dir_xml",
|
||||
"-dx",
|
||||
help="directory of GT page-xml files",
|
||||
type=click.Path(exists=True, file_okay=False),
|
||||
)
|
||||
@click.option(
|
||||
"--dir_out_modal_image",
|
||||
"-domi",
|
||||
help="directory where ground truth images would be written",
|
||||
type=click.Path(exists=True, file_okay=False),
|
||||
)
|
||||
@click.option(
|
||||
"--dir_out_classes",
|
||||
"-docl",
|
||||
help="directory where ground truth classes would be written",
|
||||
type=click.Path(exists=True, file_okay=False),
|
||||
)
|
||||
@click.option(
|
||||
"--input_height",
|
||||
"-ih",
|
||||
help="input height",
|
||||
)
|
||||
@click.option(
|
||||
"--input_width",
|
||||
"-iw",
|
||||
help="input width",
|
||||
)
|
||||
@click.option(
|
||||
"--min_area_size",
|
||||
"-min",
|
||||
help="min area size of regions considered for reading order training.",
|
||||
)
|
||||
def machine_based_reading_order(dir_xml, dir_out_modal_image, dir_out_classes, input_height, input_width, min_area_size):
|
||||
xml_files_ind = os.listdir(dir_xml)
|
||||
|
||||
@main.command()
|
||||
@click.option('--patches/--no-patches', default=True, help='by enabling this parameter you let the model to see the image in patches.')
|
||||
@click.option('--model_dir', '-m', type=click.Path(exists=True, file_okay=False), required=True, help='directory containing models for prediction')
|
||||
@click.argument('input_image', required=False)
|
||||
@click.argument('output_image', required=False)
|
||||
@click.option(
|
||||
"--dir_in",
|
||||
"-di",
|
||||
help="directory of input images",
|
||||
type=click.Path(exists=True, file_okay=False),
|
||||
)
|
||||
@click.option(
|
||||
"--dir_out",
|
||||
"-do",
|
||||
help="directory for output images",
|
||||
type=click.Path(exists=True, file_okay=False),
|
||||
)
|
||||
def binarization(patches, model_dir, input_image, output_image, dir_in, dir_out):
|
||||
assert (dir_out is None) == (dir_in is None), "Options -di and -do are mutually dependent"
|
||||
assert (input_image is None) == (output_image is None), "INPUT_IMAGE and OUTPUT_IMAGE are mutually dependent"
|
||||
assert (dir_in is None) != (input_image is None), "Specify either -di and -do options, or INPUT_IMAGE and OUTPUT_IMAGE"
|
||||
SbbBinarizer(model_dir).run(image_path=input_image, use_patches=patches, save=output_image, dir_in=dir_in, dir_out=dir_out)
|
||||
|
||||
|
||||
|
||||
|
||||
@main.command()
|
||||
@click.option(
|
||||
"--image",
|
||||
"-i",
|
||||
help="image filename",
|
||||
type=click.Path(exists=True, dir_okay=False),
|
||||
)
|
||||
|
||||
@click.option(
|
||||
"--out",
|
||||
"-o",
|
||||
help="directory to write output xml data",
|
||||
type=click.Path(exists=True, file_okay=False),
|
||||
required=True,
|
||||
)
|
||||
@click.option(
|
||||
"--overwrite",
|
||||
"-O",
|
||||
help="overwrite (instead of skipping) if output xml exists",
|
||||
is_flag=True,
|
||||
)
|
||||
@click.option(
|
||||
"--dir_in",
|
||||
"-di",
|
||||
help="directory of images",
|
||||
type=click.Path(exists=True, file_okay=False),
|
||||
)
|
||||
@click.option(
|
||||
"--model",
|
||||
"-m",
|
||||
help="directory of models",
|
||||
type=click.Path(exists=True, file_okay=False),
|
||||
required=True,
|
||||
)
|
||||
@click.option(
|
||||
"--save_images",
|
||||
"-si",
|
||||
help="if a directory is given, images in documents will be cropped and saved there",
|
||||
type=click.Path(exists=True, file_okay=False),
|
||||
)
|
||||
@click.option(
|
||||
"--save_layout",
|
||||
"-sl",
|
||||
help="if a directory is given, plot of layout will be saved there",
|
||||
type=click.Path(exists=True, file_okay=False),
|
||||
)
|
||||
@click.option(
|
||||
"--save_deskewed",
|
||||
"-sd",
|
||||
help="if a directory is given, deskewed image will be saved there",
|
||||
type=click.Path(exists=True, file_okay=False),
|
||||
)
|
||||
@click.option(
|
||||
"--save_all",
|
||||
"-sa",
|
||||
help="if a directory is given, all plots needed for documentation will be saved there",
|
||||
type=click.Path(exists=True, file_okay=False),
|
||||
)
|
||||
@click.option(
|
||||
"--save_page",
|
||||
"-sp",
|
||||
help="if a directory is given, page crop of image will be saved there",
|
||||
type=click.Path(exists=True, file_okay=False),
|
||||
)
|
||||
@click.option(
|
||||
"--enable-plotting/--disable-plotting",
|
||||
"-ep/-noep",
|
||||
is_flag=True,
|
||||
help="If set, will plot intermediary files and images",
|
||||
)
|
||||
@click.option(
|
||||
"--extract_only_images/--disable-extracting_only_images",
|
||||
"-eoi/-noeoi",
|
||||
is_flag=True,
|
||||
help="If a directory is given, only images in documents will be cropped and saved there and the other processing will not be done",
|
||||
)
|
||||
@click.option(
|
||||
"--allow-enhancement/--no-allow-enhancement",
|
||||
"-ae/-noae",
|
||||
is_flag=True,
|
||||
help="if this parameter set to true, this tool would check that input image need resizing and enhancement or not. If so output of resized and enhanced image and corresponding layout data will be written in out directory",
|
||||
)
|
||||
@click.option(
|
||||
"--curved-line/--no-curvedline",
|
||||
"-cl/-nocl",
|
||||
is_flag=True,
|
||||
help="if this parameter set to true, this tool will try to return contoure of textlines instead of rectangle bounding box of textline. This should be taken into account that with this option the tool need more time to do process.",
|
||||
)
|
||||
@click.option(
|
||||
"--textline_light/--no-textline_light",
|
||||
"-tll/-notll",
|
||||
is_flag=True,
|
||||
help="if this parameter set to true, this tool will try to return contoure of textlines instead of rectangle bounding box of textline with a faster method.",
|
||||
)
|
||||
@click.option(
|
||||
"--full-layout/--no-full-layout",
|
||||
"-fl/-nofl",
|
||||
is_flag=True,
|
||||
help="if this parameter set to true, this tool will try to return all elements of layout.",
|
||||
)
|
||||
@click.option(
|
||||
"--tables/--no-tables",
|
||||
"-tab/-notab",
|
||||
is_flag=True,
|
||||
help="if this parameter set to true, this tool will try to detect tables.",
|
||||
)
|
||||
@click.option(
|
||||
"--right2left/--left2right",
|
||||
"-r2l/-l2r",
|
||||
is_flag=True,
|
||||
help="if this parameter set to true, this tool will extract right-to-left reading order.",
|
||||
)
|
||||
@click.option(
|
||||
"--input_binary/--input-RGB",
|
||||
"-ib/-irgb",
|
||||
is_flag=True,
|
||||
help="in general, eynollah uses RGB as input but if the input document is strongly dark, bright or for any other reason you can turn binarized input on. This option does not mean that you have to provide a binary image, otherwise this means that the tool itself will binarized the RGB input document.",
|
||||
)
|
||||
@click.option(
|
||||
"--allow_scaling/--no-allow-scaling",
|
||||
"-as/-noas",
|
||||
is_flag=True,
|
||||
help="if this parameter set to true, this tool would check the scale and if needed it will scale it to perform better layout detection",
|
||||
)
|
||||
@click.option(
|
||||
"--headers_off/--headers-on",
|
||||
"-ho/-noho",
|
||||
is_flag=True,
|
||||
help="if this parameter set to true, this tool would ignore headers role in reading order",
|
||||
)
|
||||
@click.option(
|
||||
"--light_version/--original",
|
||||
"-light/-org",
|
||||
is_flag=True,
|
||||
help="if this parameter set to true, this tool would use lighter version",
|
||||
)
|
||||
@click.option(
|
||||
"--ignore_page_extraction/--extract_page_included",
|
||||
"-ipe/-epi",
|
||||
is_flag=True,
|
||||
help="if this parameter set to true, this tool would ignore page extraction",
|
||||
)
|
||||
@click.option(
|
||||
"--reading_order_machine_based/--heuristic_reading_order",
|
||||
"-romb/-hro",
|
||||
is_flag=True,
|
||||
help="if this parameter set to true, this tool would apply machine based reading order detection",
|
||||
)
|
||||
@click.option(
|
||||
"--do_ocr",
|
||||
"-ocr/-noocr",
|
||||
is_flag=True,
|
||||
help="if this parameter set to true, this tool will try to do ocr",
|
||||
)
|
||||
@click.option(
|
||||
"--num_col_upper",
|
||||
"-ncu",
|
||||
help="lower limit of columns in document image",
|
||||
)
|
||||
@click.option(
|
||||
"--num_col_lower",
|
||||
"-ncl",
|
||||
help="upper limit of columns in document image",
|
||||
)
|
||||
@click.option(
|
||||
"--skip_layout_and_reading_order",
|
||||
"-slro/-noslro",
|
||||
is_flag=True,
|
||||
help="if this parameter set to true, this tool will ignore layout detection and reading order. It means that textline detection will be done within printspace and contours of textline will be written in xml output file.",
|
||||
)
|
||||
@click.option(
|
||||
"--log_level",
|
||||
"-l",
|
||||
type=click.Choice(['OFF', 'DEBUG', 'INFO', 'WARN', 'ERROR']),
|
||||
help="Override log level globally to this",
|
||||
)
|
||||
|
||||
def layout(image, out, overwrite, dir_in, model, save_images, save_layout, save_deskewed, save_all, extract_only_images, save_page, enable_plotting, allow_enhancement, curved_line, textline_light, full_layout, tables, right2left, input_binary, allow_scaling, headers_off, light_version, reading_order_machine_based, do_ocr, num_col_upper, num_col_lower, skip_layout_and_reading_order, ignore_page_extraction, log_level):
|
||||
initLogging()
|
||||
if log_level:
|
||||
getLogger('eynollah').setLevel(getLevelName(log_level))
|
||||
assert enable_plotting or not save_layout, "Plotting with -sl also requires -ep"
|
||||
assert enable_plotting or not save_deskewed, "Plotting with -sd also requires -ep"
|
||||
assert enable_plotting or not save_all, "Plotting with -sa also requires -ep"
|
||||
assert enable_plotting or not save_page, "Plotting with -sp also requires -ep"
|
||||
assert enable_plotting or not save_images, "Plotting with -si also requires -ep"
|
||||
assert enable_plotting or not allow_enhancement, "Plotting with -ae also requires -ep"
|
||||
assert not enable_plotting or save_layout or save_deskewed or save_all or save_page or save_images or allow_enhancement, \
|
||||
"Plotting with -ep also requires -sl, -sd, -sa, -sp, -si or -ae"
|
||||
assert textline_light == light_version, "Both light textline detection -tll and light version -light must be set or unset equally"
|
||||
assert not extract_only_images or not allow_enhancement, "Image extraction -eoi can not be set alongside allow_enhancement -ae"
|
||||
assert not extract_only_images or not allow_scaling, "Image extraction -eoi can not be set alongside allow_scaling -as"
|
||||
assert not extract_only_images or not light_version, "Image extraction -eoi can not be set alongside light_version -light"
|
||||
assert not extract_only_images or not curved_line, "Image extraction -eoi can not be set alongside curved_line -cl"
|
||||
assert not extract_only_images or not textline_light, "Image extraction -eoi can not be set alongside textline_light -tll"
|
||||
assert not extract_only_images or not full_layout, "Image extraction -eoi can not be set alongside full_layout -fl"
|
||||
assert not extract_only_images or not tables, "Image extraction -eoi can not be set alongside tables -tab"
|
||||
assert not extract_only_images or not right2left, "Image extraction -eoi can not be set alongside right2left -r2l"
|
||||
assert not extract_only_images or not headers_off, "Image extraction -eoi can not be set alongside headers_off -ho"
|
||||
assert image or dir_in, "Either a single image -i or a dir_in -di is required"
|
||||
eynollah = Eynollah(
|
||||
model,
|
||||
logger=getLogger('eynollah'),
|
||||
dir_out=out,
|
||||
dir_of_cropped_images=save_images,
|
||||
extract_only_images=extract_only_images,
|
||||
dir_of_layout=save_layout,
|
||||
dir_of_deskewed=save_deskewed,
|
||||
dir_of_all=save_all,
|
||||
dir_save_page=save_page,
|
||||
enable_plotting=enable_plotting,
|
||||
allow_enhancement=allow_enhancement,
|
||||
curved_line=curved_line,
|
||||
textline_light=textline_light,
|
||||
full_layout=full_layout,
|
||||
tables=tables,
|
||||
right2left=right2left,
|
||||
input_binary=input_binary,
|
||||
allow_scaling=allow_scaling,
|
||||
headers_off=headers_off,
|
||||
light_version=light_version,
|
||||
ignore_page_extraction=ignore_page_extraction,
|
||||
reading_order_machine_based=reading_order_machine_based,
|
||||
do_ocr=do_ocr,
|
||||
num_col_upper=num_col_upper,
|
||||
num_col_lower=num_col_lower,
|
||||
skip_layout_and_reading_order=skip_layout_and_reading_order,
|
||||
)
|
||||
if dir_in:
|
||||
eynollah.run(dir_in=dir_in, overwrite=overwrite)
|
||||
else:
|
||||
eynollah.run(image_filename=image, overwrite=overwrite)
|
||||
|
||||
|
||||
@main.command()
|
||||
@click.option(
|
||||
"--dir_in",
|
||||
"-di",
|
||||
help="directory of images",
|
||||
type=click.Path(exists=True, file_okay=False),
|
||||
)
|
||||
@click.option(
|
||||
"--dir_in_bin",
|
||||
"-dib",
|
||||
help="directory of binarized images. This should be given if you want to do prediction based on both rgb and bin images. And all bin images are png files",
|
||||
type=click.Path(exists=True, file_okay=False),
|
||||
)
|
||||
@click.option(
|
||||
"--out",
|
||||
"-o",
|
||||
help="directory to write output xml data",
|
||||
type=click.Path(exists=True, file_okay=False),
|
||||
required=True,
|
||||
)
|
||||
@click.option(
|
||||
"--dir_xmls",
|
||||
"-dx",
|
||||
help="directory of xmls",
|
||||
type=click.Path(exists=True, file_okay=False),
|
||||
)
|
||||
@click.option(
|
||||
"--dir_out_image_text",
|
||||
"-doit",
|
||||
help="directory of images with predicted text",
|
||||
type=click.Path(exists=True, file_okay=False),
|
||||
)
|
||||
@click.option(
|
||||
"--model",
|
||||
"-m",
|
||||
help="directory of models",
|
||||
type=click.Path(exists=True, file_okay=False),
|
||||
required=True,
|
||||
)
|
||||
@click.option(
|
||||
"--tr_ocr",
|
||||
"-trocr/-notrocr",
|
||||
is_flag=True,
|
||||
help="if this parameter set to true, transformer ocr will be applied, otherwise cnn_rnn model.",
|
||||
)
|
||||
@click.option(
|
||||
"--export_textline_images_and_text",
|
||||
"-etit/-noetit",
|
||||
is_flag=True,
|
||||
help="if this parameter set to true, images and text in xml will be exported into output dir. This files can be used for training a OCR engine.",
|
||||
)
|
||||
@click.option(
|
||||
"--do_not_mask_with_textline_contour",
|
||||
"-nmtc/-mtc",
|
||||
is_flag=True,
|
||||
help="if this parameter set to true, cropped textline images will not be masked with textline contour.",
|
||||
)
|
||||
@click.option(
|
||||
"--draw_texts_on_image",
|
||||
"-dtoi/-ndtoi",
|
||||
is_flag=True,
|
||||
help="if this parameter set to true, the predicted texts will be displayed on an image.",
|
||||
)
|
||||
@click.option(
|
||||
"--prediction_with_both_of_rgb_and_bin",
|
||||
"-brb/-nbrb",
|
||||
is_flag=True,
|
||||
help="If this parameter is set to True, the prediction will be performed using both RGB and binary images. However, this does not necessarily improve results; it may be beneficial for certain document images.",
|
||||
)
|
||||
@click.option(
|
||||
"--log_level",
|
||||
"-l",
|
||||
type=click.Choice(['OFF', 'DEBUG', 'INFO', 'WARN', 'ERROR']),
|
||||
help="Override log level globally to this",
|
||||
)
|
||||
|
||||
def ocr(dir_in, dir_in_bin, out, dir_xmls, dir_out_image_text, model, tr_ocr, export_textline_images_and_text, do_not_mask_with_textline_contour, draw_texts_on_image, prediction_with_both_of_rgb_and_bin, log_level):
|
||||
initLogging()
|
||||
if log_level:
|
||||
getLogger('eynollah').setLevel(getLevelName(log_level))
|
||||
eynollah_ocr = Eynollah_ocr(
|
||||
dir_xmls=dir_xmls,
|
||||
dir_out_image_text=dir_out_image_text,
|
||||
dir_in=dir_in,
|
||||
dir_in_bin=dir_in_bin,
|
||||
dir_out=out,
|
||||
dir_models=model,
|
||||
tr_ocr=tr_ocr,
|
||||
export_textline_images_and_text=export_textline_images_and_text,
|
||||
do_not_mask_with_textline_contour=do_not_mask_with_textline_contour,
|
||||
draw_texts_on_image=draw_texts_on_image,
|
||||
prediction_with_both_of_rgb_and_bin=prediction_with_both_of_rgb_and_bin,
|
||||
)
|
||||
eynollah_ocr.run()
|
||||
|
||||
if __name__ == "__main__":
|
||||
main()
|
File diff suppressed because it is too large
Load diff
|
@ -1,137 +0,0 @@
|
|||
{
|
||||
"version": "0.4.0",
|
||||
"git_url": "https://github.com/qurator-spk/eynollah",
|
||||
"dockerhub": "ocrd/eynollah",
|
||||
"tools": {
|
||||
"ocrd-eynollah-segment": {
|
||||
"executable": "ocrd-eynollah-segment",
|
||||
"categories": ["Layout analysis"],
|
||||
"description": "Segment page into regions and lines and do reading order detection with eynollah",
|
||||
"input_file_grp_cardinality": 1,
|
||||
"output_file_grp_cardinality": 1,
|
||||
"steps": ["layout/segmentation/region", "layout/segmentation/line"],
|
||||
"parameters": {
|
||||
"models": {
|
||||
"type": "string",
|
||||
"format": "uri",
|
||||
"content-type": "text/directory",
|
||||
"cacheable": true,
|
||||
"description": "Directory containing models to be used (See https://qurator-data.de/eynollah)",
|
||||
"required": true
|
||||
},
|
||||
"dpi": {
|
||||
"type": "number",
|
||||
"format": "float",
|
||||
"description": "pixel density in dots per inch (overrides any meta-data in the images); ignored if <= 0 (with fall-back 230)",
|
||||
"default": 0
|
||||
},
|
||||
"full_layout": {
|
||||
"type": "boolean",
|
||||
"default": true,
|
||||
"description": "Try to detect all element subtypes, including drop-caps and headings"
|
||||
},
|
||||
"light_version": {
|
||||
"type": "boolean",
|
||||
"default": true,
|
||||
"description": "Try to detect all element subtypes in light version (faster+simpler method for main region detection and deskewing)"
|
||||
},
|
||||
"textline_light": {
|
||||
"type": "boolean",
|
||||
"default": true,
|
||||
"description": "Light version need textline light"
|
||||
},
|
||||
"tables": {
|
||||
"type": "boolean",
|
||||
"default": false,
|
||||
"description": "Try to detect table regions"
|
||||
},
|
||||
"curved_line": {
|
||||
"type": "boolean",
|
||||
"default": false,
|
||||
"description": "try to return contour of textlines instead of just rectangle bounding box. Needs more processing time"
|
||||
},
|
||||
"ignore_page_extraction": {
|
||||
"type": "boolean",
|
||||
"default": false,
|
||||
"description": "if this parameter set to true, this tool would ignore page extraction"
|
||||
},
|
||||
"allow_scaling": {
|
||||
"type": "boolean",
|
||||
"default": false,
|
||||
"description": "check the resolution against the number of detected columns and if needed, scale the image up or down during layout detection (heuristic to improve quality and performance)"
|
||||
},
|
||||
"allow_enhancement": {
|
||||
"type": "boolean",
|
||||
"default": false,
|
||||
"description": "if this parameter set to true, this tool would check that input image need resizing and enhancement or not."
|
||||
},
|
||||
"textline_light": {
|
||||
"type": "boolean",
|
||||
"default": false,
|
||||
"description": "if this parameter set to true, this tool will try to return contoure of textlines instead of rectangle bounding box of textline with a faster method."
|
||||
},
|
||||
"right_to_left": {
|
||||
"type": "boolean",
|
||||
"default": false,
|
||||
"description": "if this parameter set to true, this tool will extract right-to-left reading order."
|
||||
},
|
||||
"headers_off": {
|
||||
"type": "boolean",
|
||||
"default": false,
|
||||
"description": "ignore the special role of headings during reading order detection"
|
||||
}
|
||||
},
|
||||
"resources": [
|
||||
{
|
||||
"description": "models for eynollah (TensorFlow SavedModel format)",
|
||||
"url": "https://github.com/qurator-spk/eynollah/releases/download/v0.3.1/models_eynollah.tar.gz",
|
||||
"name": "default",
|
||||
"size": 1894627041,
|
||||
"type": "archive",
|
||||
"path_in_archive": "models_eynollah"
|
||||
}
|
||||
]
|
||||
},
|
||||
"ocrd-sbb-binarize": {
|
||||
"executable": "ocrd-sbb-binarize",
|
||||
"description": "Pixelwise binarization with selectional auto-encoders in Keras",
|
||||
"categories": ["Image preprocessing"],
|
||||
"steps": ["preprocessing/optimization/binarization"],
|
||||
"input_file_grp_cardinality": 1,
|
||||
"output_file_grp_cardinality": 1,
|
||||
"parameters": {
|
||||
"operation_level": {
|
||||
"type": "string",
|
||||
"enum": ["page", "region"],
|
||||
"default": "page",
|
||||
"description": "PAGE XML hierarchy level to operate on"
|
||||
},
|
||||
"model": {
|
||||
"description": "Directory containing HDF5 or SavedModel/ProtoBuf models. Can be an absolute path or a path relative to the OCR-D resource location, the current working directory or the $SBB_BINARIZE_DATA environment variable (if set)",
|
||||
"type": "string",
|
||||
"format": "uri",
|
||||
"content-type": "text/directory",
|
||||
"required": true
|
||||
}
|
||||
},
|
||||
"resources": [
|
||||
{
|
||||
"url": "https://github.com/qurator-spk/sbb_binarization/releases/download/v0.0.11/saved_model_2020_01_16.zip",
|
||||
"name": "default",
|
||||
"type": "archive",
|
||||
"path_in_archive": "saved_model_2020_01_16",
|
||||
"size": 563147331,
|
||||
"description": "default models provided by github.com/qurator-spk (SavedModel format)"
|
||||
},
|
||||
{
|
||||
"url": "https://github.com/qurator-spk/sbb_binarization/releases/download/v0.0.11/saved_model_2021_03_09.zip",
|
||||
"name": "default-2021-03-09",
|
||||
"type": "archive",
|
||||
"path_in_archive": ".",
|
||||
"size": 133230419,
|
||||
"description": "updated default models provided by github.com/qurator-spk (SavedModel format)"
|
||||
}
|
||||
]
|
||||
}
|
||||
}
|
||||
}
|
|
@ -1,109 +0,0 @@
|
|||
from typing import Optional
|
||||
|
||||
from PIL import Image
|
||||
import numpy as np
|
||||
import cv2
|
||||
from click import command
|
||||
|
||||
from ocrd import Processor, OcrdPageResult, OcrdPageResultImage
|
||||
from ocrd_models.ocrd_page import OcrdPage, AlternativeImageType
|
||||
from ocrd.decorators import ocrd_cli_options, ocrd_cli_wrap_processor
|
||||
|
||||
from .sbb_binarize import SbbBinarizer
|
||||
|
||||
|
||||
def cv2pil(img):
|
||||
return Image.fromarray(img.astype('uint8'))
|
||||
|
||||
def pil2cv(img):
|
||||
# from ocrd/workspace.py
|
||||
color_conversion = cv2.COLOR_GRAY2BGR if img.mode in ('1', 'L') else cv2.COLOR_RGB2BGR
|
||||
pil_as_np_array = np.array(img).astype('uint8') if img.mode == '1' else np.array(img)
|
||||
return cv2.cvtColor(pil_as_np_array, color_conversion)
|
||||
|
||||
class SbbBinarizeProcessor(Processor):
|
||||
# already employs GPU (without singleton process atm)
|
||||
max_workers = 1
|
||||
|
||||
@property
|
||||
def executable(self):
|
||||
return 'ocrd-sbb-binarize'
|
||||
|
||||
def setup(self):
|
||||
"""
|
||||
Set up the model prior to processing.
|
||||
"""
|
||||
# resolve relative path via OCR-D ResourceManager
|
||||
model_path = self.resolve_resource(self.parameter['model'])
|
||||
self.binarizer = SbbBinarizer(model_dir=model_path, logger=self.logger)
|
||||
|
||||
def process_page_pcgts(self, *input_pcgts: Optional[OcrdPage], page_id: Optional[str] = None) -> OcrdPageResult:
|
||||
"""
|
||||
Binarize images with sbb_binarization (based on selectional auto-encoders).
|
||||
|
||||
For each page of the input file group, open and deserialize input PAGE-XML
|
||||
and its respective images. Then iterate over the element hierarchy down to
|
||||
the requested ``operation_level``.
|
||||
|
||||
For each segment element, retrieve a raw (non-binarized) segment image
|
||||
according to the layout annotation (from an existing ``AlternativeImage``,
|
||||
or by cropping into the higher-level images, and deskewing when applicable).
|
||||
|
||||
Pass the image to the binarizer (which runs in fixed-size windows/patches
|
||||
across the image and stitches the results together).
|
||||
|
||||
Serialize the resulting bilevel image as PNG file and add it to the output
|
||||
file group (with file ID suffix ``.IMG-BIN``) along with the output PAGE-XML
|
||||
(referencing it as new ``AlternativeImage`` for the segment element).
|
||||
|
||||
Produce a new PAGE output file by serialising the resulting hierarchy.
|
||||
"""
|
||||
assert input_pcgts
|
||||
assert input_pcgts[0]
|
||||
assert self.parameter
|
||||
oplevel = self.parameter['operation_level']
|
||||
pcgts = input_pcgts[0]
|
||||
result = OcrdPageResult(pcgts)
|
||||
page = pcgts.get_Page()
|
||||
page_image, page_xywh, _ = self.workspace.image_from_page(
|
||||
page, page_id, feature_filter='binarized')
|
||||
|
||||
if oplevel == 'page':
|
||||
self.logger.info("Binarizing on 'page' level in page '%s'", page_id)
|
||||
page_image_bin = cv2pil(self.binarizer.run(image=pil2cv(page_image), use_patches=True))
|
||||
# update PAGE (reference the image file):
|
||||
page_image_ref = AlternativeImageType(comments=page_xywh['features'] + ',binarized,clipped')
|
||||
page.add_AlternativeImage(page_image_ref)
|
||||
result.images.append(OcrdPageResultImage(page_image_bin, '.IMG-BIN', page_image_ref))
|
||||
|
||||
elif oplevel == 'region':
|
||||
regions = page.get_AllRegions(['Text', 'Table'], depth=1)
|
||||
if not regions:
|
||||
self.logger.warning("Page '%s' contains no text/table regions", page_id)
|
||||
for region in regions:
|
||||
region_image, region_xywh = self.workspace.image_from_segment(
|
||||
region, page_image, page_xywh, feature_filter='binarized')
|
||||
region_image_bin = cv2pil(self.binarizer.run(image=pil2cv(region_image), use_patches=True))
|
||||
# update PAGE (reference the image file):
|
||||
region_image_ref = AlternativeImageType(comments=region_xywh['features'] + ',binarized')
|
||||
region.add_AlternativeImage(region_image_ref)
|
||||
result.images.append(OcrdPageResultImage(region_image_bin, region.id + '.IMG-BIN', region_image_ref))
|
||||
|
||||
elif oplevel == 'line':
|
||||
lines = page.get_AllTextLines()
|
||||
if not lines:
|
||||
self.logger.warning("Page '%s' contains no text lines", page_id)
|
||||
for line in lines:
|
||||
line_image, line_xywh = self.workspace.image_from_segment(line, page_image, page_xywh, feature_filter='binarized')
|
||||
line_image_bin = cv2pil(self.binarizer.run(image=pil2cv(line_image), use_patches=True))
|
||||
# update PAGE (reference the image file):
|
||||
line_image_ref = AlternativeImageType(comments=line_xywh['features'] + ',binarized')
|
||||
line.add_AlternativeImage(region_image_ref)
|
||||
result.images.append(OcrdPageResultImage(line_image_bin, line.id + '.IMG-BIN', line_image_ref))
|
||||
|
||||
return result
|
||||
|
||||
@command()
|
||||
@ocrd_cli_options
|
||||
def main(*args, **kwargs):
|
||||
return ocrd_cli_wrap_processor(SbbBinarizeProcessor, *args, **kwargs)
|
|
@ -1,91 +0,0 @@
|
|||
from typing import Optional
|
||||
from ocrd_models import OcrdPage
|
||||
from ocrd import Processor, OcrdPageResult
|
||||
|
||||
from .eynollah import Eynollah, EynollahXmlWriter
|
||||
|
||||
class EynollahProcessor(Processor):
|
||||
# already employs background CPU multiprocessing per page
|
||||
# already employs GPU (without singleton process atm)
|
||||
max_workers = 1
|
||||
|
||||
@property
|
||||
def executable(self):
|
||||
return 'ocrd-eynollah-segment'
|
||||
|
||||
def setup(self) -> None:
|
||||
if self.parameter['textline_light'] and not self.parameter['light_version']:
|
||||
raise ValueError("Error: You set parameter 'textline_light' to enable light textline detection, "
|
||||
"but parameter 'light_version' is not enabled")
|
||||
self.eynollah = Eynollah(
|
||||
self.resolve_resource(self.parameter['models']),
|
||||
logger=self.logger,
|
||||
allow_enhancement=self.parameter['allow_enhancement'],
|
||||
curved_line=self.parameter['curved_line'],
|
||||
right2left=self.parameter['right_to_left'],
|
||||
ignore_page_extraction=self.parameter['ignore_page_extraction'],
|
||||
light_version=self.parameter['light_version'],
|
||||
textline_light=self.parameter['textline_light'],
|
||||
full_layout=self.parameter['full_layout'],
|
||||
allow_scaling=self.parameter['allow_scaling'],
|
||||
headers_off=self.parameter['headers_off'],
|
||||
tables=self.parameter['tables'],
|
||||
)
|
||||
self.eynollah.plotter = None
|
||||
|
||||
def shutdown(self):
|
||||
if hasattr(self, 'eynollah'):
|
||||
del self.eynollah
|
||||
|
||||
def process_page_pcgts(self, *input_pcgts: Optional[OcrdPage], page_id: Optional[str] = None) -> OcrdPageResult:
|
||||
"""
|
||||
Performs cropping, region and line segmentation with Eynollah.
|
||||
|
||||
For each page, open and deserialize PAGE input file (from existing
|
||||
PAGE file in the input fileGrp, or generated from image file).
|
||||
Retrieve its respective page-level image (ignoring annotation that
|
||||
already added `binarized`, `cropped` or `deskewed` features).
|
||||
|
||||
Set up Eynollah to detect regions and lines, and add each one to the
|
||||
page, respectively.
|
||||
|
||||
\b
|
||||
- If ``tables``, try to detect table blocks and add them as TableRegion.
|
||||
- If ``full_layout``, then in addition to paragraphs and marginals, also
|
||||
try to detect drop capitals and headings.
|
||||
- If ``ignore_page_extraction``, then attempt no cropping of the page.
|
||||
- If ``curved_line``, then compute contour polygons for text lines
|
||||
instead of simple bounding boxes.
|
||||
|
||||
Produce a new output file by serialising the resulting hierarchy.
|
||||
"""
|
||||
assert input_pcgts
|
||||
assert input_pcgts[0]
|
||||
assert self.parameter
|
||||
pcgts = input_pcgts[0]
|
||||
result = OcrdPageResult(pcgts)
|
||||
page = pcgts.get_Page()
|
||||
page_image, _, _ = self.workspace.image_from_page(
|
||||
page, page_id,
|
||||
# avoid any features that would change the coordinate system: cropped,deskewed
|
||||
# (the PAGE builder merely adds regions, so afterwards we would not know which to transform)
|
||||
# also avoid binarization as models usually fare better on grayscale/RGB
|
||||
feature_filter='cropped,deskewed,binarized')
|
||||
if hasattr(page_image, 'filename'):
|
||||
image_filename = page_image.filename
|
||||
else:
|
||||
image_filename = "dummy" # will be replaced by ocrd.Processor.process_page_file
|
||||
result.images.append(OcrdPageResultImage(page_image, '.IMG', page)) # mark as new original
|
||||
# FIXME: mask out already existing regions (incremental segmentation)
|
||||
self.eynollah.cache_images(
|
||||
image_pil=page_image,
|
||||
dpi=self.parameter['dpi'],
|
||||
)
|
||||
self.eynollah.writer = EynollahXmlWriter(
|
||||
dir_out=None,
|
||||
image_filename=image_filename,
|
||||
curved_line=self.eynollah.curved_line,
|
||||
textline_light=self.eynollah.textline_light,
|
||||
pcgts=pcgts)
|
||||
self.eynollah.run_single()
|
||||
return result
|
|
@ -1,377 +0,0 @@
|
|||
"""
|
||||
Tool to load model and binarize a given image.
|
||||
"""
|
||||
|
||||
import sys
|
||||
from glob import glob
|
||||
import os
|
||||
import logging
|
||||
|
||||
import numpy as np
|
||||
from PIL import Image
|
||||
import cv2
|
||||
from ocrd_utils import tf_disable_interactive_logs
|
||||
tf_disable_interactive_logs()
|
||||
import tensorflow as tf
|
||||
from tensorflow.keras.models import load_model
|
||||
from tensorflow.python.keras import backend as tensorflow_backend
|
||||
|
||||
|
||||
def resize_image(img_in, input_height, input_width):
|
||||
return cv2.resize(img_in, (input_width, input_height), interpolation=cv2.INTER_NEAREST)
|
||||
|
||||
class SbbBinarizer:
|
||||
|
||||
def __init__(self, model_dir, logger=None):
|
||||
self.model_dir = model_dir
|
||||
self.log = logger if logger else logging.getLogger('SbbBinarizer')
|
||||
|
||||
self.start_new_session()
|
||||
|
||||
self.model_files = glob(self.model_dir+"/*/", recursive = True)
|
||||
|
||||
self.models = []
|
||||
for model_file in self.model_files:
|
||||
self.models.append(self.load_model(model_file))
|
||||
|
||||
def start_new_session(self):
|
||||
config = tf.compat.v1.ConfigProto()
|
||||
config.gpu_options.allow_growth = True
|
||||
|
||||
self.session = tf.compat.v1.Session(config=config) # tf.InteractiveSession()
|
||||
tensorflow_backend.set_session(self.session)
|
||||
|
||||
def end_session(self):
|
||||
tensorflow_backend.clear_session()
|
||||
self.session.close()
|
||||
del self.session
|
||||
|
||||
def load_model(self, model_name):
|
||||
model = load_model(os.path.join(self.model_dir, model_name), compile=False)
|
||||
model_height = model.layers[len(model.layers)-1].output_shape[1]
|
||||
model_width = model.layers[len(model.layers)-1].output_shape[2]
|
||||
n_classes = model.layers[len(model.layers)-1].output_shape[3]
|
||||
return model, model_height, model_width, n_classes
|
||||
|
||||
def predict(self, model_in, img, use_patches, n_batch_inference=5):
|
||||
tensorflow_backend.set_session(self.session)
|
||||
model, model_height, model_width, n_classes = model_in
|
||||
|
||||
img_org_h = img.shape[0]
|
||||
img_org_w = img.shape[1]
|
||||
|
||||
if img.shape[0] < model_height and img.shape[1] >= model_width:
|
||||
img_padded = np.zeros(( model_height, img.shape[1], img.shape[2] ))
|
||||
|
||||
index_start_h = int( abs( img.shape[0] - model_height) /2.)
|
||||
index_start_w = 0
|
||||
|
||||
img_padded [ index_start_h: index_start_h+img.shape[0], :, : ] = img[:,:,:]
|
||||
|
||||
elif img.shape[0] >= model_height and img.shape[1] < model_width:
|
||||
img_padded = np.zeros(( img.shape[0], model_width, img.shape[2] ))
|
||||
|
||||
index_start_h = 0
|
||||
index_start_w = int( abs( img.shape[1] - model_width) /2.)
|
||||
|
||||
img_padded [ :, index_start_w: index_start_w+img.shape[1], : ] = img[:,:,:]
|
||||
|
||||
|
||||
elif img.shape[0] < model_height and img.shape[1] < model_width:
|
||||
img_padded = np.zeros(( model_height, model_width, img.shape[2] ))
|
||||
|
||||
index_start_h = int( abs( img.shape[0] - model_height) /2.)
|
||||
index_start_w = int( abs( img.shape[1] - model_width) /2.)
|
||||
|
||||
img_padded [ index_start_h: index_start_h+img.shape[0], index_start_w: index_start_w+img.shape[1], : ] = img[:,:,:]
|
||||
|
||||
else:
|
||||
index_start_h = 0
|
||||
index_start_w = 0
|
||||
img_padded = np.copy(img)
|
||||
|
||||
|
||||
img = np.copy(img_padded)
|
||||
|
||||
|
||||
|
||||
if use_patches:
|
||||
|
||||
margin = int(0.1 * model_width)
|
||||
|
||||
width_mid = model_width - 2 * margin
|
||||
height_mid = model_height - 2 * margin
|
||||
|
||||
|
||||
img = img / float(255.0)
|
||||
|
||||
img_h = img.shape[0]
|
||||
img_w = img.shape[1]
|
||||
|
||||
prediction_true = np.zeros((img_h, img_w, 3))
|
||||
mask_true = np.zeros((img_h, img_w))
|
||||
nxf = img_w / float(width_mid)
|
||||
nyf = img_h / float(height_mid)
|
||||
|
||||
if nxf > int(nxf):
|
||||
nxf = int(nxf) + 1
|
||||
else:
|
||||
nxf = int(nxf)
|
||||
|
||||
if nyf > int(nyf):
|
||||
nyf = int(nyf) + 1
|
||||
else:
|
||||
nyf = int(nyf)
|
||||
|
||||
|
||||
list_i_s = []
|
||||
list_j_s = []
|
||||
list_x_u = []
|
||||
list_x_d = []
|
||||
list_y_u = []
|
||||
list_y_d = []
|
||||
|
||||
batch_indexer = 0
|
||||
|
||||
img_patch = np.zeros((n_batch_inference, model_height, model_width,3))
|
||||
|
||||
for i in range(nxf):
|
||||
for j in range(nyf):
|
||||
|
||||
if i == 0:
|
||||
index_x_d = i * width_mid
|
||||
index_x_u = index_x_d + model_width
|
||||
elif i > 0:
|
||||
index_x_d = i * width_mid
|
||||
index_x_u = index_x_d + model_width
|
||||
|
||||
if j == 0:
|
||||
index_y_d = j * height_mid
|
||||
index_y_u = index_y_d + model_height
|
||||
elif j > 0:
|
||||
index_y_d = j * height_mid
|
||||
index_y_u = index_y_d + model_height
|
||||
|
||||
if index_x_u > img_w:
|
||||
index_x_u = img_w
|
||||
index_x_d = img_w - model_width
|
||||
if index_y_u > img_h:
|
||||
index_y_u = img_h
|
||||
index_y_d = img_h - model_height
|
||||
|
||||
|
||||
list_i_s.append(i)
|
||||
list_j_s.append(j)
|
||||
list_x_u.append(index_x_u)
|
||||
list_x_d.append(index_x_d)
|
||||
list_y_d.append(index_y_d)
|
||||
list_y_u.append(index_y_u)
|
||||
|
||||
|
||||
img_patch[batch_indexer,:,:,:] = img[index_y_d:index_y_u, index_x_d:index_x_u, :]
|
||||
|
||||
batch_indexer = batch_indexer + 1
|
||||
|
||||
|
||||
|
||||
if batch_indexer == n_batch_inference:
|
||||
|
||||
label_p_pred = model.predict(img_patch,verbose=0)
|
||||
|
||||
seg = np.argmax(label_p_pred, axis=3)
|
||||
|
||||
#print(seg.shape, len(seg), len(list_i_s))
|
||||
|
||||
indexer_inside_batch = 0
|
||||
for i_batch, j_batch in zip(list_i_s, list_j_s):
|
||||
seg_in = seg[indexer_inside_batch,:,:]
|
||||
seg_color = np.repeat(seg_in[:, :, np.newaxis], 3, axis=2)
|
||||
|
||||
index_y_u_in = list_y_u[indexer_inside_batch]
|
||||
index_y_d_in = list_y_d[indexer_inside_batch]
|
||||
|
||||
index_x_u_in = list_x_u[indexer_inside_batch]
|
||||
index_x_d_in = list_x_d[indexer_inside_batch]
|
||||
|
||||
if i_batch == 0 and j_batch == 0:
|
||||
seg_color = seg_color[0 : seg_color.shape[0] - margin, 0 : seg_color.shape[1] - margin, :]
|
||||
prediction_true[index_y_d_in + 0 : index_y_u_in - margin, index_x_d_in + 0 : index_x_u_in - margin, :] = seg_color
|
||||
elif i_batch == nxf - 1 and j_batch == nyf - 1:
|
||||
seg_color = seg_color[margin : seg_color.shape[0] - 0, margin : seg_color.shape[1] - 0, :]
|
||||
prediction_true[index_y_d_in + margin : index_y_u_in - 0, index_x_d_in + margin : index_x_u_in - 0, :] = seg_color
|
||||
elif i_batch == 0 and j_batch == nyf - 1:
|
||||
seg_color = seg_color[margin : seg_color.shape[0] - 0, 0 : seg_color.shape[1] - margin, :]
|
||||
prediction_true[index_y_d_in + margin : index_y_u_in - 0, index_x_d_in + 0 : index_x_u_in - margin, :] = seg_color
|
||||
elif i_batch == nxf - 1 and j_batch == 0:
|
||||
seg_color = seg_color[0 : seg_color.shape[0] - margin, margin : seg_color.shape[1] - 0, :]
|
||||
prediction_true[index_y_d_in + 0 : index_y_u_in - margin, index_x_d_in + margin : index_x_u_in - 0, :] = seg_color
|
||||
elif i_batch == 0 and j_batch != 0 and j_batch != nyf - 1:
|
||||
seg_color = seg_color[margin : seg_color.shape[0] - margin, 0 : seg_color.shape[1] - margin, :]
|
||||
prediction_true[index_y_d_in + margin : index_y_u_in - margin, index_x_d_in + 0 : index_x_u_in - margin, :] = seg_color
|
||||
elif i_batch == nxf - 1 and j_batch != 0 and j_batch != nyf - 1:
|
||||
seg_color = seg_color[margin : seg_color.shape[0] - margin, margin : seg_color.shape[1] - 0, :]
|
||||
prediction_true[index_y_d_in + margin : index_y_u_in - margin, index_x_d_in + margin : index_x_u_in - 0, :] = seg_color
|
||||
elif i_batch != 0 and i_batch != nxf - 1 and j_batch == 0:
|
||||
seg_color = seg_color[0 : seg_color.shape[0] - margin, margin : seg_color.shape[1] - margin, :]
|
||||
prediction_true[index_y_d_in + 0 : index_y_u_in - margin, index_x_d_in + margin : index_x_u_in - margin, :] = seg_color
|
||||
elif i_batch != 0 and i_batch != nxf - 1 and j_batch == nyf - 1:
|
||||
seg_color = seg_color[margin : seg_color.shape[0] - 0, margin : seg_color.shape[1] - margin, :]
|
||||
prediction_true[index_y_d_in + margin : index_y_u_in - 0, index_x_d_in + margin : index_x_u_in - margin, :] = seg_color
|
||||
else:
|
||||
seg_color = seg_color[margin : seg_color.shape[0] - margin, margin : seg_color.shape[1] - margin, :]
|
||||
prediction_true[index_y_d_in + margin : index_y_u_in - margin, index_x_d_in + margin : index_x_u_in - margin, :] = seg_color
|
||||
|
||||
indexer_inside_batch = indexer_inside_batch +1
|
||||
|
||||
|
||||
list_i_s = []
|
||||
list_j_s = []
|
||||
list_x_u = []
|
||||
list_x_d = []
|
||||
list_y_u = []
|
||||
list_y_d = []
|
||||
|
||||
batch_indexer = 0
|
||||
|
||||
img_patch = np.zeros((n_batch_inference, model_height, model_width,3))
|
||||
|
||||
elif i==(nxf-1) and j==(nyf-1):
|
||||
label_p_pred = model.predict(img_patch,verbose=0)
|
||||
|
||||
seg = np.argmax(label_p_pred, axis=3)
|
||||
|
||||
#print(seg.shape, len(seg), len(list_i_s))
|
||||
|
||||
indexer_inside_batch = 0
|
||||
for i_batch, j_batch in zip(list_i_s, list_j_s):
|
||||
seg_in = seg[indexer_inside_batch,:,:]
|
||||
seg_color = np.repeat(seg_in[:, :, np.newaxis], 3, axis=2)
|
||||
|
||||
index_y_u_in = list_y_u[indexer_inside_batch]
|
||||
index_y_d_in = list_y_d[indexer_inside_batch]
|
||||
|
||||
index_x_u_in = list_x_u[indexer_inside_batch]
|
||||
index_x_d_in = list_x_d[indexer_inside_batch]
|
||||
|
||||
if i_batch == 0 and j_batch == 0:
|
||||
seg_color = seg_color[0 : seg_color.shape[0] - margin, 0 : seg_color.shape[1] - margin, :]
|
||||
prediction_true[index_y_d_in + 0 : index_y_u_in - margin, index_x_d_in + 0 : index_x_u_in - margin, :] = seg_color
|
||||
elif i_batch == nxf - 1 and j_batch == nyf - 1:
|
||||
seg_color = seg_color[margin : seg_color.shape[0] - 0, margin : seg_color.shape[1] - 0, :]
|
||||
prediction_true[index_y_d_in + margin : index_y_u_in - 0, index_x_d_in + margin : index_x_u_in - 0, :] = seg_color
|
||||
elif i_batch == 0 and j_batch == nyf - 1:
|
||||
seg_color = seg_color[margin : seg_color.shape[0] - 0, 0 : seg_color.shape[1] - margin, :]
|
||||
prediction_true[index_y_d_in + margin : index_y_u_in - 0, index_x_d_in + 0 : index_x_u_in - margin, :] = seg_color
|
||||
elif i_batch == nxf - 1 and j_batch == 0:
|
||||
seg_color = seg_color[0 : seg_color.shape[0] - margin, margin : seg_color.shape[1] - 0, :]
|
||||
prediction_true[index_y_d_in + 0 : index_y_u_in - margin, index_x_d_in + margin : index_x_u_in - 0, :] = seg_color
|
||||
elif i_batch == 0 and j_batch != 0 and j_batch != nyf - 1:
|
||||
seg_color = seg_color[margin : seg_color.shape[0] - margin, 0 : seg_color.shape[1] - margin, :]
|
||||
prediction_true[index_y_d_in + margin : index_y_u_in - margin, index_x_d_in + 0 : index_x_u_in - margin, :] = seg_color
|
||||
elif i_batch == nxf - 1 and j_batch != 0 and j_batch != nyf - 1:
|
||||
seg_color = seg_color[margin : seg_color.shape[0] - margin, margin : seg_color.shape[1] - 0, :]
|
||||
prediction_true[index_y_d_in + margin : index_y_u_in - margin, index_x_d_in + margin : index_x_u_in - 0, :] = seg_color
|
||||
elif i_batch != 0 and i_batch != nxf - 1 and j_batch == 0:
|
||||
seg_color = seg_color[0 : seg_color.shape[0] - margin, margin : seg_color.shape[1] - margin, :]
|
||||
prediction_true[index_y_d_in + 0 : index_y_u_in - margin, index_x_d_in + margin : index_x_u_in - margin, :] = seg_color
|
||||
elif i_batch != 0 and i_batch != nxf - 1 and j_batch == nyf - 1:
|
||||
seg_color = seg_color[margin : seg_color.shape[0] - 0, margin : seg_color.shape[1] - margin, :]
|
||||
prediction_true[index_y_d_in + margin : index_y_u_in - 0, index_x_d_in + margin : index_x_u_in - margin, :] = seg_color
|
||||
else:
|
||||
seg_color = seg_color[margin : seg_color.shape[0] - margin, margin : seg_color.shape[1] - margin, :]
|
||||
prediction_true[index_y_d_in + margin : index_y_u_in - margin, index_x_d_in + margin : index_x_u_in - margin, :] = seg_color
|
||||
|
||||
indexer_inside_batch = indexer_inside_batch +1
|
||||
|
||||
|
||||
list_i_s = []
|
||||
list_j_s = []
|
||||
list_x_u = []
|
||||
list_x_d = []
|
||||
list_y_u = []
|
||||
list_y_d = []
|
||||
|
||||
batch_indexer = 0
|
||||
|
||||
img_patch = np.zeros((n_batch_inference, model_height, model_width,3))
|
||||
|
||||
|
||||
|
||||
prediction_true = prediction_true[index_start_h: index_start_h+img_org_h, index_start_w: index_start_w+img_org_w,:]
|
||||
prediction_true = prediction_true.astype(np.uint8)
|
||||
|
||||
else:
|
||||
img_h_page = img.shape[0]
|
||||
img_w_page = img.shape[1]
|
||||
img = img / float(255.0)
|
||||
img = resize_image(img, model_height, model_width)
|
||||
|
||||
label_p_pred = model.predict(img.reshape(1, img.shape[0], img.shape[1], img.shape[2]))
|
||||
|
||||
seg = np.argmax(label_p_pred, axis=3)[0]
|
||||
seg_color = np.repeat(seg[:, :, np.newaxis], 3, axis=2)
|
||||
prediction_true = resize_image(seg_color, img_h_page, img_w_page)
|
||||
prediction_true = prediction_true.astype(np.uint8)
|
||||
return prediction_true[:,:,0]
|
||||
|
||||
def run(self, image=None, image_path=None, save=None, use_patches=False, dir_in=None, dir_out=None):
|
||||
print(dir_in,'dir_in')
|
||||
if not dir_in:
|
||||
if (image is not None and image_path is not None) or \
|
||||
(image is None and image_path is None):
|
||||
raise ValueError("Must pass either a opencv2 image or an image_path")
|
||||
if image_path is not None:
|
||||
image = cv2.imread(image_path)
|
||||
img_last = 0
|
||||
for n, (model, model_file) in enumerate(zip(self.models, self.model_files)):
|
||||
self.log.info('Predicting with model %s [%s/%s]' % (model_file, n + 1, len(self.model_files)))
|
||||
|
||||
res = self.predict(model, image, use_patches)
|
||||
|
||||
img_fin = np.zeros((res.shape[0], res.shape[1], 3))
|
||||
res[:, :][res[:, :] == 0] = 2
|
||||
res = res - 1
|
||||
res = res * 255
|
||||
img_fin[:, :, 0] = res
|
||||
img_fin[:, :, 1] = res
|
||||
img_fin[:, :, 2] = res
|
||||
|
||||
img_fin = img_fin.astype(np.uint8)
|
||||
img_fin = (res[:, :] == 0) * 255
|
||||
img_last = img_last + img_fin
|
||||
|
||||
kernel = np.ones((5, 5), np.uint8)
|
||||
img_last[:, :][img_last[:, :] > 0] = 255
|
||||
img_last = (img_last[:, :] == 0) * 255
|
||||
if save:
|
||||
cv2.imwrite(save, img_last)
|
||||
return img_last
|
||||
else:
|
||||
ls_imgs = os.listdir(dir_in)
|
||||
for image_name in ls_imgs:
|
||||
image_stem = image_name.split('.')[0]
|
||||
print(image_name,'image_name')
|
||||
image = cv2.imread(os.path.join(dir_in,image_name) )
|
||||
img_last = 0
|
||||
for n, (model, model_file) in enumerate(zip(self.models, self.model_files)):
|
||||
self.log.info('Predicting with model %s [%s/%s]' % (model_file, n + 1, len(self.model_files)))
|
||||
|
||||
res = self.predict(model, image, use_patches)
|
||||
|
||||
img_fin = np.zeros((res.shape[0], res.shape[1], 3))
|
||||
res[:, :][res[:, :] == 0] = 2
|
||||
res = res - 1
|
||||
res = res * 255
|
||||
img_fin[:, :, 0] = res
|
||||
img_fin[:, :, 1] = res
|
||||
img_fin[:, :, 2] = res
|
||||
|
||||
img_fin = img_fin.astype(np.uint8)
|
||||
img_fin = (res[:, :] == 0) * 255
|
||||
img_last = img_last + img_fin
|
||||
|
||||
kernel = np.ones((5, 5), np.uint8)
|
||||
img_last[:, :][img_last[:, :] > 0] = 255
|
||||
img_last = (img_last[:, :] == 0) * 255
|
||||
|
||||
cv2.imwrite(os.path.join(dir_out,image_stem+'.png'), img_last)
|
54
tests/base.py
Normal file
54
tests/base.py
Normal file
|
@ -0,0 +1,54 @@
|
|||
# pylint: disable=unused-import
|
||||
|
||||
from os.path import dirname, realpath
|
||||
from os import chdir
|
||||
import sys
|
||||
import logging
|
||||
import io
|
||||
import collections
|
||||
from unittest import TestCase as VanillaTestCase, skip, main as unittests_main
|
||||
import pytest
|
||||
from ocrd_utils import disableLogging, initLogging
|
||||
|
||||
def main(fn=None):
|
||||
if fn:
|
||||
sys.exit(pytest.main([fn]))
|
||||
else:
|
||||
unittests_main()
|
||||
|
||||
class TestCase(VanillaTestCase):
|
||||
|
||||
@classmethod
|
||||
def setUpClass(cls):
|
||||
chdir(dirname(realpath(__file__)) + '/..')
|
||||
|
||||
def setUp(self):
|
||||
disableLogging()
|
||||
initLogging()
|
||||
|
||||
class CapturingTestCase(TestCase):
|
||||
"""
|
||||
A TestCase that needs to capture stderr/stdout and invoke click CLI.
|
||||
"""
|
||||
|
||||
@pytest.fixture(autouse=True)
|
||||
def _setup_pytest_capfd(self, capfd):
|
||||
self.capfd = capfd
|
||||
|
||||
def invoke_cli(self, cli, args):
|
||||
"""
|
||||
Substitution for click.CliRunner.invooke that works together nicely
|
||||
with unittests/pytest capturing stdout/stderr.
|
||||
"""
|
||||
self.capture_out_err() # XXX snapshot just before executing the CLI
|
||||
code = 0
|
||||
sys.argv[1:] = args # XXX necessary because sys.argv reflects pytest args not cli args
|
||||
try:
|
||||
cli.main(args=args)
|
||||
except SystemExit as e:
|
||||
code = e.code
|
||||
out, err = self.capture_out_err()
|
||||
return code, out, err
|
||||
|
||||
def capture_out_err(self):
|
||||
return self.capfd.readouterr()
|
Binary file not shown.
|
@ -1,4 +1,5 @@
|
|||
from eynollah.utils.counter import EynollahIdCounter
|
||||
from tests.base import main
|
||||
from qurator.eynollah.utils.counter import EynollahIdCounter
|
||||
|
||||
def test_counter_string():
|
||||
c = EynollahIdCounter()
|
||||
|
@ -28,3 +29,5 @@ def test_counter_methods():
|
|||
c.inc('region', -9)
|
||||
assert c.get('region') == 1
|
||||
|
||||
if __name__ == '__main__':
|
||||
main(__file__)
|
||||
|
|
|
@ -1,8 +1,11 @@
|
|||
import cv2
|
||||
from pathlib import Path
|
||||
from eynollah.utils.pil_cv2 import check_dpi
|
||||
from qurator.eynollah.utils.pil_cv2 import check_dpi
|
||||
from tests.base import main
|
||||
|
||||
def test_dpi():
|
||||
fpath = str(Path(__file__).parent.joinpath('resources', 'kant_aufklaerung_1784_0020.tif'))
|
||||
assert 230 == check_dpi(cv2.imread(fpath))
|
||||
|
||||
if __name__ == '__main__':
|
||||
main(__file__)
|
||||
|
|
|
@ -1,132 +1,24 @@
|
|||
from os import environ
|
||||
from pathlib import Path
|
||||
import logging
|
||||
from PIL import Image
|
||||
from eynollah.cli import layout as layout_cli, binarization as binarization_cli
|
||||
from click.testing import CliRunner
|
||||
from ocrd_modelfactory import page_from_file
|
||||
from ocrd_models.constants import NAMESPACES as NS
|
||||
from ocrd_utils import pushd_popd
|
||||
from tests.base import CapturingTestCase as TestCase, main
|
||||
from qurator.eynollah.cli import main as eynollah_cli
|
||||
|
||||
testdir = Path(__file__).parent.resolve()
|
||||
|
||||
EYNOLLAH_MODELS = environ.get('EYNOLLAH_MODELS', str(testdir.joinpath('..', 'models_eynollah').resolve()))
|
||||
SBBBIN_MODELS = environ.get('SBBBIN_MODELS', str(testdir.joinpath('..', 'default-2021-03-09').resolve()))
|
||||
|
||||
def test_run_eynollah_layout_filename(tmp_path, subtests, pytestconfig, caplog):
|
||||
infile = testdir.joinpath('resources/kant_aufklaerung_1784_0020.tif')
|
||||
outfile = tmp_path / 'kant_aufklaerung_1784_0020.xml'
|
||||
args = [
|
||||
'-m', EYNOLLAH_MODELS,
|
||||
'-i', str(infile),
|
||||
'-o', str(outfile.parent),
|
||||
# subtests write to same location
|
||||
'--overwrite',
|
||||
]
|
||||
if pytestconfig.getoption('verbose') > 0:
|
||||
args.extend(['-l', 'DEBUG'])
|
||||
caplog.set_level(logging.INFO)
|
||||
def only_eynollah(logrec):
|
||||
return logrec.name == 'eynollah'
|
||||
runner = CliRunner()
|
||||
for options in [
|
||||
[], # defaults
|
||||
["--allow_scaling", "--curved-line"],
|
||||
["--allow_scaling", "--curved-line", "--full-layout"],
|
||||
["--allow_scaling", "--curved-line", "--full-layout", "--reading_order_machine_based"],
|
||||
["--allow_scaling", "--curved-line", "--full-layout", "--reading_order_machine_based",
|
||||
"--textline_light", "--light_version"],
|
||||
# -ep ...
|
||||
# -eoi ...
|
||||
# --do_ocr
|
||||
# --skip_layout_and_reading_order
|
||||
]:
|
||||
with subtests.test(#msg="test CLI",
|
||||
options=options):
|
||||
with caplog.filtering(only_eynollah):
|
||||
result = runner.invoke(layout_cli, args + options, catch_exceptions=False)
|
||||
print(result)
|
||||
assert result.exit_code == 0
|
||||
logmsgs = [logrec.message for logrec in caplog.records]
|
||||
assert str(infile) in logmsgs
|
||||
assert outfile.exists()
|
||||
tree = page_from_file(str(outfile)).etree
|
||||
regions = tree.xpath("//page:TextRegion", namespaces=NS)
|
||||
assert len(regions) >= 2, "result is inaccurate"
|
||||
regions = tree.xpath("//page:SeparatorRegion", namespaces=NS)
|
||||
assert len(regions) >= 2, "result is inaccurate"
|
||||
lines = tree.xpath("//page:TextLine", namespaces=NS)
|
||||
assert len(lines) == 31, "result is inaccurate" # 29 paragraph lines, 1 page and 1 catch-word line
|
||||
class TestEynollahRun(TestCase):
|
||||
|
||||
def test_run_eynollah_layout_directory(tmp_path, pytestconfig, caplog):
|
||||
indir = testdir.joinpath('resources')
|
||||
outdir = tmp_path
|
||||
args = [
|
||||
'-m', EYNOLLAH_MODELS,
|
||||
'-di', str(indir),
|
||||
'-o', str(outdir),
|
||||
]
|
||||
if pytestconfig.getoption('verbose') > 0:
|
||||
args.extend(['-l', 'DEBUG'])
|
||||
caplog.set_level(logging.INFO)
|
||||
def only_eynollah(logrec):
|
||||
return logrec.name == 'eynollah'
|
||||
runner = CliRunner()
|
||||
with caplog.filtering(only_eynollah):
|
||||
result = runner.invoke(layout_cli, args)
|
||||
print(result)
|
||||
assert result.exit_code == 0
|
||||
logmsgs = [logrec.message for logrec in caplog.records]
|
||||
assert len([logmsg for logmsg in logmsgs if logmsg.startswith('Job done in')]) == 2
|
||||
assert any(logmsg for logmsg in logmsgs if logmsg.startswith('All jobs done in'))
|
||||
assert len(list(outdir.iterdir())) == 2
|
||||
def test_full_run(self):
|
||||
with pushd_popd(tempdir=True) as tempdir:
|
||||
code, out, err = self.invoke_cli(eynollah_cli, [
|
||||
'-m', EYNOLLAH_MODELS,
|
||||
'-i', str(testdir.joinpath('resources/kant_aufklaerung_1784_0020.tif')),
|
||||
'-o', tempdir
|
||||
])
|
||||
print(code, out, err)
|
||||
assert not code
|
||||
|
||||
def test_run_eynollah_binarization_filename(tmp_path, subtests, pytestconfig, caplog):
|
||||
infile = testdir.joinpath('resources/kant_aufklaerung_1784_0020.tif')
|
||||
outfile = tmp_path.joinpath('kant_aufklaerung_1784_0020.png')
|
||||
args = [
|
||||
'-m', SBBBIN_MODELS,
|
||||
str(infile),
|
||||
str(outfile),
|
||||
]
|
||||
caplog.set_level(logging.INFO)
|
||||
def only_eynollah(logrec):
|
||||
return logrec.name == 'SbbBinarizer'
|
||||
runner = CliRunner()
|
||||
for options in [
|
||||
[], # defaults
|
||||
["--no-patches"],
|
||||
]:
|
||||
with subtests.test(#msg="test CLI",
|
||||
options=options):
|
||||
with caplog.filtering(only_eynollah):
|
||||
result = runner.invoke(binarization_cli, args + options)
|
||||
print(result)
|
||||
assert result.exit_code == 0
|
||||
logmsgs = [logrec.message for logrec in caplog.records]
|
||||
assert any(True for logmsg in logmsgs if logmsg.startswith('Predicting'))
|
||||
assert outfile.exists()
|
||||
with Image.open(infile) as original_img:
|
||||
original_size = original_img.size
|
||||
with Image.open(outfile) as binarized_img:
|
||||
binarized_size = binarized_img.size
|
||||
assert original_size == binarized_size
|
||||
|
||||
def test_run_eynollah_binarization_directory(tmp_path, subtests, pytestconfig, caplog):
|
||||
indir = testdir.joinpath('resources')
|
||||
outdir = tmp_path
|
||||
args = [
|
||||
'-m', SBBBIN_MODELS,
|
||||
'-di', str(indir),
|
||||
'-do', str(outdir),
|
||||
]
|
||||
caplog.set_level(logging.INFO)
|
||||
def only_eynollah(logrec):
|
||||
return logrec.name == 'SbbBinarizer'
|
||||
runner = CliRunner()
|
||||
with caplog.filtering(only_eynollah):
|
||||
result = runner.invoke(binarization_cli, args)
|
||||
print(result)
|
||||
assert result.exit_code == 0
|
||||
logmsgs = [logrec.message for logrec in caplog.records]
|
||||
assert len([logmsg for logmsg in logmsgs if logmsg.startswith('Predicting')]) == 2
|
||||
assert len(list(outdir.iterdir())) == 2
|
||||
if __name__ == '__main__':
|
||||
main(__file__)
|
||||
|
|
|
@ -1,7 +1,7 @@
|
|||
def test_utils_import():
|
||||
import eynollah.utils
|
||||
import eynollah.utils.contour
|
||||
import eynollah.utils.drop_capitals
|
||||
import eynollah.utils.drop_capitals
|
||||
import eynollah.utils.is_nan
|
||||
import eynollah.utils.rotate
|
||||
import qurator.eynollah.utils
|
||||
import qurator.eynollah.utils.contour
|
||||
import qurator.eynollah.utils.drop_capitals
|
||||
import qurator.eynollah.utils.drop_capitals
|
||||
import qurator.eynollah.utils.is_nan
|
||||
import qurator.eynollah.utils.rotate
|
||||
|
|
|
@ -1,4 +1,5 @@
|
|||
from eynollah.utils.xml import create_page_xml
|
||||
from pytest import main
|
||||
from qurator.eynollah.utils.xml import create_page_xml
|
||||
from ocrd_models.ocrd_page import to_xml
|
||||
|
||||
PAGE_2019 = 'http://schema.primaresearch.org/PAGE/gts/pagecontent/2019-07-15'
|
||||
|
@ -8,3 +9,6 @@ def test_create_xml():
|
|||
xmlstr = to_xml(pcgts)
|
||||
assert 'xmlns:pc="%s"' % PAGE_2019 in xmlstr
|
||||
assert 'Metadata' in xmlstr
|
||||
|
||||
if __name__ == '__main__':
|
||||
main([__file__])
|
||||
|
|
Loading…
Add table
Add a link
Reference in a new issue